Track4Gen: Teaching Video Diffusion Models to Track Points Improves Video Generation
Hyeonho Jeong, Chun-Hao Paul Huang, Jong Chul Ye, Niloy Mitra, Duygu Ceylan
2024-12-12
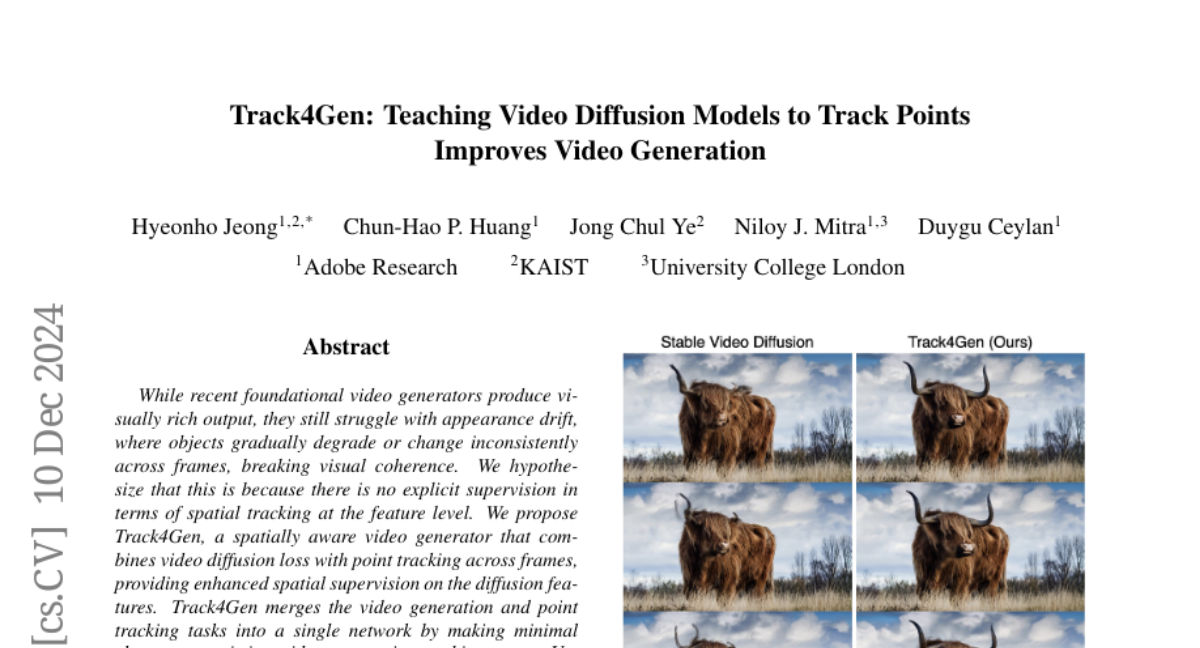
Summary
This paper talks about Track4Gen, a new method that helps improve how AI generates videos by teaching it to track specific points in the video, which makes the output more consistent and visually coherent.
What's the problem?
When AI generates videos, it often suffers from a problem called appearance drift, where objects in the video look different or change in an unnatural way from one frame to the next. This inconsistency can break the flow of the video and make it less realistic, which is a significant issue for applications like animation or virtual reality.
What's the solution?
To tackle this issue, the authors developed Track4Gen, which combines video generation with point tracking. By integrating point tracking into the video generation process, Track4Gen ensures that the model pays attention to specific points in the video and maintains their appearance across frames. This is achieved by making slight modifications to existing video generation models and using a technique called diffusion loss to enhance the tracking of these points. The result is a more stable and coherent video output.
Why it matters?
This research is important because it significantly enhances the quality of AI-generated videos. By reducing appearance drift and improving consistency, Track4Gen can lead to better visual experiences in various fields such as film production, gaming, and virtual reality. This advancement makes it easier for creators to produce high-quality content that looks natural and engaging.
Abstract
While recent foundational video generators produce visually rich output, they still struggle with appearance drift, where objects gradually degrade or change inconsistently across frames, breaking visual coherence. We hypothesize that this is because there is no explicit supervision in terms of spatial tracking at the feature level. We propose Track4Gen, a spatially aware video generator that combines video diffusion loss with point tracking across frames, providing enhanced spatial supervision on the diffusion features. Track4Gen merges the video generation and point tracking tasks into a single network by making minimal changes to existing video generation architectures. Using Stable Video Diffusion as a backbone, Track4Gen demonstrates that it is possible to unify video generation and point tracking, which are typically handled as separate tasks. Our extensive evaluations show that Track4Gen effectively reduces appearance drift, resulting in temporally stable and visually coherent video generation. Project page: hyeonho99.github.io/track4gen