TrackGo: A Flexible and Efficient Method for Controllable Video Generation
Haitao Zhou, Chuang Wang, Rui Nie, Jinxiao Lin, Dongdong Yu, Qian Yu, Changhu Wang
2024-08-22
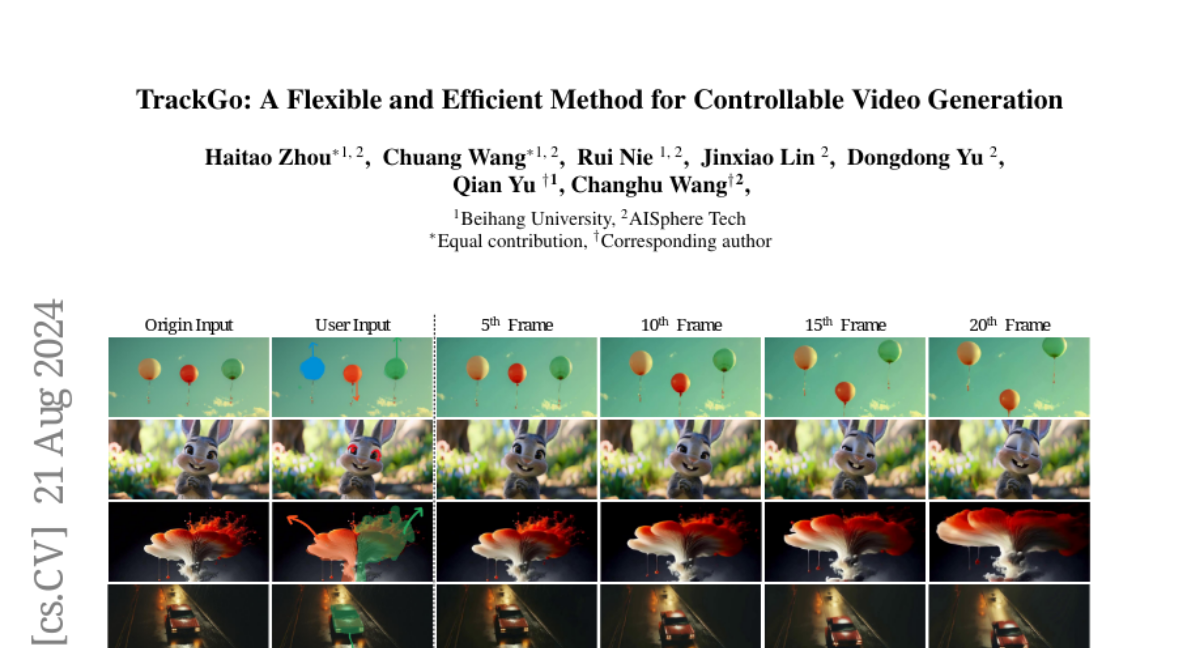
Summary
This paper presents TrackGo, a new method for generating videos that allows users to have precise control over video content using free-form masks and arrows.
What's the problem?
Creating videos with specific details, like moving objects or backgrounds, can be challenging. Existing methods often lack the flexibility needed to manipulate these elements accurately, making it hard to achieve the desired results in complex scenarios.
What's the solution?
TrackGo introduces a system that uses free-form masks and arrows to help users control video generation more effectively. It includes a component called the TrackAdapter, which is a lightweight tool that integrates with existing video models to enhance their ability to focus on relevant motion areas in videos. This approach allows for better manipulation of video content while maintaining high quality.
Why it matters?
This research is important because it improves how we create and edit videos, making it easier for users to generate content that meets their specific needs. With better control over video elements, applications in fields like filmmaking, animation, and advertising can become more efficient and creative.
Abstract
Recent years have seen substantial progress in diffusion-based controllable video generation. However, achieving precise control in complex scenarios, including fine-grained object parts, sophisticated motion trajectories, and coherent background movement, remains a challenge. In this paper, we introduce TrackGo, a novel approach that leverages free-form masks and arrows for conditional video generation. This method offers users with a flexible and precise mechanism for manipulating video content. We also propose the TrackAdapter for control implementation, an efficient and lightweight adapter designed to be seamlessly integrated into the temporal self-attention layers of a pretrained video generation model. This design leverages our observation that the attention map of these layers can accurately activate regions corresponding to motion in videos. Our experimental results demonstrate that our new approach, enhanced by the TrackAdapter, achieves state-of-the-art performance on key metrics such as FVD, FID, and ObjMC scores. The project page of TrackGo can be found at: https://zhtjtcz.github.io/TrackGo-Page/