Training-free Camera Control for Video Generation
Chen Hou, Guoqiang Wei, Yan Zeng, Zhibo Chen
2024-06-17
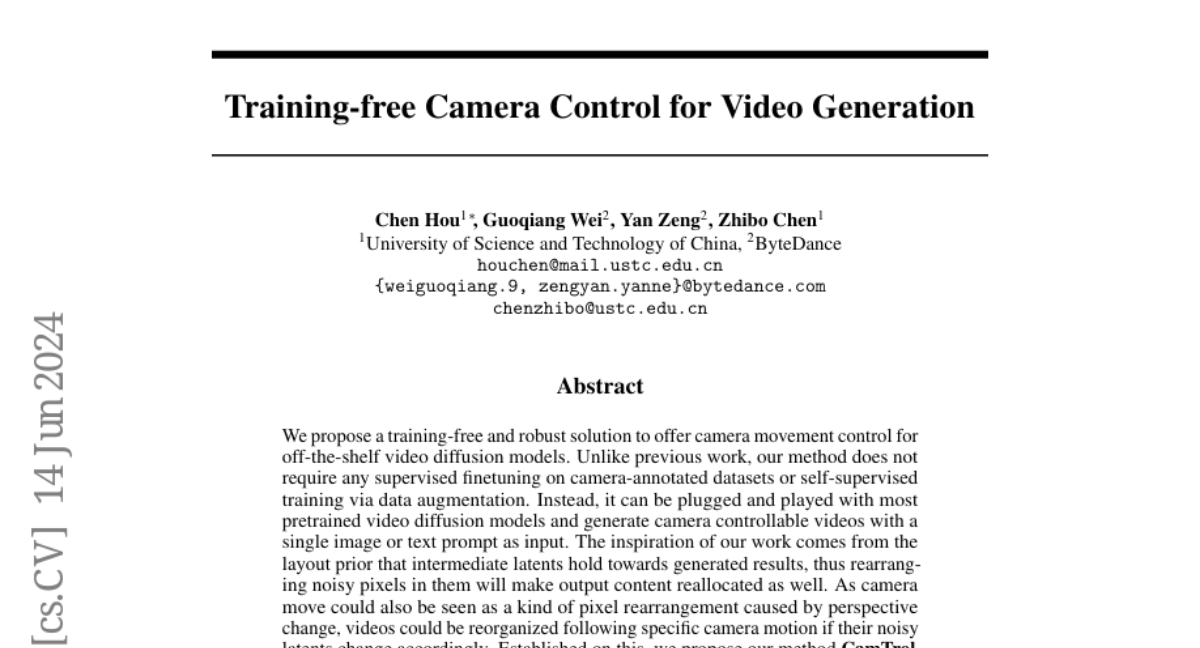
Summary
This paper introduces a new method called CamTrol, which allows users to control camera movements in video generation without needing any prior training. It works with existing video models and can create videos based on simple inputs like images or text prompts.
What's the problem?
Creating videos with the right camera angles and movements usually requires a lot of skill and experience. Traditional methods often need extensive training on specific datasets to understand how to control the camera effectively, making it difficult for average users to create high-quality videos. This can be a barrier for those who want to produce videos without having technical expertise in video production.
What's the solution?
To solve this problem, the authors developed CamTrol, which can be easily integrated into most pre-trained video generation models. Instead of requiring complex training, CamTrol uses a two-step process: first, it models how the camera should move in a 3D space based on the input; then it generates the video by rearranging pixels according to the desired camera movements. This allows users to create videos that match their vision without needing detailed knowledge about camera operation.
Why it matters?
This research is important because it makes video creation more accessible to everyone, not just experts. By simplifying the process of controlling camera movements, CamTrol enables more people to express their creativity through video. This could lead to a wider variety of content being produced and encourage experimentation in video storytelling.
Abstract
We propose a training-free and robust solution to offer camera movement control for off-the-shelf video diffusion models. Unlike previous work, our method does not require any supervised finetuning on camera-annotated datasets or self-supervised training via data augmentation. Instead, it can be plugged and played with most pretrained video diffusion models and generate camera controllable videos with a single image or text prompt as input. The inspiration of our work comes from the layout prior that intermediate latents hold towards generated results, thus rearranging noisy pixels in them will make output content reallocated as well. As camera move could also be seen as a kind of pixel rearrangement caused by perspective change, videos could be reorganized following specific camera motion if their noisy latents change accordingly. Established on this, we propose our method CamTrol, which enables robust camera control for video diffusion models. It is achieved by a two-stage process. First, we model image layout rearrangement through explicit camera movement in 3D point cloud space. Second, we generate videos with camera motion using layout prior of noisy latents formed by a series of rearranged images. Extensive experiments have demonstrated the robustness our method holds in controlling camera motion of generated videos. Furthermore, we show that our method can produce impressive results in generating 3D rotation videos with dynamic content. Project page at https://lifedecoder.github.io/CamTrol/.