Training Language Models on the Knowledge Graph: Insights on Hallucinations and Their Detectability
Jiri Hron, Laura Culp, Gamaleldin Elsayed, Rosanne Liu, Ben Adlam, Maxwell Bileschi, Bernd Bohnet, JD Co-Reyes, Noah Fiedel, C. Daniel Freeman, Izzeddin Gur, Kathleen Kenealy, Jaehoon Lee, Peter J. Liu, Gaurav Mishra, Igor Mordatch, Azade Nova, Roman Novak, Aaron Parisi, Jeffrey Pennington, Alex Rizkowsky, Isabelle Simpson
2024-08-16
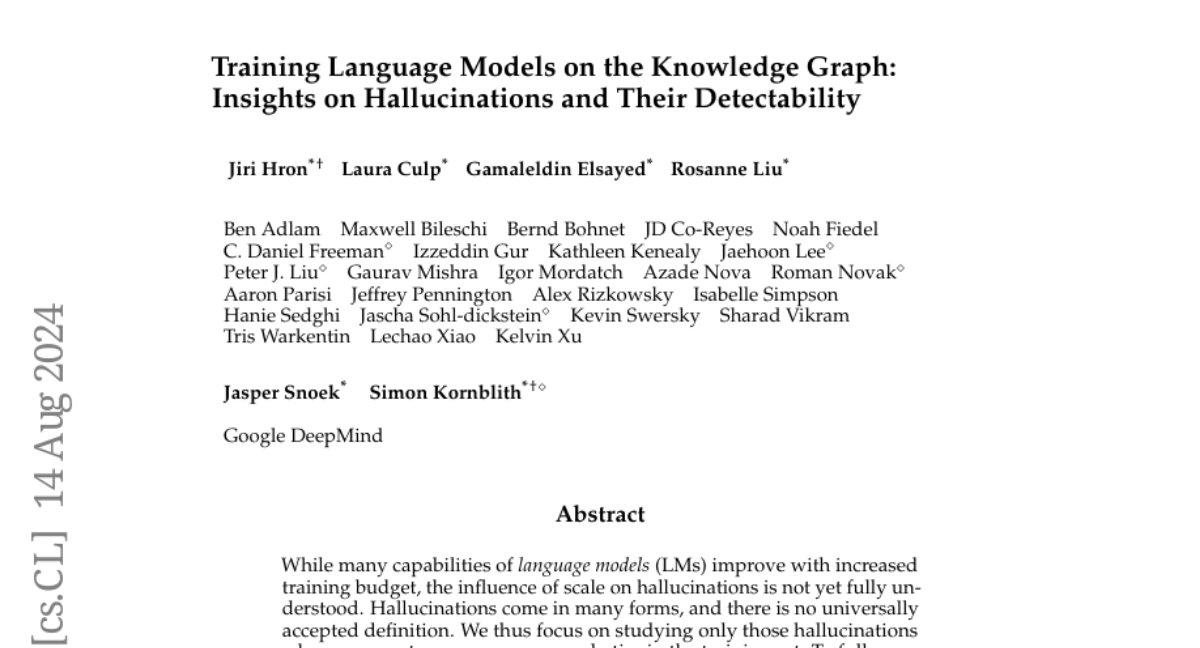
Summary
This paper explores how training language models (LMs) with a knowledge graph can help reduce errors known as hallucinations, where the model generates incorrect information that sounds plausible.
What's the problem?
Language models often make mistakes by producing information that isn't accurate or doesn't exist, which is referred to as hallucinations. Understanding why these errors happen and how to detect them is crucial, especially since there isn't a clear definition of what counts as a hallucination. Additionally, larger models tend to hallucinate less, but figuring out how to achieve this efficiently is challenging.
What's the solution?
The authors created a dataset based on a knowledge graph to train LMs more effectively. They found that larger models trained on this dataset made fewer hallucinations. They also studied how well different sizes of detection systems could identify these errors. Their research showed that while bigger models are better at reducing hallucinations, it becomes harder to detect these errors as the model size increases.
Why it matters?
This research matters because it helps improve the reliability of language models, making them more trustworthy for applications like chatbots and information retrieval. By understanding and reducing hallucinations, we can create better AI systems that provide accurate and useful information.
Abstract
While many capabilities of language models (LMs) improve with increased training budget, the influence of scale on hallucinations is not yet fully understood. Hallucinations come in many forms, and there is no universally accepted definition. We thus focus on studying only those hallucinations where a correct answer appears verbatim in the training set. To fully control the training data content, we construct a knowledge graph (KG)-based dataset, and use it to train a set of increasingly large LMs. We find that for a fixed dataset, larger and longer-trained LMs hallucinate less. However, hallucinating on leq5% of the training data requires an order of magnitude larger model, and thus an order of magnitude more compute, than Hoffmann et al. (2022) reported was optimal. Given this costliness, we study how hallucination detectors depend on scale. While we see detector size improves performance on fixed LM's outputs, we find an inverse relationship between the scale of the LM and the detectability of its hallucinations.