Transfusion: Predict the Next Token and Diffuse Images with One Multi-Modal Model
Chunting Zhou, Lili Yu, Arun Babu, Kushal Tirumala, Michihiro Yasunaga, Leonid Shamis, Jacob Kahn, Xuezhe Ma, Luke Zettlemoyer, Omer Levy
2024-08-21
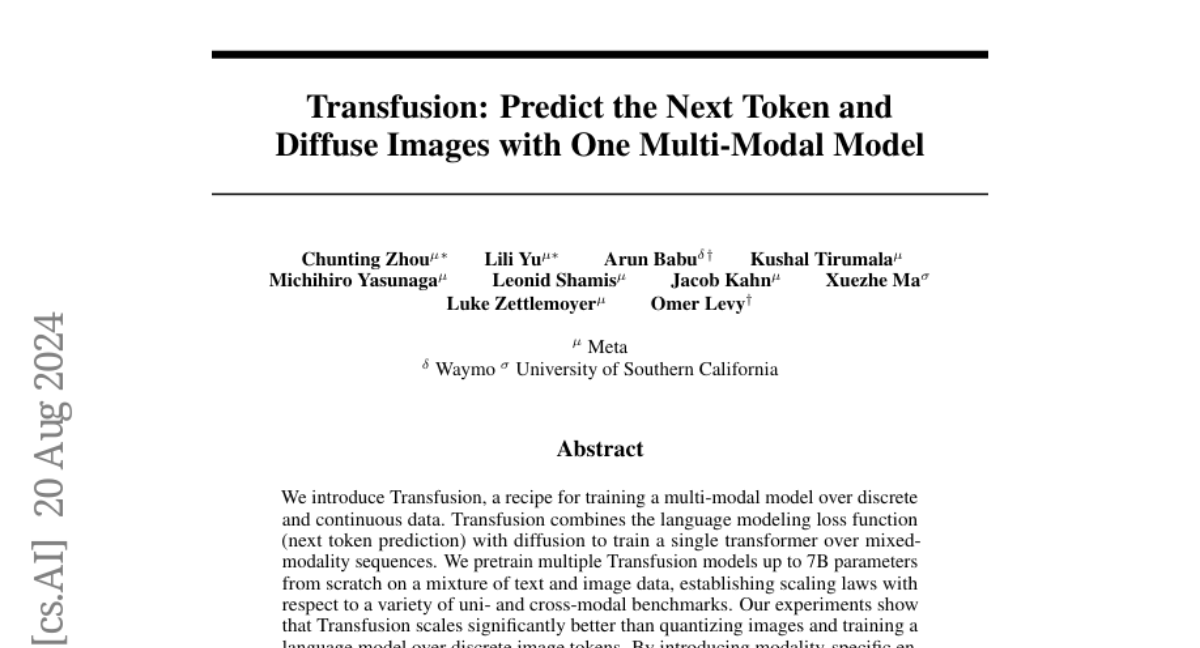
Summary
This paper introduces Transfusion, a new method for training a multi-modal model that can generate both images and text by predicting the next token and using diffusion techniques.
What's the problem?
Generating high-quality images and text together is challenging because existing models often require separate training processes for each type of data. This can lead to inefficiencies and difficulties in combining these different data types effectively.
What's the solution?
Transfusion combines the tasks of predicting the next word in a sentence (language modeling) with diffusion, which is a method used to generate images. By training a single model on both text and image data, it can learn to generate content that is coherent in both formats. The model uses special layers to handle different types of data and can compress images into smaller pieces, making it more efficient.
Why it matters?
This research is significant because it simplifies the process of creating models that can handle multiple types of data at once. By improving how we generate images and text together, Transfusion can enhance applications in areas like storytelling, video game design, and virtual reality, where combining visuals and narratives is essential.
Abstract
We introduce Transfusion, a recipe for training a multi-modal model over discrete and continuous data. Transfusion combines the language modeling loss function (next token prediction) with diffusion to train a single transformer over mixed-modality sequences. We pretrain multiple Transfusion models up to 7B parameters from scratch on a mixture of text and image data, establishing scaling laws with respect to a variety of uni- and cross-modal benchmarks. Our experiments show that Transfusion scales significantly better than quantizing images and training a language model over discrete image tokens. By introducing modality-specific encoding and decoding layers, we can further improve the performance of Transfusion models, and even compress each image to just 16 patches. We further demonstrate that scaling our Transfusion recipe to 7B parameters and 2T multi-modal tokens produces a model that can generate images and text on a par with similar scale diffusion models and language models, reaping the benefits of both worlds.