TVG: A Training-free Transition Video Generation Method with Diffusion Models
Rui Zhang, Yaosen Chen, Yuegen Liu, Wei Wang, Xuming Wen, Hongxia Wang
2024-08-27
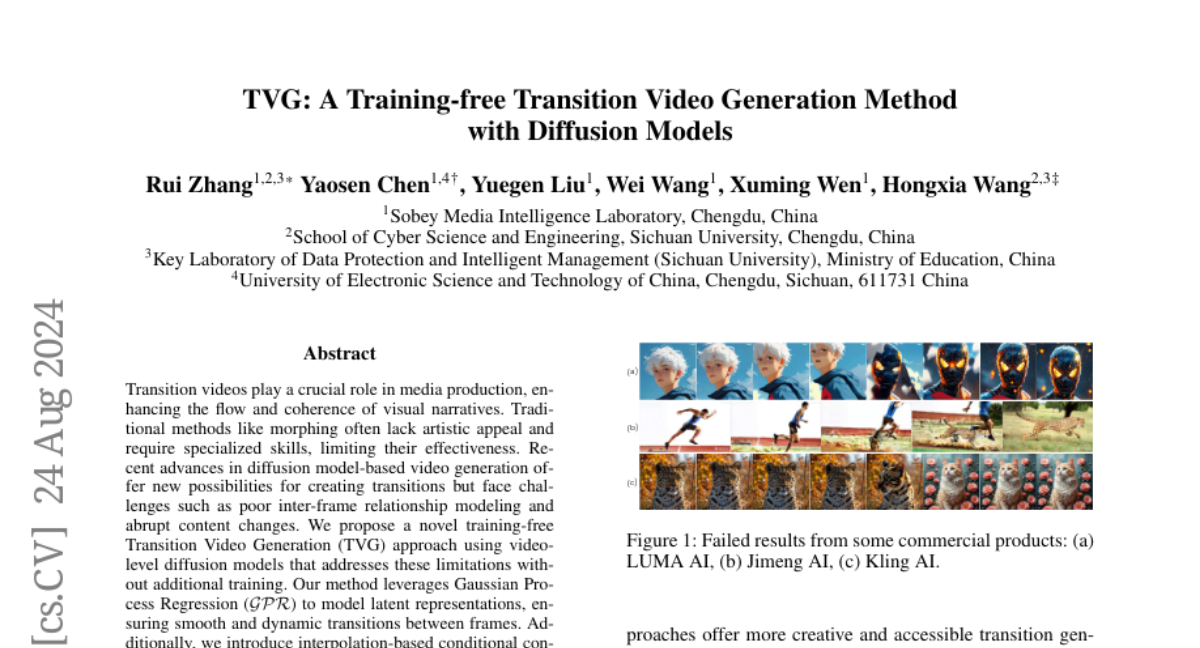
Summary
This paper discusses TVG, a new method for creating transition videos without needing extensive training, using advanced diffusion models to ensure smooth and artistic transitions between frames.
What's the problem?
Creating transition videos, which help improve the flow of visual stories, can be difficult with traditional methods like morphing. These methods often require special skills and can lack creativity, making them less effective for media production. Additionally, existing video generation techniques struggle with maintaining smooth connections between different frames.
What's the solution?
The authors propose a training-free approach called Transition Video Generation (TVG) that uses video-level diffusion models. This method breaks down the video creation process into simpler tasks, ensuring smoother transitions by modeling how frames relate to each other. They also introduce new techniques like interpolation-based controls and a special architecture to improve the reliability of these transitions. Their experiments show that TVG can produce high-quality transition videos effectively.
Why it matters?
This research is important because it simplifies the process of making visually appealing transition videos, making it accessible to more creators in media production. By improving how transitions are generated, TVG can enhance storytelling in films, animations, and other visual media.
Abstract
Transition videos play a crucial role in media production, enhancing the flow and coherence of visual narratives. Traditional methods like morphing often lack artistic appeal and require specialized skills, limiting their effectiveness. Recent advances in diffusion model-based video generation offer new possibilities for creating transitions but face challenges such as poor inter-frame relationship modeling and abrupt content changes. We propose a novel training-free Transition Video Generation (TVG) approach using video-level diffusion models that addresses these limitations without additional training. Our method leverages Gaussian Process Regression (GPR) to model latent representations, ensuring smooth and dynamic transitions between frames. Additionally, we introduce interpolation-based conditional controls and a Frequency-aware Bidirectional Fusion (FBiF) architecture to enhance temporal control and transition reliability. Evaluations of benchmark datasets and custom image pairs demonstrate the effectiveness of our approach in generating high-quality smooth transition videos. The code are provided in https://sobeymil.github.io/tvg.com.