TweedieMix: Improving Multi-Concept Fusion for Diffusion-based Image/Video Generation
Gihyun Kwon, Jong Chul Ye
2024-10-10
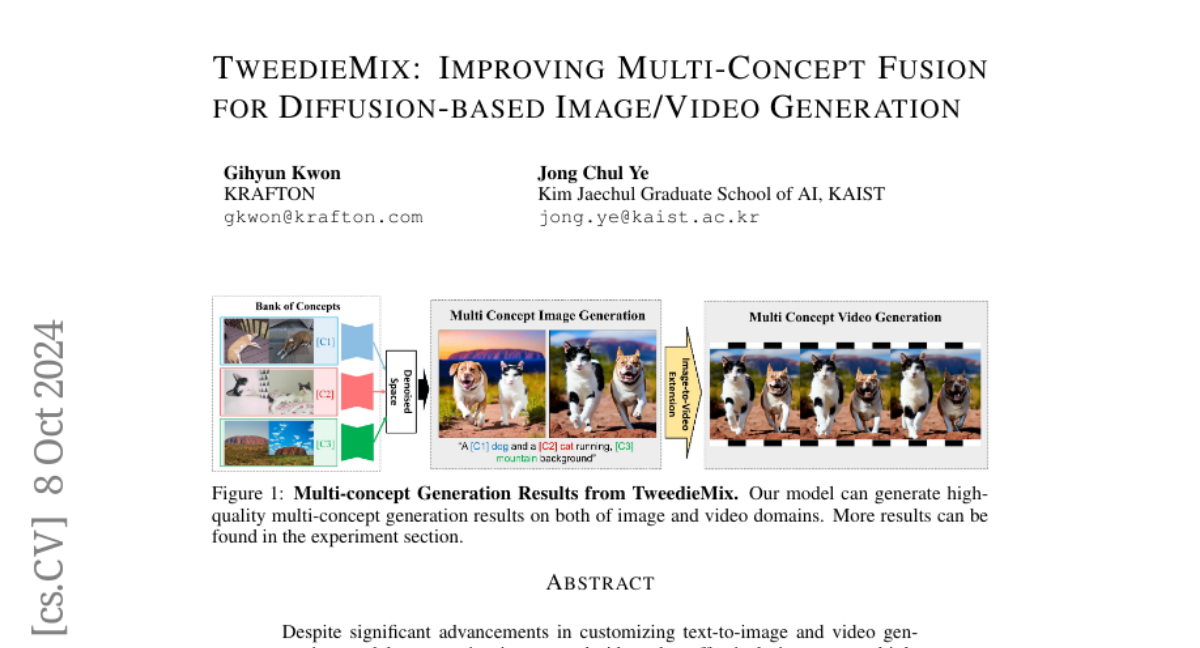
Summary
This paper introduces TweedieMix, a new method for generating images and videos that effectively combine multiple personalized concepts using diffusion models.
What's the problem?
Creating images and videos that seamlessly integrate several different ideas or themes can be very challenging. Existing methods often struggle to blend these concepts well, leading to lower quality outputs and difficulties in maintaining visual consistency.
What's the solution?
TweedieMix addresses this problem by using a two-stage sampling process. In the first stage, it focuses on ensuring that all desired objects are included in the generated images. In the second stage, it blends the appearances of these concepts using a mathematical approach called Tweedie's formula. This allows for better integration of multiple concepts, resulting in higher quality images and videos. The method can also be applied to video generation, making it versatile.
Why it matters?
This research is significant because it enhances the ability of AI to create complex visual content that reflects user-defined ideas. By improving how multiple concepts are fused together, TweedieMix can benefit content creators in fields like entertainment, advertising, and education, enabling them to produce richer and more personalized visual stories.
Abstract
Despite significant advancements in customizing text-to-image and video generation models, generating images and videos that effectively integrate multiple personalized concepts remains a challenging task. To address this, we present TweedieMix, a novel method for composing customized diffusion models during the inference phase. By analyzing the properties of reverse diffusion sampling, our approach divides the sampling process into two stages. During the initial steps, we apply a multiple object-aware sampling technique to ensure the inclusion of the desired target objects. In the later steps, we blend the appearances of the custom concepts in the de-noised image space using Tweedie's formula. Our results demonstrate that TweedieMix can generate multiple personalized concepts with higher fidelity than existing methods. Moreover, our framework can be effortlessly extended to image-to-video diffusion models, enabling the generation of videos that feature multiple personalized concepts. Results and source code are in our anonymous project page.