Two Giraffes in a Dirt Field: Using Game Play to Investigate Situation Modelling in Large Multimodal Models
Sherzod Hakimov, Yerkezhan Abdullayeva, Kushal Koshti, Antonia Schmidt, Yan Weiser, Anne Beyer, David Schlangen
2024-06-24
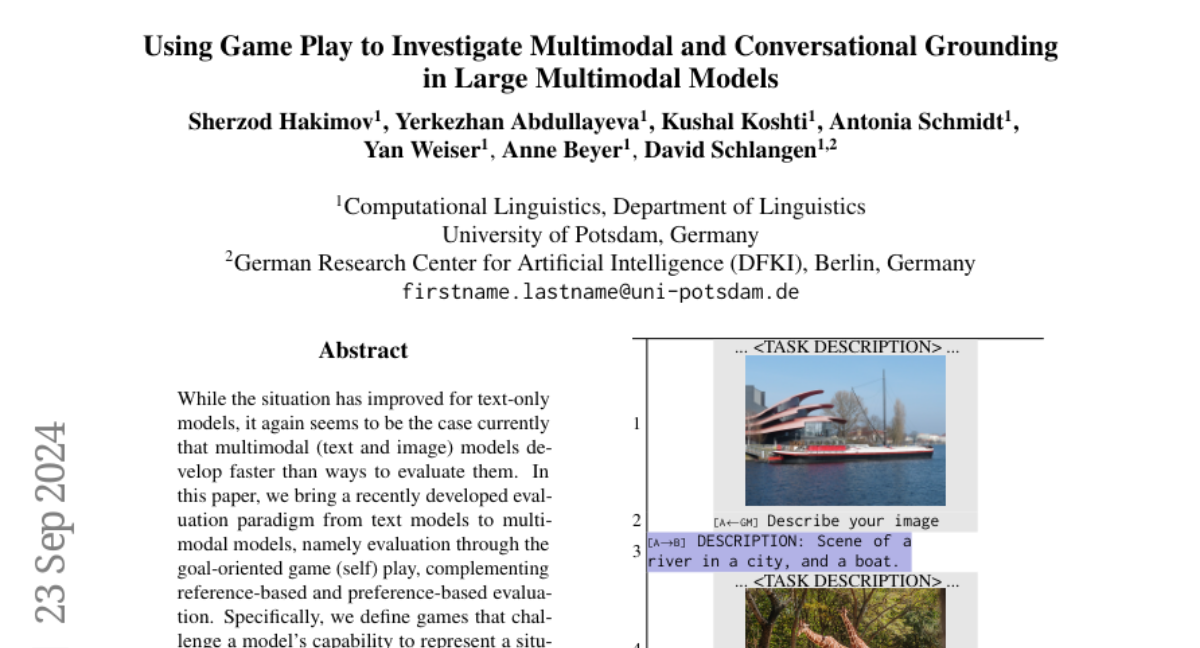
Summary
This paper discusses how to evaluate large multimodal models (which use both text and images) by using games that test their ability to understand and represent situations based on visual information.
What's the problem?
While there have been improvements in evaluating text-only models, the evaluation methods for multimodal models are not keeping pace with their development. This makes it difficult to assess how well these models can understand and process complex information that combines both text and images. Without effective evaluation methods, it’s hard to know how good these models really are.
What's the solution?
The authors propose a new evaluation method that uses goal-oriented games to challenge multimodal models. These games require the models to represent situations based on visual inputs and communicate about them through dialogue. The researchers found that the largest closed models (those that are not open-source) performed well in these games, while the best open-weight models had more difficulty. They also noted that the strong performance of the largest models is largely due to their advanced captioning abilities, which help them understand and describe what they see in images.
Why it matters?
This research is important because it provides a new way to evaluate multimodal models, ensuring that they can effectively understand and interact with complex visual information. By developing better evaluation methods, we can improve these AI systems, making them more useful for applications like virtual assistants, gaming, and content creation where understanding both text and images is crucial.
Abstract
While the situation has improved for text-only models, it again seems to be the case currently that multimodal (text and image) models develop faster than ways to evaluate them. In this paper, we bring a recently developed evaluation paradigm from text models to multimodal models, namely evaluation through the goal-oriented game (self) play, complementing reference-based and preference-based evaluation. Specifically, we define games that challenge a model's capability to represent a situation from visual information and align such representations through dialogue. We find that the largest closed models perform rather well on the games that we define, while even the best open-weight models struggle with them. On further analysis, we find that the exceptional deep captioning capabilities of the largest models drive some of the performance. There is still room to grow for both kinds of models, ensuring the continued relevance of the benchmark.