UCFE: A User-Centric Financial Expertise Benchmark for Large Language Models
Yuzhe Yang, Yifei Zhang, Yan Hu, Yilin Guo, Ruoli Gan, Yueru He, Mingcong Lei, Xiao Zhang, Haining Wang, Qianqian Xie, Jimin Huang, Honghai Yu, Benyou Wang
2024-10-21
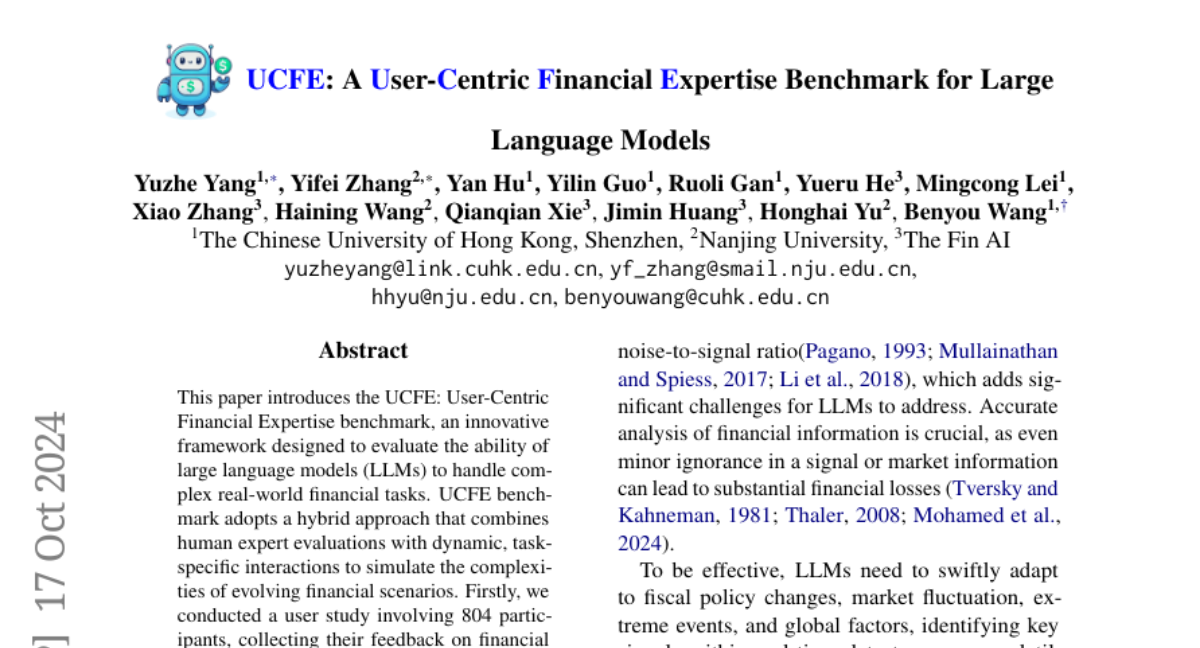
Summary
This paper introduces the UCFE benchmark, which is designed to evaluate how well large language models (LLMs) can perform complex financial tasks by using feedback from real users.
What's the problem?
Many existing benchmarks for evaluating LLMs do not effectively measure their ability to handle real-world financial scenarios. This is a problem because financial tasks can be very complex and require a deep understanding of user needs and preferences. Without proper evaluation methods, it’s hard to know how well these models will perform in actual financial situations.
What's the solution?
To address this issue, the authors developed the UCFE (User-Centric Financial Expertise) benchmark. They conducted a study with 804 participants to gather feedback on various financial tasks. Using this feedback, they created a dataset that includes a wide range of user intents and interactions. This dataset was then used to benchmark 12 different LLM services, comparing their performance with human preferences. The results showed a strong correlation between the benchmark scores and what users actually preferred, confirming that UCFE is an effective way to assess LLMs in the financial sector.
Why it matters?
This research is important because it helps improve how AI models are evaluated in the finance industry. By focusing on user-centric evaluations, the UCFE benchmark ensures that LLMs are not only accurate but also aligned with what users need and expect. This could lead to better AI tools for managing finances, making investments, and providing financial advice, ultimately benefiting users in real-world applications.
Abstract
This paper introduces the UCFE: User-Centric Financial Expertise benchmark, an innovative framework designed to evaluate the ability of large language models (LLMs) to handle complex real-world financial tasks. UCFE benchmark adopts a hybrid approach that combines human expert evaluations with dynamic, task-specific interactions to simulate the complexities of evolving financial scenarios. Firstly, we conducted a user study involving 804 participants, collecting their feedback on financial tasks. Secondly, based on this feedback, we created our dataset that encompasses a wide range of user intents and interactions. This dataset serves as the foundation for benchmarking 12 LLM services using the LLM-as-Judge methodology. Our results show a significant alignment between benchmark scores and human preferences, with a Pearson correlation coefficient of 0.78, confirming the effectiveness of the UCFE dataset and our evaluation approach. UCFE benchmark not only reveals the potential of LLMs in the financial sector but also provides a robust framework for assessing their performance and user satisfaction.The benchmark dataset and evaluation code are available.