UIP2P: Unsupervised Instruction-based Image Editing via Cycle Edit Consistency
Enis Simsar, Alessio Tonioni, Yongqin Xian, Thomas Hofmann, Federico Tombari
2024-12-20
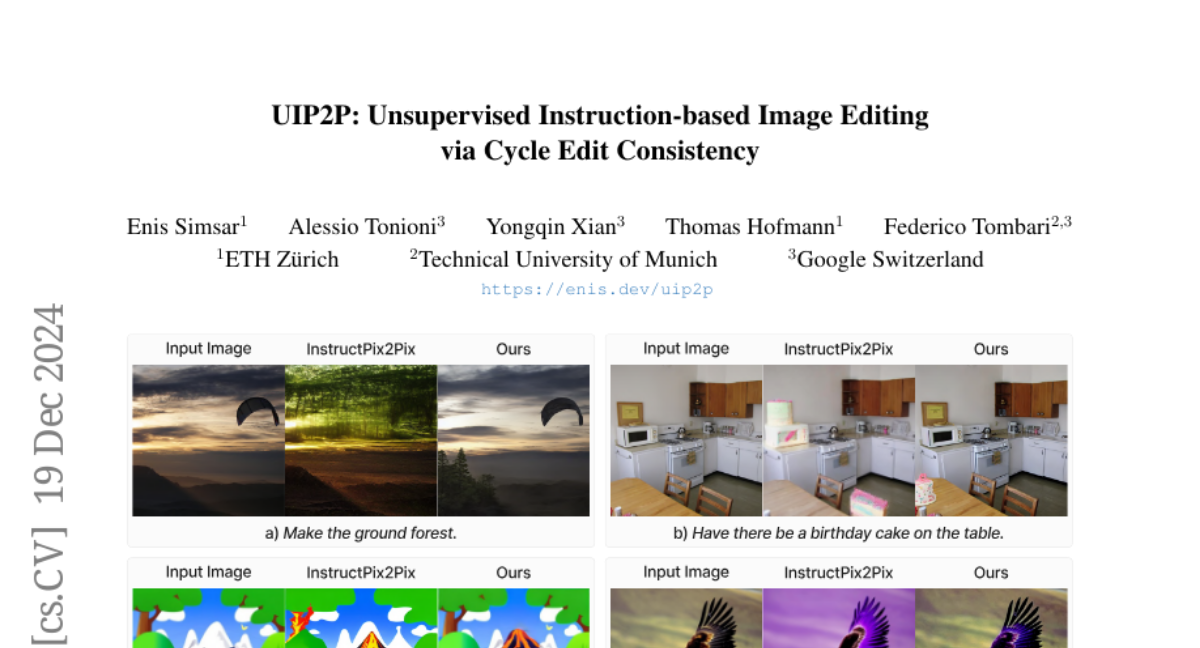
Summary
This paper talks about UIP2P, a new method for editing images based on instructions without needing pre-edited images for training. It introduces a technique called Cycle Edit Consistency (CEC) to ensure that edits can be reversed and are consistent.
What's the problem?
Traditional image editing methods require datasets that include original images, edited images, and instructions on how to edit them. This can introduce biases and limit the model's ability to learn effectively, making it hard for the model to generalize to new types of edits.
What's the solution?
UIP2P addresses this issue by using an unsupervised approach that does not rely on ground-truth edited images. Instead, it uses CEC to apply edits in both forward and reverse directions in one training step. This means that when an image is edited, the model also learns how to revert back to the original image, ensuring that the edits are coherent and accurate. The method allows training on datasets with real image-caption pairs or image-caption-edit triplets without needing pre-existing edited images.
Why it matters?
This research is important because it enhances the flexibility and effectiveness of image editing AI. By removing the dependency on specific datasets for training, UIP2P can work with a wider range of images and instructions, making it a powerful tool for various applications in graphic design, content creation, and more.
Abstract
We propose an unsupervised model for instruction-based image editing that eliminates the need for ground-truth edited images during training. Existing supervised methods depend on datasets containing triplets of input image, edited image, and edit instruction. These are generated by either existing editing methods or human-annotations, which introduce biases and limit their generalization ability. Our method addresses these challenges by introducing a novel editing mechanism called Cycle Edit Consistency (CEC), which applies forward and backward edits in one training step and enforces consistency in image and attention spaces. This allows us to bypass the need for ground-truth edited images and unlock training for the first time on datasets comprising either real image-caption pairs or image-caption-edit triplets. We empirically show that our unsupervised technique performs better across a broader range of edits with high fidelity and precision. By eliminating the need for pre-existing datasets of triplets, reducing biases associated with supervised methods, and proposing CEC, our work represents a significant advancement in unblocking scaling of instruction-based image editing.