Unboxing Occupational Bias: Grounded Debiasing LLMs with U.S. Labor Data
Atmika Gorti, Manas Gaur, Aman Chadha
2024-08-22
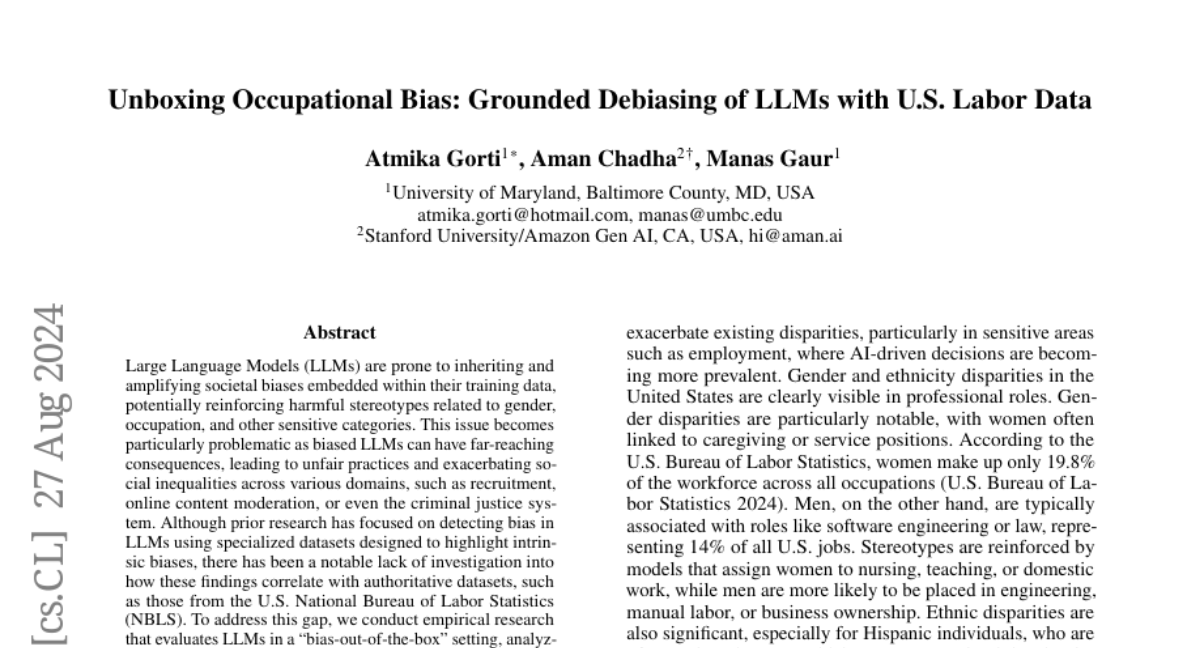
Summary
This paper discusses how to reduce biases in large language models (LLMs) by using data from the U.S. labor market to create fairer and more accurate AI systems.
What's the problem?
Large language models can pick up and amplify biases present in the data they are trained on, which can lead to unfair stereotypes about gender, jobs, and other sensitive topics. This is a serious issue because biased AI can affect important areas like hiring practices and content moderation, leading to social inequalities.
What's the solution?
The authors conducted research to see how well LLMs perform when compared to reliable labor statistics from the U.S. They developed a method that incorporates this labor data directly into the training process of the models, helping to reduce bias without needing additional datasets. Their approach was tested on seven different LLMs and showed significant improvements in reducing bias while maintaining accuracy.
Why it matters?
This research is important because it addresses a critical issue in AI development—bias. By creating methods to make LLMs fairer, this work can help ensure that AI technologies are used responsibly and equitably, ultimately contributing to a more just society.
Abstract
Large Language Models (LLMs) are prone to inheriting and amplifying societal biases embedded within their training data, potentially reinforcing harmful stereotypes related to gender, occupation, and other sensitive categories. This issue becomes particularly problematic as biased LLMs can have far-reaching consequences, leading to unfair practices and exacerbating social inequalities across various domains, such as recruitment, online content moderation, or even the criminal justice system. Although prior research has focused on detecting bias in LLMs using specialized datasets designed to highlight intrinsic biases, there has been a notable lack of investigation into how these findings correlate with authoritative datasets, such as those from the U.S. National Bureau of Labor Statistics (NBLS). To address this gap, we conduct empirical research that evaluates LLMs in a ``bias-out-of-the-box" setting, analyzing how the generated outputs compare with the distributions found in NBLS data. Furthermore, we propose a straightforward yet effective debiasing mechanism that directly incorporates NBLS instances to mitigate bias within LLMs. Our study spans seven different LLMs, including instructable, base, and mixture-of-expert models, and reveals significant levels of bias that are often overlooked by existing bias detection techniques. Importantly, our debiasing method, which does not rely on external datasets, demonstrates a substantial reduction in bias scores, highlighting the efficacy of our approach in creating fairer and more reliable LLMs.