UnCommon Objects in 3D
Xingchen Liu, Piyush Tayal, Jianyuan Wang, Jesus Zarzar, Tom Monnier, Konstantinos Tertikas, Jiali Duan, Antoine Toisoul, Jason Y. Zhang, Natalia Neverova, Andrea Vedaldi, Roman Shapovalov, David Novotny
2025-01-14
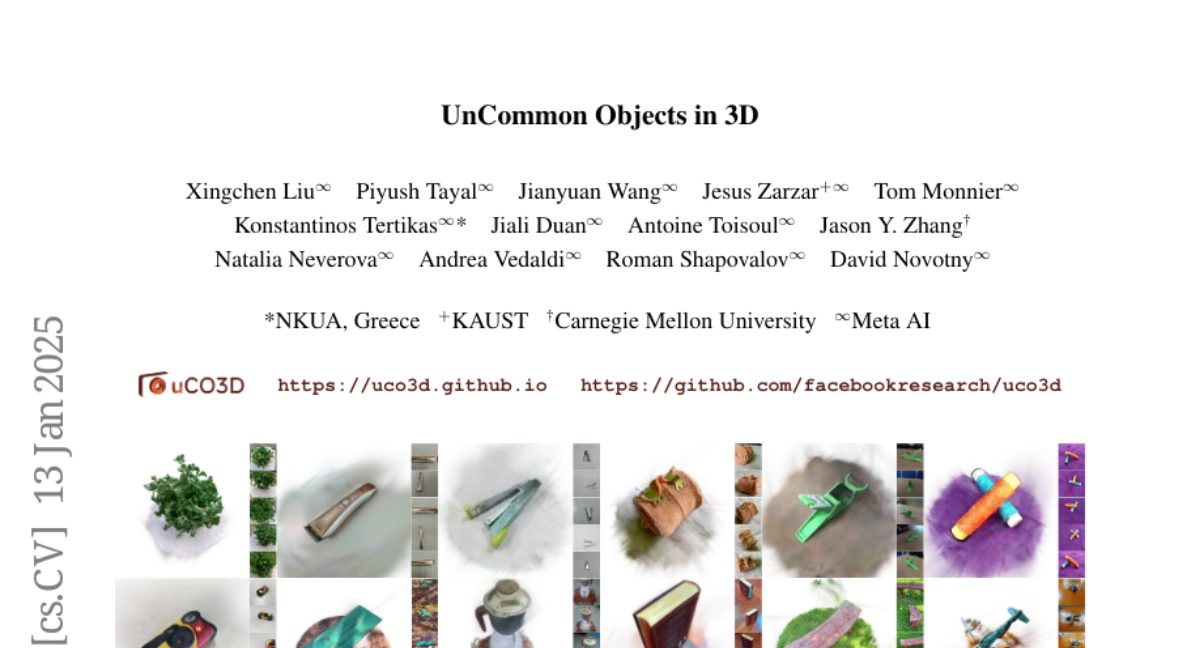
Summary
This paper talks about a new dataset called Uncommon Objects in 3D (uCO3D), which is like a huge digital library of videos showing everyday objects from all angles. It's designed to help computers better understand and create 3D images of objects.
What's the problem?
Current datasets used to teach computers about 3D objects are limited. They don't have enough variety in the types of objects they show, and the quality of the information isn't always great. This makes it hard for AI to learn how to recognize and work with 3D objects in the real world.
What's the solution?
The researchers created uCO3D, which includes high-quality videos of over 1,000 different types of objects, each filmed from every angle. They carefully checked all the videos and added detailed information about each object's shape, depth, and position. They also included descriptions of each object and used a special technique called 3D Gaussian Splat to create really accurate 3D models. When they tested AI systems trained on uCO3D, these systems performed better than those trained on older datasets.
Why it matters?
This matters because it could help make AI much better at understanding the 3D world around us. Better 3D understanding could lead to improvements in things like virtual reality, robots that can interact with objects more naturally, and even self-driving cars that can better recognize objects on the road. It could also help create more realistic computer graphics for movies and video games. By making this dataset public, the researchers are helping other scientists and developers create smarter, more capable AI systems that can work with 3D objects.
Abstract
We introduce Uncommon Objects in 3D (uCO3D), a new object-centric dataset for 3D deep learning and 3D generative AI. uCO3D is the largest publicly-available collection of high-resolution videos of objects with 3D annotations that ensures full-360^{circ} coverage. uCO3D is significantly more diverse than MVImgNet and CO3Dv2, covering more than 1,000 object categories. It is also of higher quality, due to extensive quality checks of both the collected videos and the 3D annotations. Similar to analogous datasets, uCO3D contains annotations for 3D camera poses, depth maps and sparse point clouds. In addition, each object is equipped with a caption and a 3D Gaussian Splat reconstruction. We train several large 3D models on MVImgNet, CO3Dv2, and uCO3D and obtain superior results using the latter, showing that uCO3D is better for learning applications.