Understanding Reference Policies in Direct Preference Optimization
Yixin Liu, Pengfei Liu, Arman Cohan
2024-07-19
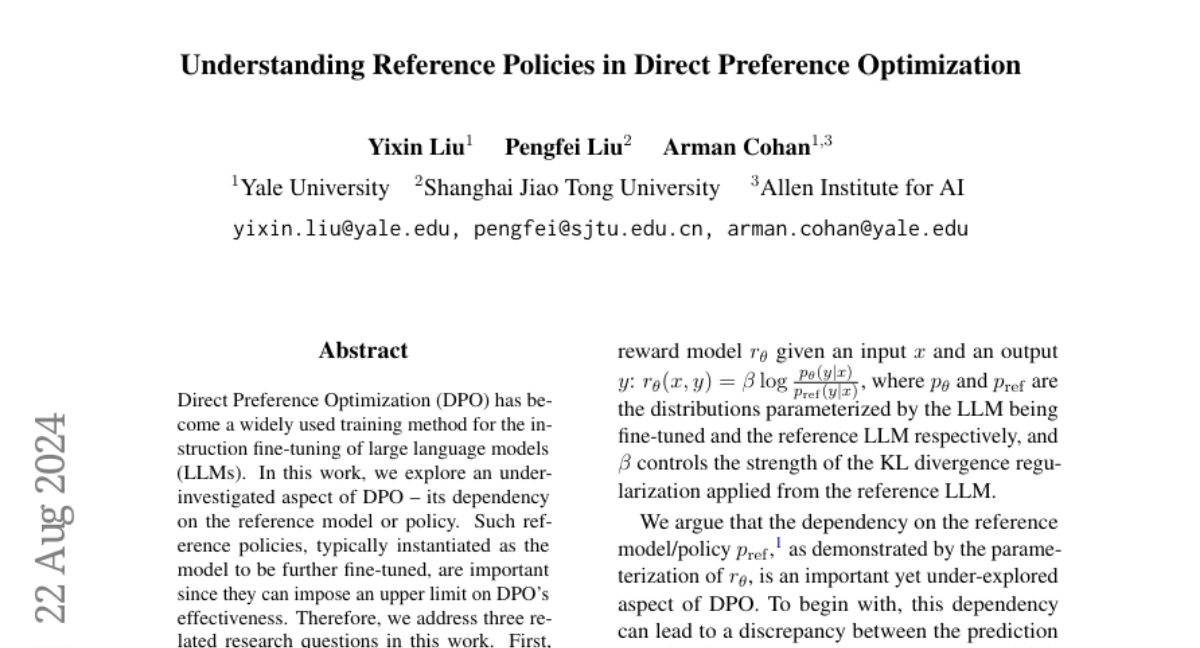
Summary
This paper explores how Direct Preference Optimization (DPO), a method used to improve large language models (LLMs), relies on reference policies. It examines how these reference models can affect the effectiveness of DPO and suggests best practices for their use.
What's the problem?
DPO is a training technique for fine-tuning LLMs, but it can be limited by the reference model used during training. If the reference model is not strong enough or not similar enough to the model being improved, it can hinder the performance of DPO, making it essential to understand how to choose and use these reference policies effectively.
What's the solution?
The authors investigated three main questions: how strong the constraints should be when using a reference model, whether reference models are necessary for effective training, and if stronger reference models improve performance. They found that DPO is sensitive to the strength of constraints and that using a stronger reference model can help, but only if it closely matches the model being fine-tuned. This research provides insights into how to optimize DPO training by carefully selecting and using reference policies.
Why it matters?
Understanding how reference policies impact DPO is crucial for improving the training of LLMs. This research can lead to better-performing AI models, which are important for applications in various fields such as education, customer service, and content creation. By identifying best practices, this work paves the way for future advancements in AI training methods.
Abstract
Direct Preference Optimization (DPO) has become a widely used training method for the instruction fine-tuning of large language models (LLMs). In this work, we explore an under-investigated aspect of DPO - its dependency on the reference model or policy. Such reference policies, typically instantiated as the model to be further fine-tuned, are important since they can impose an upper limit on DPO's effectiveness. Therefore, we address three related research questions in this work. First, we explore the optimal strength of the KL-divergence constraint in DPO, which penalizes deviations from the reference policy, and find that DPO is sensitive to this strength. Next, we examine the necessity of reference policies for instruction fine-tuning by providing both theoretical and empirical comparisons between DPO and related learning objectives, demonstrating DPO's superiority. Additionally, we investigate whether DPO benefits from stronger reference policies, finding that a stronger reference policy can lead to improved performance, but only when it is similar to the model being fine-tuned. Our findings highlight the confounding role of reference policies in DPO and offer insights for best practices, while also identifying open research questions for future studies.