Understanding Retrieval Robustness for Retrieval-Augmented Image Captioning
Wenyan Li, Jiaang Li, Rita Ramos, Raphael Tang, Desmond Elliott
2024-07-15
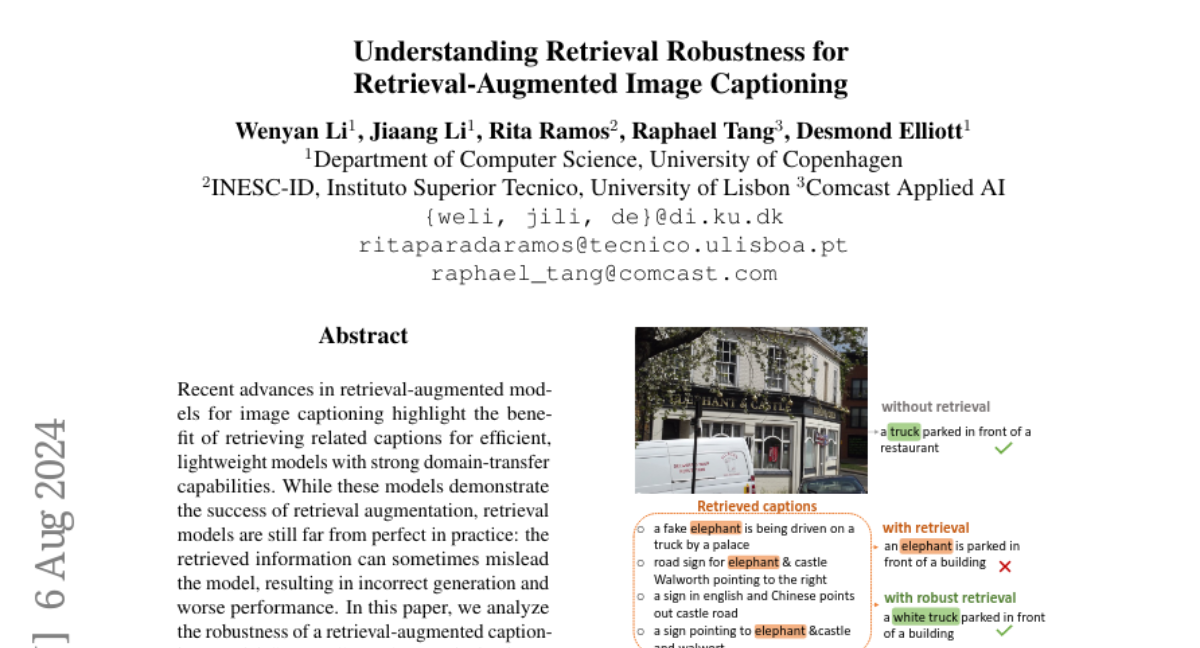
Summary
This paper discusses a new approach to improving image captioning by analyzing how well models retrieve and use information from related captions, focusing on the SmallCap model.
What's the problem?
When models generate captions for images, they often rely on retrieving similar captions from a database. However, if the retrieved information is not accurate or relevant, it can lead to incorrect captions. This problem is made worse because many existing methods do not adequately evaluate how well these retrieval systems work across different tasks, leading to inconsistent results.
What's the solution?
The researchers conducted a detailed analysis of the SmallCap model to understand its performance when using retrieved captions. They discovered that the model tends to focus on common words found in most retrieved captions, which can lead to copying those words instead of generating unique responses. To improve this, they proposed training the model with a more diverse set of retrieved captions, reducing the likelihood that it will rely too heavily on frequently used tokens. This change helped enhance the model's performance in both familiar and new contexts.
Why it matters?
This research is important because it helps improve how AI systems generate captions for images, making them more accurate and relevant. By understanding and addressing the weaknesses in retrieval-augmented captioning models, developers can create better tools for applications like automated image descriptions, which can be beneficial in fields such as education, accessibility, and content creation.
Abstract
Recent advances in retrieval-augmented models for image captioning highlight the benefit of retrieving related captions for efficient, lightweight models with strong domain-transfer capabilities. While these models demonstrate the success of retrieval augmentation, retrieval models are still far from perfect in practice: the retrieved information can sometimes mislead the model, resulting in incorrect generation and worse performance. In this paper, we analyze the robustness of a retrieval-augmented captioning model SmallCap. Our analysis shows that the model is sensitive to tokens that appear in the majority of the retrieved captions, and the input attribution shows that those tokens are likely copied into the generated output. Given these findings, we propose to train the model by sampling retrieved captions from more diverse sets. This decreases the chance that the model learns to copy majority tokens, and improves both in-domain and cross-domain performance.