Understanding Visual Feature Reliance through the Lens of Complexity
Thomas Fel, Louis Bethune, Andrew Kyle Lampinen, Thomas Serre, Katherine Hermann
2024-07-09
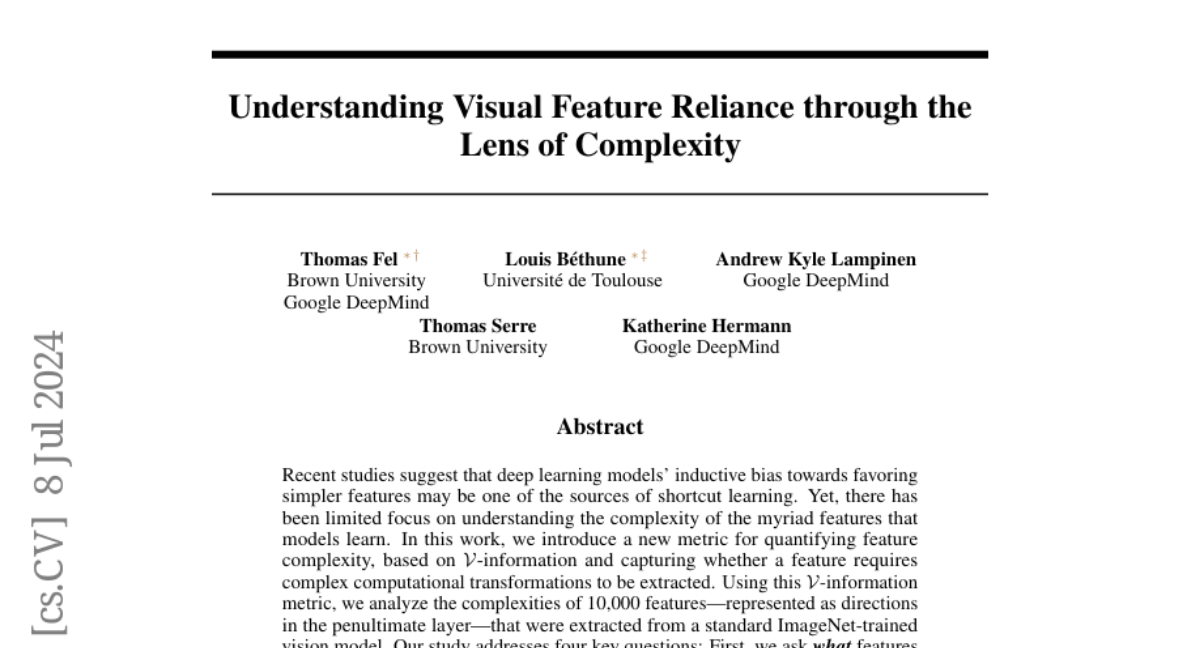
Summary
This paper talks about a new way to understand how deep learning models rely on different visual features by measuring their complexity. It introduces a metric called V-information to analyze how these features are learned and used in models trained on image data.
What's the problem?
The main problem is that deep learning models often prefer simpler features when making decisions, which can lead to shortcut learning—where the model relies on easy patterns instead of understanding the full complexity of the data. However, there hasn't been much research into the complexity of the various features these models learn, making it hard to know how they really work.
What's the solution?
To tackle this issue, the authors developed a new metric called V-information to measure the complexity of features in a model. They analyzed 10,000 features from a standard vision model trained on ImageNet. The study aimed to answer four main questions: what the features look like in terms of complexity, when they are learned during training, how they flow through the network, and how their complexity relates to their importance in the model's decisions. They found that simpler features are learned first and are often more important for decision-making, while complex features take longer to emerge and are less significant.
Why it matters?
This research is important because it helps improve our understanding of how deep learning models function. By examining feature complexity, researchers can develop better models that avoid shortcut learning and make more accurate predictions. This knowledge can lead to advancements in various applications, such as computer vision and artificial intelligence, ensuring these systems are more reliable and effective.
Abstract
Recent studies suggest that deep learning models inductive bias towards favoring simpler features may be one of the sources of shortcut learning. Yet, there has been limited focus on understanding the complexity of the myriad features that models learn. In this work, we introduce a new metric for quantifying feature complexity, based on V-information and capturing whether a feature requires complex computational transformations to be extracted. Using this V-information metric, we analyze the complexities of 10,000 features, represented as directions in the penultimate layer, that were extracted from a standard ImageNet-trained vision model. Our study addresses four key questions: First, we ask what features look like as a function of complexity and find a spectrum of simple to complex features present within the model. Second, we ask when features are learned during training. We find that simpler features dominate early in training, and more complex features emerge gradually. Third, we investigate where within the network simple and complex features flow, and find that simpler features tend to bypass the visual hierarchy via residual connections. Fourth, we explore the connection between features complexity and their importance in driving the networks decision. We find that complex features tend to be less important. Surprisingly, important features become accessible at earlier layers during training, like a sedimentation process, allowing the model to build upon these foundational elements.