UniAff: A Unified Representation of Affordances for Tool Usage and Articulation with Vision-Language Models
Qiaojun Yu, Siyuan Huang, Xibin Yuan, Zhengkai Jiang, Ce Hao, Xin Li, Haonan Chang, Junbo Wang, Liu Liu, Hongsheng Li, Peng Gao, Cewu Lu
2024-10-01
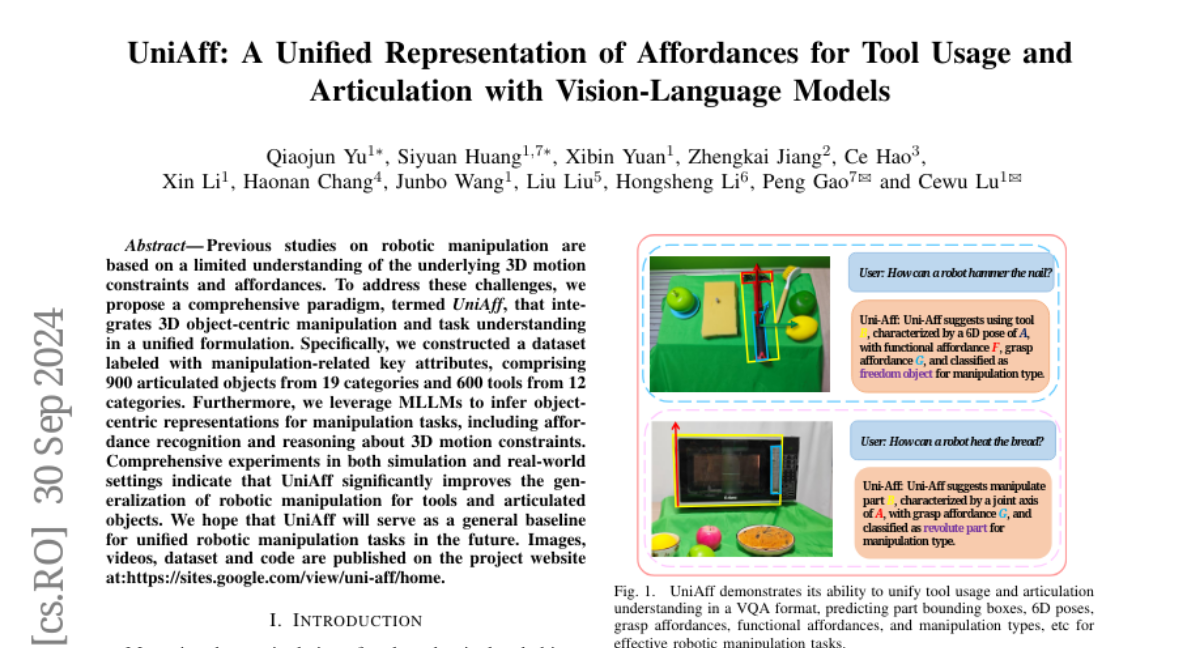
Summary
This paper introduces UniAff, a new framework that helps robots understand how to use tools and manipulate objects by integrating 3D motion and task understanding.
What's the problem?
Robots often struggle with manipulating objects because they lack a comprehensive understanding of how to move and interact with different tools. Previous research has not fully addressed the complexities of 3D motion and the specific actions needed for various tasks, leading to limitations in robotic capabilities.
What's the solution?
UniAff solves this problem by creating a unified system that combines knowledge of 3D object manipulation and task understanding. The researchers developed a large dataset with detailed information about 900 articulated objects and 600 tools, including how these objects can be used. They also used advanced models to help robots recognize these objects and understand their motion constraints, allowing for better manipulation in both simulations and real-world settings.
Why it matters?
This research is significant because it provides a better foundation for robotic manipulation, making robots more capable of understanding and using tools effectively. By improving how robots learn to interact with their environment, UniAff could lead to advancements in robotics applications, such as automation in manufacturing, healthcare, and assistive technologies.
Abstract
Previous studies on robotic manipulation are based on a limited understanding of the underlying 3D motion constraints and affordances. To address these challenges, we propose a comprehensive paradigm, termed UniAff, that integrates 3D object-centric manipulation and task understanding in a unified formulation. Specifically, we constructed a dataset labeled with manipulation-related key attributes, comprising 900 articulated objects from 19 categories and 600 tools from 12 categories. Furthermore, we leverage MLLMs to infer object-centric representations for manipulation tasks, including affordance recognition and reasoning about 3D motion constraints. Comprehensive experiments in both simulation and real-world settings indicate that UniAff significantly improves the generalization of robotic manipulation for tools and articulated objects. We hope that UniAff will serve as a general baseline for unified robotic manipulation tasks in the future. Images, videos, dataset, and code are published on the project website at:https://sites.google.com/view/uni-aff/home