UniBench: Visual Reasoning Requires Rethinking Vision-Language Beyond Scaling
Haider Al-Tahan, Quentin Garrido, Randall Balestriero, Diane Bouchacourt, Caner Hazirbas, Mark Ibrahim
2024-08-12
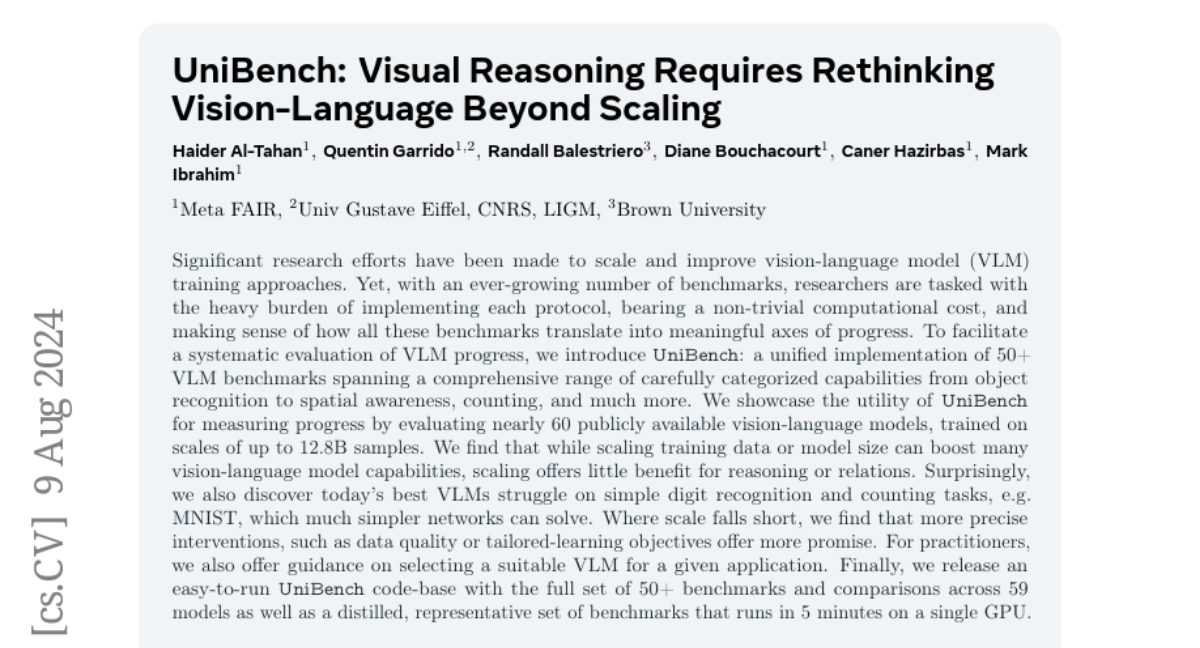
Summary
This paper introduces UniBench, a new evaluation framework designed to assess the capabilities of Vision-Language Models (VLMs) in a comprehensive way, focusing on their visual reasoning skills.
What's the problem?
As researchers develop more advanced VLMs, simply making these models larger or training them on more data isn't enough to ensure they understand complex visual tasks. There are many benchmarks available, but they can be overwhelming and costly to implement, making it hard for researchers to track real progress in visual reasoning abilities.
What's the solution?
To address these challenges, the authors created UniBench, which includes over 50 standardized benchmarks that cover a wide range of skills needed for visual reasoning, such as object recognition and spatial awareness. By evaluating nearly 60 different VLMs using this unified framework, they found that while increasing the size of models helps with some tasks, it doesn't improve their ability to reason about images or understand relationships between objects. Instead, focusing on data quality and specific learning goals proved to be more effective.
Why it matters?
This research is significant because it provides a structured way for researchers to evaluate and compare the performance of VLMs. By highlighting the limitations of current models and suggesting areas for improvement, UniBench can help guide future research efforts toward developing better AI systems that can reason about and interact with the visual world more effectively.
Abstract
Significant research efforts have been made to scale and improve vision-language model (VLM) training approaches. Yet, with an ever-growing number of benchmarks, researchers are tasked with the heavy burden of implementing each protocol, bearing a non-trivial computational cost, and making sense of how all these benchmarks translate into meaningful axes of progress. To facilitate a systematic evaluation of VLM progress, we introduce UniBench: a unified implementation of 50+ VLM benchmarks spanning a comprehensive range of carefully categorized capabilities from object recognition to spatial awareness, counting, and much more. We showcase the utility of UniBench for measuring progress by evaluating nearly 60 publicly available vision-language models, trained on scales of up to 12.8B samples. We find that while scaling training data or model size can boost many vision-language model capabilities, scaling offers little benefit for reasoning or relations. Surprisingly, we also discover today's best VLMs struggle on simple digit recognition and counting tasks, e.g. MNIST, which much simpler networks can solve. Where scale falls short, we find that more precise interventions, such as data quality or tailored-learning objectives offer more promise. For practitioners, we also offer guidance on selecting a suitable VLM for a given application. Finally, we release an easy-to-run UniBench code-base with the full set of 50+ benchmarks and comparisons across 59 models as well as a distilled, representative set of benchmarks that runs in 5 minutes on a single GPU.