UniReal: Universal Image Generation and Editing via Learning Real-world Dynamics
Xi Chen, Zhifei Zhang, He Zhang, Yuqian Zhou, Soo Ye Kim, Qing Liu, Yijun Li, Jianming Zhang, Nanxuan Zhao, Yilin Wang, Hui Ding, Zhe Lin, Hengshuang Zhao
2024-12-11
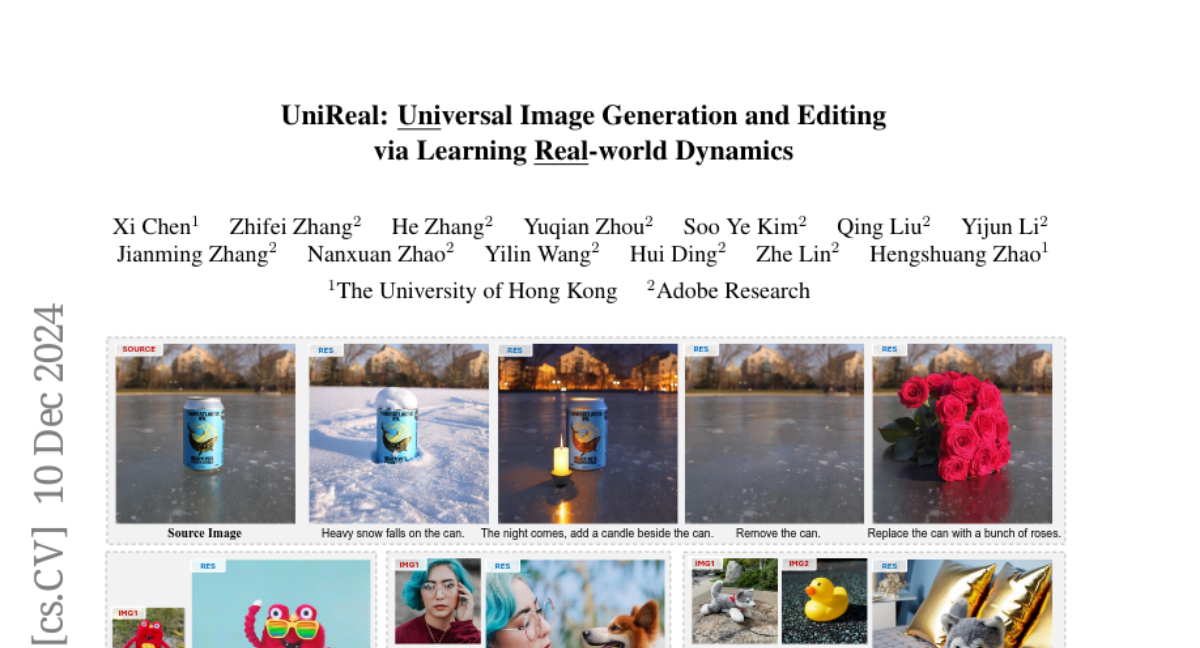
Summary
This paper talks about UniReal, a new system that can generate and edit images by learning from real-world video dynamics, allowing for a wide range of creative tasks.
What's the problem?
Current methods for generating and editing images often focus on specific tasks, making it hard to create a unified system that can handle various image-related tasks like generation, editing, and customization. Additionally, these methods struggle to maintain consistency and capture the natural variations found in real-world visuals.
What's the solution?
The authors propose UniReal, which treats image generation and editing tasks like creating frames in a video. By using large-scale videos as training data, UniReal learns how different elements in images interact, such as shadows and reflections. This allows it to perform multiple tasks seamlessly, including generating new images and editing existing ones while maintaining a coherent style and quality.
Why it matters?
This research is important because it simplifies the process of creating high-quality images and allows for more flexibility in artistic expression. By bridging the gap between different image tasks, UniReal can be used in various fields such as graphic design, animation, and game development, making it easier for creators to bring their ideas to life.
Abstract
We introduce UniReal, a unified framework designed to address various image generation and editing tasks. Existing solutions often vary by tasks, yet share fundamental principles: preserving consistency between inputs and outputs while capturing visual variations. Inspired by recent video generation models that effectively balance consistency and variation across frames, we propose a unifying approach that treats image-level tasks as discontinuous video generation. Specifically, we treat varying numbers of input and output images as frames, enabling seamless support for tasks such as image generation, editing, customization, composition, etc. Although designed for image-level tasks, we leverage videos as a scalable source for universal supervision. UniReal learns world dynamics from large-scale videos, demonstrating advanced capability in handling shadows, reflections, pose variation, and object interaction, while also exhibiting emergent capability for novel applications.