UniTalker: Scaling up Audio-Driven 3D Facial Animation through A Unified Model
Xiangyu Fan, Jiaqi Li, Zhiqian Lin, Weiye Xiao, Lei Yang
2024-08-02
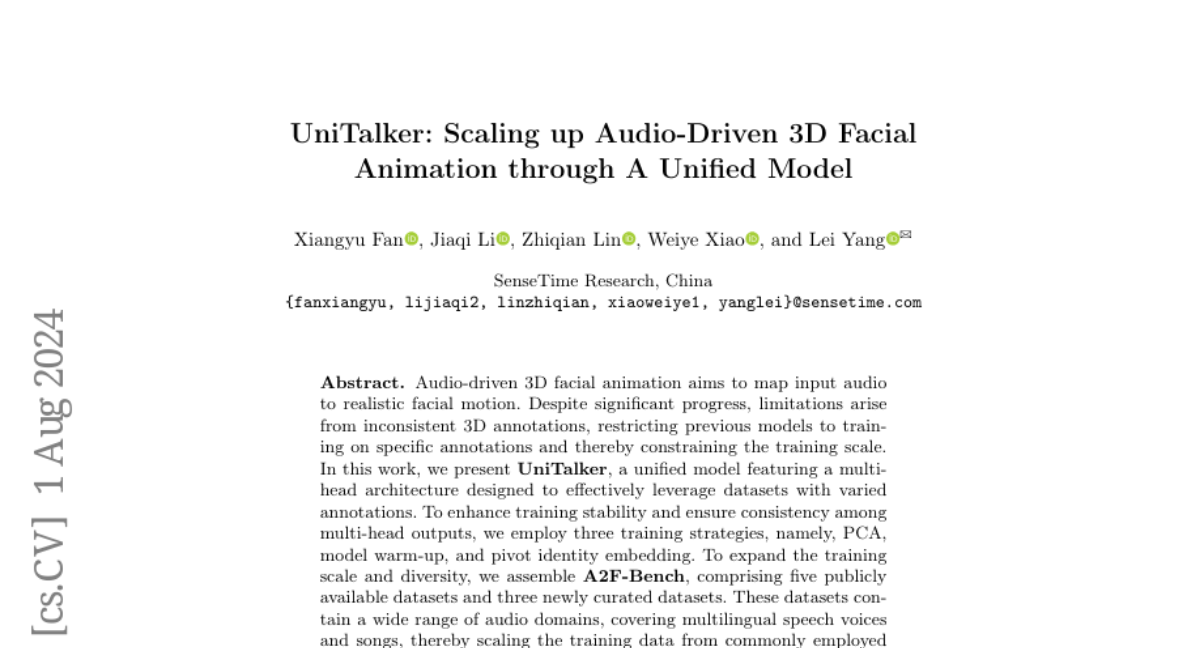
Summary
This paper presents UniTalker, a new model designed to create realistic 3D facial animations from audio inputs. It aims to improve how well animated characters can mimic human speech and expressions based on what they hear.
What's the problem?
Creating convincing 3D facial animations that accurately match spoken audio is challenging. Previous methods often struggled because they relied on inconsistent data and could only handle specific types of audio. This made it difficult to train models that could work well across different scenarios, such as various languages or emotional expressions.
What's the solution?
To address these issues, the authors developed UniTalker, which uses a unified model that can learn from a wide range of audio sources. They combined multiple datasets—covering different languages and audio types—to train the model effectively. UniTalker employs advanced techniques like PCA (Principal Component Analysis) for better training stability and uses a multi-head architecture to ensure consistent outputs. This allows it to generate high-quality animations with less error compared to previous models.
Why it matters?
This research is significant because it enhances the ability of AI to create lifelike animations for applications such as video games, virtual assistants, and movies. By improving the synchronization between audio and facial movements, UniTalker can make digital characters more engaging and realistic, ultimately leading to better user experiences in interactive media.
Abstract
Audio-driven 3D facial animation aims to map input audio to realistic facial motion. Despite significant progress, limitations arise from inconsistent 3D annotations, restricting previous models to training on specific annotations and thereby constraining the training scale. In this work, we present UniTalker, a unified model featuring a multi-head architecture designed to effectively leverage datasets with varied annotations. To enhance training stability and ensure consistency among multi-head outputs, we employ three training strategies, namely, PCA, model warm-up, and pivot identity embedding. To expand the training scale and diversity, we assemble A2F-Bench, comprising five publicly available datasets and three newly curated datasets. These datasets contain a wide range of audio domains, covering multilingual speech voices and songs, thereby scaling the training data from commonly employed datasets, typically less than 1 hour, to 18.5 hours. With a single trained UniTalker model, we achieve substantial lip vertex error reductions of 9.2% for BIWI dataset and 13.7% for Vocaset. Additionally, the pre-trained UniTalker exhibits promise as the foundation model for audio-driven facial animation tasks. Fine-tuning the pre-trained UniTalker on seen datasets further enhances performance on each dataset, with an average error reduction of 6.3% on A2F-Bench. Moreover, fine-tuning UniTalker on an unseen dataset with only half the data surpasses prior state-of-the-art models trained on the full dataset. The code and dataset are available at the project page https://github.com/X-niper/UniTalker.