Unpacking SDXL Turbo: Interpreting Text-to-Image Models with Sparse Autoencoders
Viacheslav Surkov, Chris Wendler, Mikhail Terekhov, Justin Deschenaux, Robert West, Caglar Gulcehre
2024-11-01
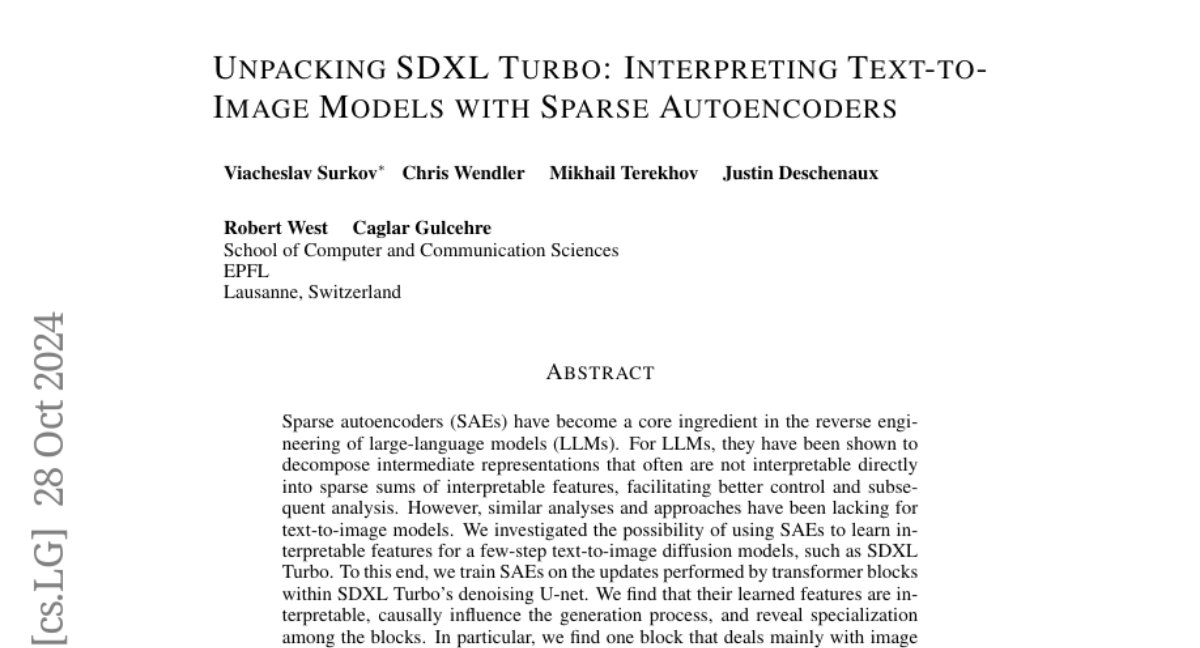
Summary
This paper discusses how to use Sparse Autoencoders (SAEs) to better understand and interpret text-to-image models like SDXL Turbo, which generate images based on text descriptions.
What's the problem?
Text-to-image models can create impressive images from written prompts, but it’s often unclear how these models work internally. Unlike large language models, which have been studied in detail, there hasn’t been much analysis of text-to-image models. This lack of understanding makes it difficult to control and improve these models effectively.
What's the solution?
The authors propose using SAEs to analyze the inner workings of the SDXL Turbo model. They train these autoencoders on the updates made by the model's transformer blocks, allowing them to identify and interpret key features that influence image generation. They discover that different parts of the model specialize in specific tasks, such as composing images, adding details, or adjusting colors and styles. This approach provides valuable insights into how text-to-image models operate.
Why it matters?
This research is important because it helps demystify how text-to-image models generate images. By understanding the features learned by SAEs, developers can gain better control over these models, leading to improvements in image quality and relevance. This knowledge can enhance applications in art, design, and any field where visual content is created from textual descriptions.
Abstract
Sparse autoencoders (SAEs) have become a core ingredient in the reverse engineering of large-language models (LLMs). For LLMs, they have been shown to decompose intermediate representations that often are not interpretable directly into sparse sums of interpretable features, facilitating better control and subsequent analysis. However, similar analyses and approaches have been lacking for text-to-image models. We investigated the possibility of using SAEs to learn interpretable features for a few-step text-to-image diffusion models, such as SDXL Turbo. To this end, we train SAEs on the updates performed by transformer blocks within SDXL Turbo's denoising U-net. We find that their learned features are interpretable, causally influence the generation process, and reveal specialization among the blocks. In particular, we find one block that deals mainly with image composition, one that is mainly responsible for adding local details, and one for color, illumination, and style. Therefore, our work is an important first step towards better understanding the internals of generative text-to-image models like SDXL Turbo and showcases the potential of features learned by SAEs for the visual domain. Code is available at https://github.com/surkovv/sdxl-unbox