UnUnlearning: Unlearning is not sufficient for content regulation in advanced generative AI
Ilia Shumailov, Jamie Hayes, Eleni Triantafillou, Guillermo Ortiz-Jimenez, Nicolas Papernot, Matthew Jagielski, Itay Yona, Heidi Howard, Eugene Bagdasaryan
2024-07-02
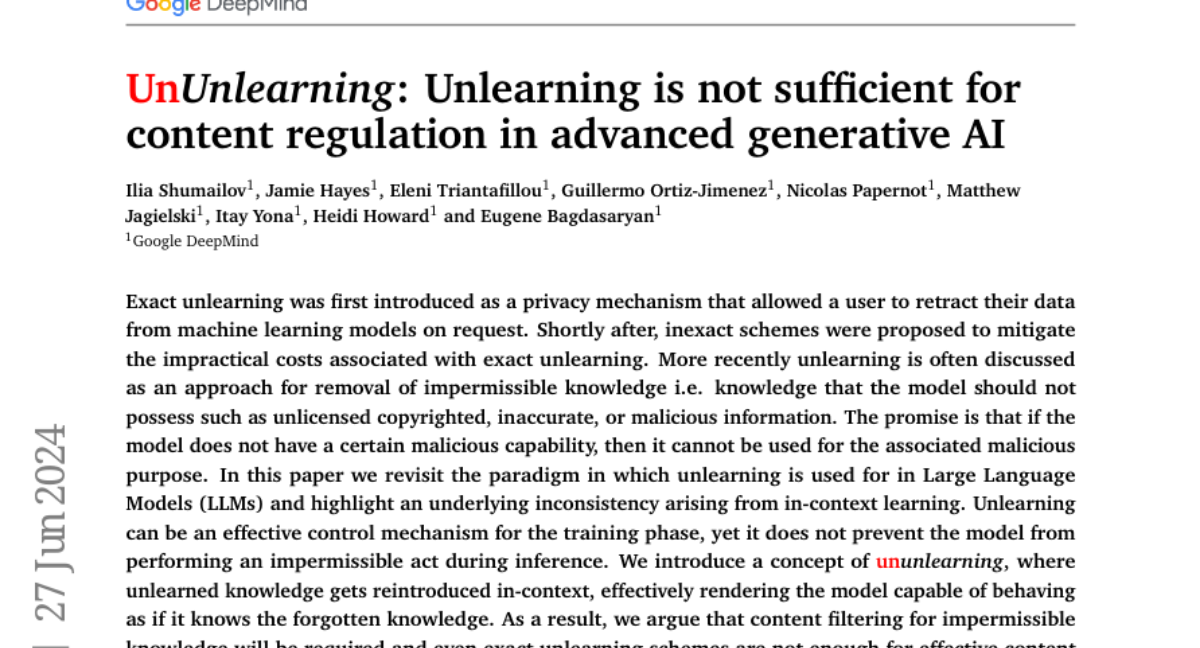
Summary
This paper discusses a new concept called 'ununlearning' in the context of generative AI, particularly focusing on how traditional unlearning methods may not be enough to prevent AI models from using harmful or unwanted knowledge.
What's the problem?
The main problem is that while unlearning allows AI models to forget specific data, it does not stop them from using that information in real-time situations, known as inference. This means that even if a model has 'forgotten' certain harmful knowledge, it can still act as if it knows that information when prompted in specific contexts. This inconsistency poses a risk for content regulation, especially regarding sensitive or malicious information.
What's the solution?
To address this issue, the authors introduce the idea of 'ununlearning,' which suggests that simply unlearning knowledge is not sufficient. Instead, they propose that additional content filtering mechanisms are necessary to prevent models from accessing or using impermissible knowledge during inference. They explore the feasibility of implementing ununlearning in modern large language models (LLMs) and discuss its implications for content regulation.
Why it matters?
This research is important because it highlights the limitations of current unlearning techniques in AI and emphasizes the need for more robust content regulation strategies. As generative AI becomes more prevalent, ensuring that these systems do not inadvertently use harmful or inappropriate information is crucial for ethical and safe AI deployment.
Abstract
Exact unlearning was first introduced as a privacy mechanism that allowed a user to retract their data from machine learning models on request. Shortly after, inexact schemes were proposed to mitigate the impractical costs associated with exact unlearning. More recently unlearning is often discussed as an approach for removal of impermissible knowledge i.e. knowledge that the model should not possess such as unlicensed copyrighted, inaccurate, or malicious information. The promise is that if the model does not have a certain malicious capability, then it cannot be used for the associated malicious purpose. In this paper we revisit the paradigm in which unlearning is used for in Large Language Models (LLMs) and highlight an underlying inconsistency arising from in-context learning. Unlearning can be an effective control mechanism for the training phase, yet it does not prevent the model from performing an impermissible act during inference. We introduce a concept of ununlearning, where unlearned knowledge gets reintroduced in-context, effectively rendering the model capable of behaving as if it knows the forgotten knowledge. As a result, we argue that content filtering for impermissible knowledge will be required and even exact unlearning schemes are not enough for effective content regulation. We discuss feasibility of ununlearning for modern LLMs and examine broader implications.