ViBe: A Text-to-Video Benchmark for Evaluating Hallucination in Large Multimodal Models
Vipula Rawte, Sarthak Jain, Aarush Sinha, Garv Kaushik, Aman Bansal, Prathiksha Rumale Vishwanath, Samyak Rajesh Jain, Aishwarya Naresh Reganti, Vinija Jain, Aman Chadha, Amit P. Sheth, Amitava Das
2024-11-21
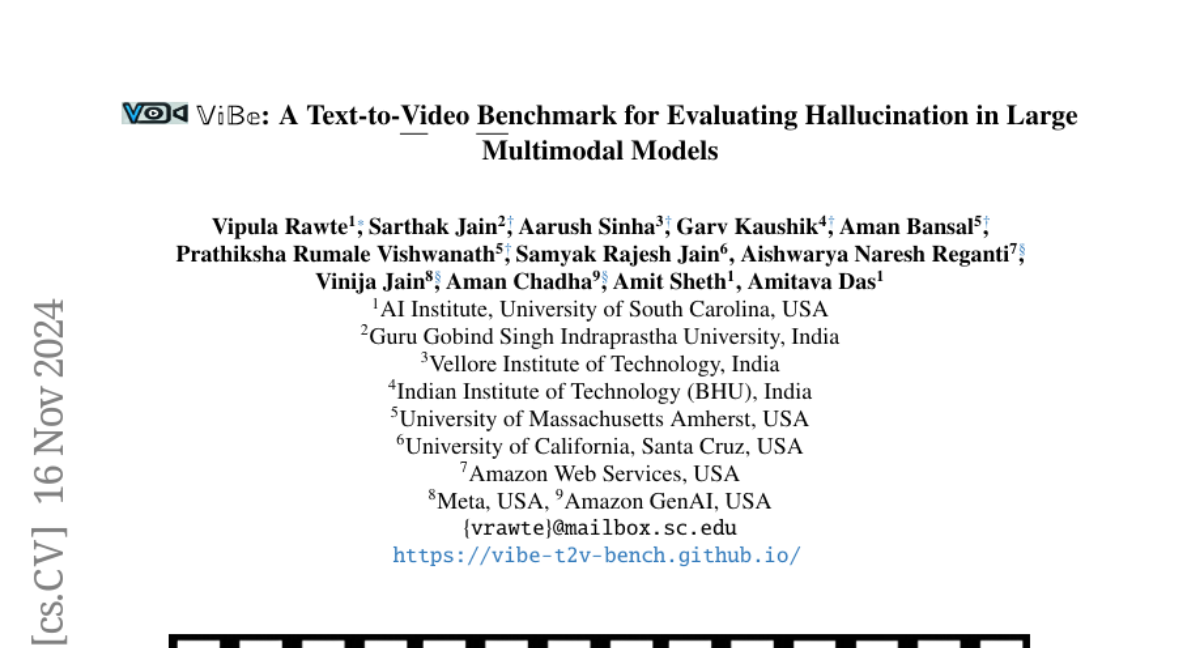
Summary
This paper presents ViBe, a benchmark designed to evaluate hallucinations in Text-to-Video (T2V) models, which are AI systems that create videos based on text descriptions.
What's the problem?
While T2V models have improved in generating videos from text, they often produce 'hallucinations'—incorrect or nonsensical content that indicates the video is AI-generated. This can include issues like characters disappearing, numbers being inconsistent, or actions not matching the timeline of the video. These problems make it hard to trust the quality of videos generated by these models.
What's the solution?
ViBe addresses this issue by creating a large dataset of 3,782 videos generated by various T2V models, which are categorized into five types of hallucinations: Vanishing Subject, Numeric Variability, Temporal Dysmorphia, Omission Error, and Physical Incongruity. The dataset is annotated by humans to help researchers evaluate and improve the reliability of T2V models. The paper also establishes a baseline for classifying these hallucinations and tests different methods to improve detection accuracy.
Why it matters?
This research is significant because it provides a structured way to assess and improve the performance of T2V models. By identifying and categorizing hallucinations, ViBe helps developers create more accurate and trustworthy AI systems for generating videos from text, which can enhance applications in entertainment, education, and beyond.
Abstract
Latest developments in Large Multimodal Models (LMMs) have broadened their capabilities to include video understanding. Specifically, Text-to-video (T2V) models have made significant progress in quality, comprehension, and duration, excelling at creating videos from simple textual prompts. Yet, they still frequently produce hallucinated content that clearly signals the video is AI-generated. We introduce ViBe: a large-scale Text-to-Video Benchmark of hallucinated videos from T2V models. We identify five major types of hallucination: Vanishing Subject, Numeric Variability, Temporal Dysmorphia, Omission Error, and Physical Incongruity. Using 10 open-source T2V models, we developed the first large-scale dataset of hallucinated videos, comprising 3,782 videos annotated by humans into these five categories. ViBe offers a unique resource for evaluating the reliability of T2V models and provides a foundation for improving hallucination detection and mitigation in video generation. We establish classification as a baseline and present various ensemble classifier configurations, with the TimeSFormer + CNN combination yielding the best performance, achieving 0.345 accuracy and 0.342 F1 score. This benchmark aims to drive the development of robust T2V models that produce videos more accurately aligned with input prompts.