Video-3D LLM: Learning Position-Aware Video Representation for 3D Scene Understanding
Duo Zheng, Shijia Huang, Liwei Wang
2024-12-05
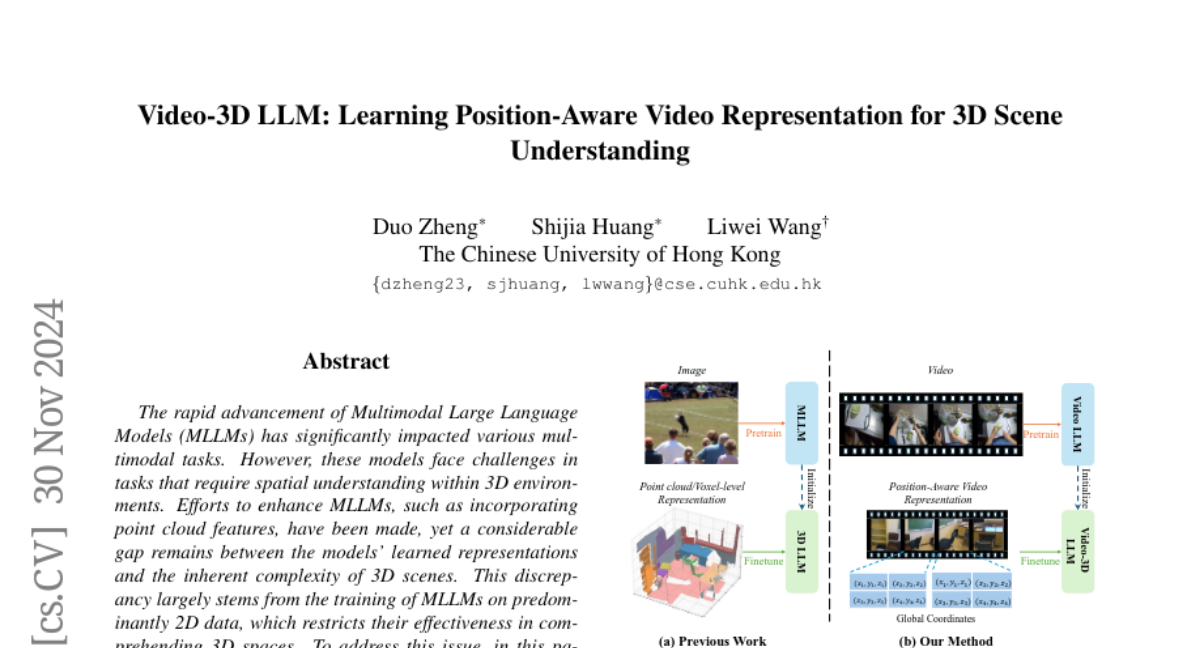
Summary
This paper introduces Video-3D LLM, a new model designed to improve the understanding of 3D scenes by treating them like dynamic videos and incorporating 3D position information.
What's the problem?
Many existing large language models (LLMs) struggle with understanding 3D environments because they are mostly trained on 2D data. This limitation makes it hard for these models to accurately interpret the spatial relationships and complexities found in 3D scenes, which are crucial for tasks like navigation or object recognition in real-world settings.
What's the solution?
To solve this problem, the researchers developed Video-3D LLM, which treats 3D scenes as sequences of video frames. This model uses a special method called 3D position encoding to help it understand the spatial context of the images better. Additionally, it employs a technique called maximum coverage sampling to balance performance and computational efficiency. By integrating these features, Video-3D LLM can generate more accurate representations of 3D environments.
Why it matters?
This research is important because it enhances how AI systems can comprehend and interact with the three-dimensional world. By improving the ability of models to understand complex 3D scenes, Video-3D LLM can be applied in various fields such as robotics, virtual reality, and autonomous vehicles, where accurate spatial understanding is essential for effective operation.
Abstract
The rapid advancement of Multimodal Large Language Models (MLLMs) has significantly impacted various multimodal tasks. However, these models face challenges in tasks that require spatial understanding within 3D environments. Efforts to enhance MLLMs, such as incorporating point cloud features, have been made, yet a considerable gap remains between the models' learned representations and the inherent complexity of 3D scenes. This discrepancy largely stems from the training of MLLMs on predominantly 2D data, which restricts their effectiveness in comprehending 3D spaces. To address this issue, in this paper, we propose a novel generalist model, i.e., Video-3D LLM, for 3D scene understanding. By treating 3D scenes as dynamic videos and incorporating 3D position encoding into these representations, our Video-3D LLM aligns video representations with real-world spatial contexts more accurately. Additionally, we have implemented a maximum coverage sampling technique to optimize the balance between computational costs and performance efficiency. Extensive experiments demonstrate that our model achieves state-of-the-art performance on several 3D scene understanding benchmarks, including ScanRefer, Multi3DRefer, Scan2Cap, ScanQA, and SQA3D.