Video-Foley: Two-Stage Video-To-Sound Generation via Temporal Event Condition For Foley Sound
Junwon Lee, Jaekwon Im, Dabin Kim, Juhan Nam
2024-08-23
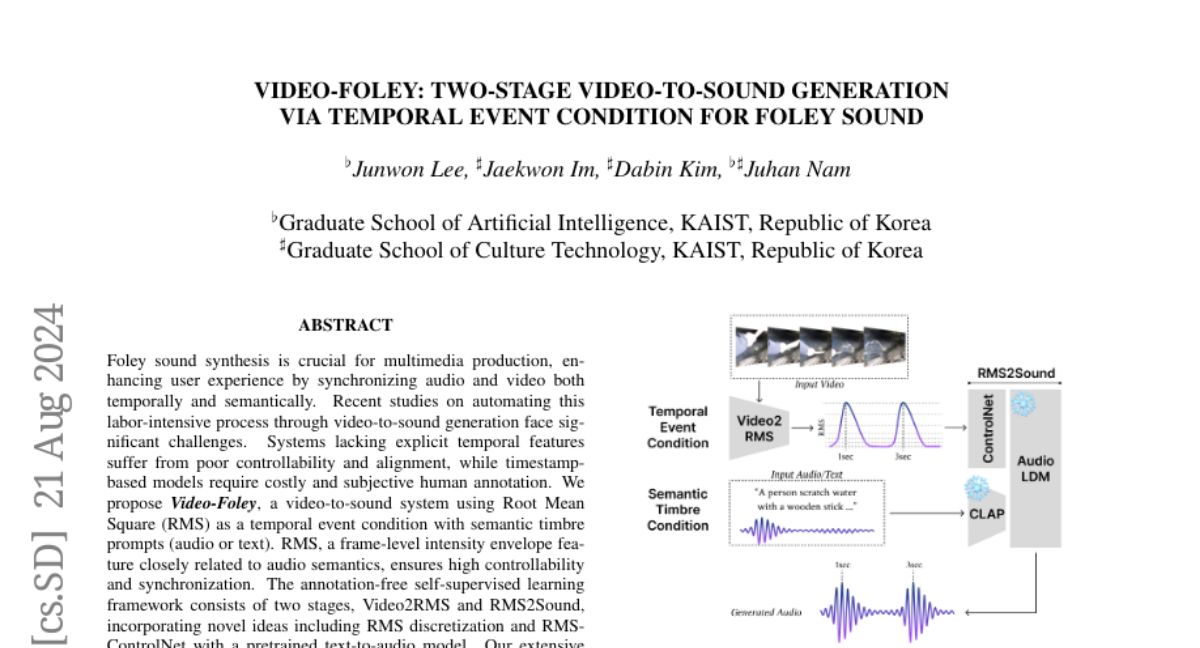
Summary
This paper presents Video-Foley, a new system that automatically generates sound effects for videos by analyzing the events happening in the video.
What's the problem?
Creating sound effects for videos, known as Foley sound synthesis, is usually a time-consuming and complex process that requires a lot of human effort. Existing methods struggle with accurately syncing sounds with video content, and many require expensive and subjective human annotations to work effectively.
What's the solution?
To tackle these issues, the authors developed Video-Foley, which uses a two-stage process to generate sound from video automatically. The first stage analyzes the video to create a temporal event condition using Root Mean Square (RMS) values, which helps in understanding the intensity of sounds needed. The second stage generates the actual sound effects based on this analysis without needing manual annotations. This system improves control over the timing and quality of the sounds produced.
Why it matters?
This research is important because it makes the process of adding sound effects to videos much easier and more efficient. By automating Foley sound synthesis, Video-Foley can save time and resources in multimedia production, allowing creators to focus more on storytelling and less on technical details.
Abstract
Foley sound synthesis is crucial for multimedia production, enhancing user experience by synchronizing audio and video both temporally and semantically. Recent studies on automating this labor-intensive process through video-to-sound generation face significant challenges. Systems lacking explicit temporal features suffer from poor controllability and alignment, while timestamp-based models require costly and subjective human annotation. We propose Video-Foley, a video-to-sound system using Root Mean Square (RMS) as a temporal event condition with semantic timbre prompts (audio or text). RMS, a frame-level intensity envelope feature closely related to audio semantics, ensures high controllability and synchronization. The annotation-free self-supervised learning framework consists of two stages, Video2RMS and RMS2Sound, incorporating novel ideas including RMS discretization and RMS-ControlNet with a pretrained text-to-audio model. Our extensive evaluation shows that Video-Foley achieves state-of-the-art performance in audio-visual alignment and controllability for sound timing, intensity, timbre, and nuance. Code, model weights, and demonstrations are available on the accompanying website. (https://jnwnlee.github.io/video-foley-demo)