Video SimpleQA: Towards Factuality Evaluation in Large Video Language Models
Meng Cao, Pengfei Hu, Yingyao Wang, Jihao Gu, Haoran Tang, Haoze Zhao, Jiahua Dong, Wangbo Yu, Ge Zhang, Ian Reid, Xiaodan Liang
2025-03-25
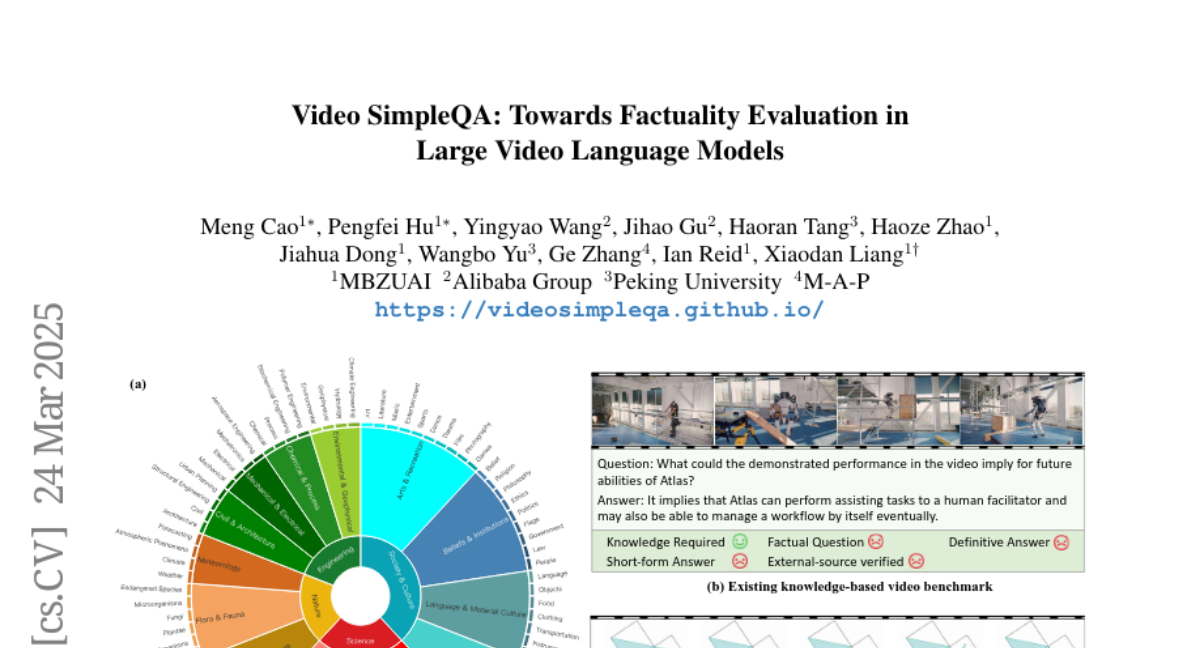
Summary
This paper is about creating a new way to test how well AI models understand videos and answer questions about them accurately.
What's the problem?
Current AI models can understand videos to some extent, but it's hard to tell if their answers are actually based on facts or just guesses.
What's the solution?
The researchers created a test called Video SimpleQA that asks AI models questions about videos that require them to use outside knowledge and provide short, clear answers that can be easily checked for accuracy.
Why it matters?
This work matters because it helps us develop AI models that can truly understand videos and provide reliable information, which is important for many applications like education, entertainment, and security.
Abstract
Recent advancements in Large Video Language Models (LVLMs) have highlighted their potential for multi-modal understanding, yet evaluating their factual grounding in video contexts remains a critical unsolved challenge. To address this gap, we introduce Video SimpleQA, the first comprehensive benchmark tailored for factuality evaluation of LVLMs. Our work distinguishes from existing video benchmarks through the following key features: 1) Knowledge required: demanding integration of external knowledge beyond the explicit narrative; 2) Fact-seeking question: targeting objective, undisputed events or relationships, avoiding subjective interpretation; 3) Definitive & short-form answer: Answers are crafted as unambiguous and definitively correct in a short format, enabling automated evaluation through LLM-as-a-judge frameworks with minimal scoring variance; 4) External-source verified: All annotations undergo rigorous validation against authoritative external references to ensure the reliability; 5) Temporal reasoning required: The annotated question types encompass both static single-frame understanding and dynamic temporal reasoning, explicitly evaluating LVLMs factuality under the long-context dependencies. We extensively evaluate 41 state-of-the-art LVLMs and summarize key findings as follows: 1) Current LVLMs exhibit notable deficiencies in factual adherence, particularly for open-source models. The best-performing model Gemini-1.5-Pro achieves merely an F-score of 54.4%; 2) Test-time compute paradigms show insignificant performance gains, revealing fundamental constraints for enhancing factuality through post-hoc computation; 3) Retrieval-Augmented Generation demonstrates consistent improvements at the cost of additional inference time overhead, presenting a critical efficiency-performance trade-off.