VideoLLM-online: Online Video Large Language Model for Streaming Video
Joya Chen, Zhaoyang Lv, Shiwei Wu, Kevin Qinghong Lin, Chenan Song, Difei Gao, Jia-Wei Liu, Ziteng Gao, Dongxing Mao, Mike Zheng Shou
2024-06-18
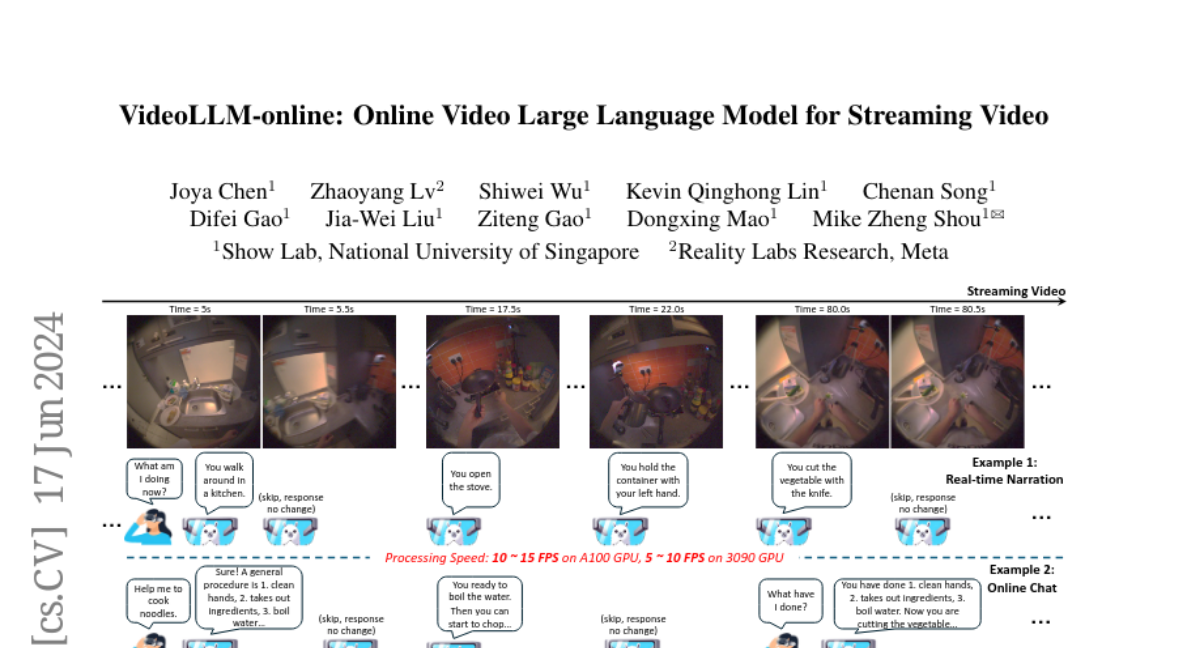
Summary
This paper discusses VideoLLM-online, a new model designed to understand and interact with streaming videos in real-time. It introduces a framework called Learning-In-Video-Stream (LIVE) that allows the model to have conversations while watching a continuous video, rather than just analyzing it as a series of separate clips.
What's the problem?
Most existing models that analyze videos treat them as fixed clips, which means they can't effectively handle live video streams. This limitation makes it hard for these models to respond to questions or provide information based on what is happening in the video at any given moment. As a result, they struggle to maintain a conversation that aligns with the ongoing events in the video.
What's the solution?
To solve this problem, the authors developed the LIVE framework, which includes several key features. First, it sets up a training goal specifically for handling continuous video inputs. Second, it creates a method to turn traditional video annotations into a format suitable for real-time dialogue. Finally, it optimizes how the model processes information so that it can respond quickly while watching the video. With this framework, they built the VideoLLM-online model using Llama-2 and Llama-3, which can process streaming videos at over 10 frames per second (FPS) on powerful GPUs.
Why it matters?
This research is important because it enhances how AI can interact with live video content, making it more useful for applications like virtual assistants or interactive media. By allowing models to engage in real-time conversations about ongoing events in videos, this technology can improve user experiences in various fields, such as education, entertainment, and customer support.
Abstract
Recent Large Language Models have been enhanced with vision capabilities, enabling them to comprehend images, videos, and interleaved vision-language content. However, the learning methods of these large multimodal models typically treat videos as predetermined clips, making them less effective and efficient at handling streaming video inputs. In this paper, we propose a novel Learning-In-Video-Stream (LIVE) framework, which enables temporally aligned, long-context, and real-time conversation within a continuous video stream. Our LIVE framework comprises comprehensive approaches to achieve video streaming dialogue, encompassing: (1) a training objective designed to perform language modeling for continuous streaming inputs, (2) a data generation scheme that converts offline temporal annotations into a streaming dialogue format, and (3) an optimized inference pipeline to speed up the model responses in real-world video streams. With our LIVE framework, we built VideoLLM-online model upon Llama-2/Llama-3 and demonstrate its significant advantages in processing streaming videos. For instance, on average, our model can support streaming dialogue in a 5-minute video clip at over 10 FPS on an A100 GPU. Moreover, it also showcases state-of-the-art performance on public offline video benchmarks, such as recognition, captioning, and forecasting. The code, model, data, and demo have been made available at https://showlab.github.io/videollm-online.