VideoWebArena: Evaluating Long Context Multimodal Agents with Video Understanding Web Tasks
Lawrence Jang, Yinheng Li, Charles Ding, Justin Lin, Paul Pu Liang, Dan Zhao, Rogerio Bonatti, Kazuhito Koishida
2024-10-29
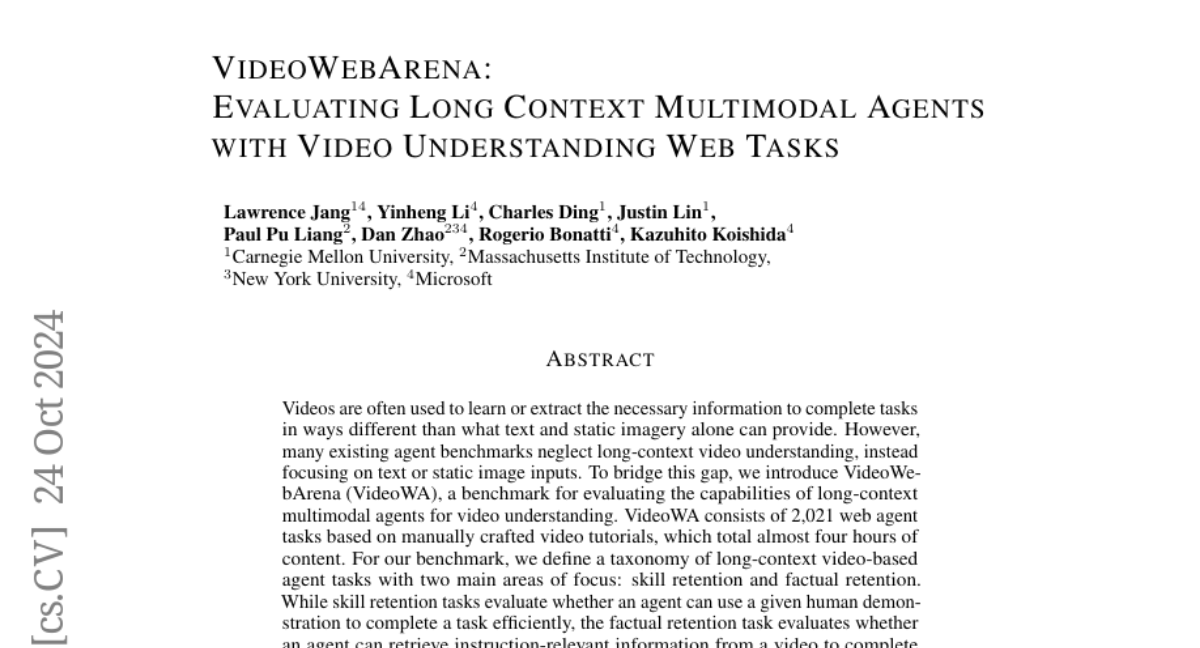
Summary
This paper introduces VideoWebArena, a new benchmark designed to evaluate how well multimodal agents (AI systems that understand both text and video) can perform tasks that require understanding long videos.
What's the problem?
Many existing benchmarks for AI agents focus only on text or static images, ignoring the challenges of understanding long videos. This is a problem because videos provide valuable information that can't be captured by just looking at pictures or reading text, especially when it comes to tasks that require remembering skills or facts demonstrated in the video.
What's the solution?
To address this gap, the authors created VideoWebArena, which includes 2,021 tasks based on video tutorials totaling nearly four hours of content. These tasks are divided into two main types: skill retention tasks, which test if an agent can use a video tutorial to complete a task, and factual retention tasks, which check if an agent can find specific information from the video to help with a task. The benchmark evaluates how well different AI models perform on these tasks, revealing that current models still lag behind human performance.
Why it matters?
This research is important because it highlights the need for better evaluation methods for AI systems that work with videos. By providing a comprehensive benchmark like VideoWebArena, researchers can identify weaknesses in current models and work towards developing more capable AI agents that can understand and utilize video content effectively in real-world scenarios.
Abstract
Videos are often used to learn or extract the necessary information to complete tasks in ways different than what text and static imagery alone can provide. However, many existing agent benchmarks neglect long-context video understanding, instead focusing on text or static image inputs. To bridge this gap, we introduce VideoWebArena (VideoWA), a benchmark for evaluating the capabilities of long-context multimodal agents for video understanding. VideoWA consists of 2,021 web agent tasks based on manually crafted video tutorials, which total almost four hours of content. For our benchmark, we define a taxonomy of long-context video-based agent tasks with two main areas of focus: skill retention and factual retention. While skill retention tasks evaluate whether an agent can use a given human demonstration to complete a task efficiently, the factual retention task evaluates whether an agent can retrieve instruction-relevant information from a video to complete a task. We find that the best model achieves 13.3% success on factual retention tasks and 45.8% on factual retention QA pairs, far below human performance at 73.9% and 79.3%, respectively. On skill retention tasks, long-context models perform worse with tutorials than without, exhibiting a 5% performance decrease in WebArena tasks and a 10.3% decrease in VisualWebArena tasks. Our work highlights the need to improve the agentic abilities of long-context multimodal models and provides a testbed for future development with long-context video agents.