VidGen-1M: A Large-Scale Dataset for Text-to-video Generation
Zhiyu Tan, Xiaomeng Yang, Luozheng Qin, Hao Li
2024-08-06
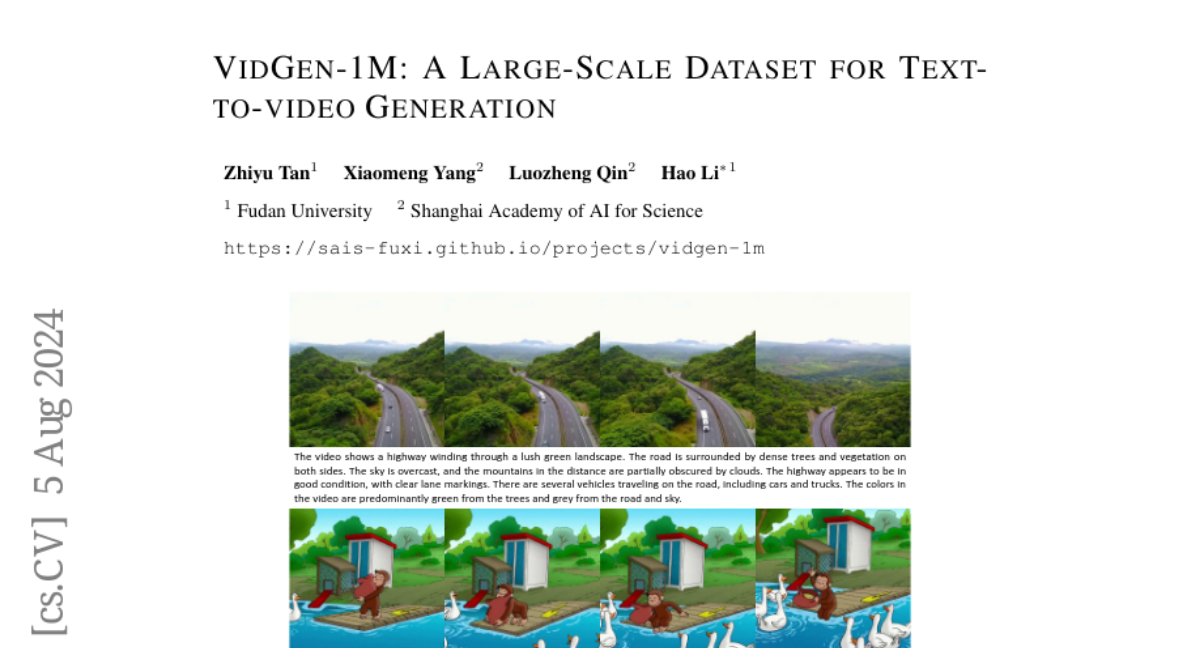
Summary
This paper introduces VidGen-1M, a new large-scale dataset designed to improve the training of text-to-video generation models by providing high-quality video and text pairs.
What's the problem?
Current datasets for training text-to-video models have several issues, such as inconsistent timing between video frames, low-quality captions, poor video quality, and uneven distribution of data. These problems make it difficult for models to learn effectively and generate good videos.
What's the solution?
The authors created VidGen-1M using a careful process that combines different curation strategies to ensure high-quality videos and detailed captions. This dataset focuses on maintaining temporal consistency, which means that the timing of actions in the video matches what is described in the text. When tested, models trained with VidGen-1M performed better than those trained on existing datasets.
Why it matters?
VidGen-1M is significant because it addresses the shortcomings of previous datasets, helping to advance the capabilities of text-to-video models. By providing better training data, this dataset can lead to more accurate and realistic video generation, which is important for applications in entertainment, education, and beyond.
Abstract
The quality of video-text pairs fundamentally determines the upper bound of text-to-video models. Currently, the datasets used for training these models suffer from significant shortcomings, including low temporal consistency, poor-quality captions, substandard video quality, and imbalanced data distribution. The prevailing video curation process, which depends on image models for tagging and manual rule-based curation, leads to a high computational load and leaves behind unclean data. As a result, there is a lack of appropriate training datasets for text-to-video models. To address this problem, we present VidGen-1M, a superior training dataset for text-to-video models. Produced through a coarse-to-fine curation strategy, this dataset guarantees high-quality videos and detailed captions with excellent temporal consistency. When used to train the video generation model, this dataset has led to experimental results that surpass those obtained with other models.