VisDoM: Multi-Document QA with Visually Rich Elements Using Multimodal Retrieval-Augmented Generation
Manan Suri, Puneet Mathur, Franck Dernoncourt, Kanika Goswami, Ryan A. Rossi, Dinesh Manocha
2024-12-18
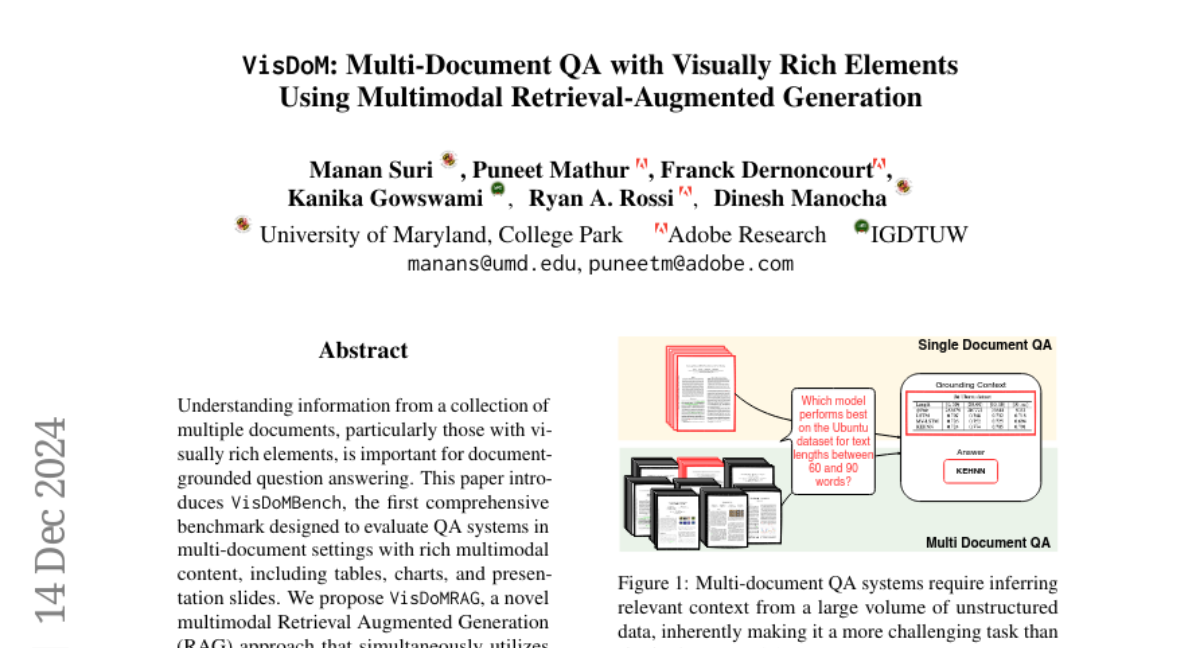
Summary
This paper talks about VisDoM, a new method for answering questions based on multiple documents that include rich visual elements like tables and charts, using a special approach called Retrieval-Augmented Generation (RAG).
What's the problem?
When trying to answer questions based on several documents, especially those with lots of visuals, current systems often struggle. They may not effectively combine information from text and images, leading to incomplete or incorrect answers. This is a big issue because many real-world documents contain important visual data that needs to be understood alongside the text.
What's the solution?
VisDoM introduces a benchmark called VisDoMBench to evaluate how well question-answering systems can handle these multi-document scenarios. It uses a novel approach called VisDoMRAG that combines both visual and text-based retrieval methods. This means it can pull relevant information from both types of content and reason through them together. A key feature of VisDoMRAG is its ability to ensure that the reasoning across different types of information is consistent, which helps produce clearer and more accurate answers.
Why it matters?
This research is important because it improves how AI systems can understand and answer complex questions that involve both text and visuals. By enhancing the ability to process multi-document information, VisDoM can help in various fields like education, finance, and research, where understanding detailed information from multiple sources is crucial.
Abstract
Understanding information from a collection of multiple documents, particularly those with visually rich elements, is important for document-grounded question answering. This paper introduces VisDoMBench, the first comprehensive benchmark designed to evaluate QA systems in multi-document settings with rich multimodal content, including tables, charts, and presentation slides. We propose VisDoMRAG, a novel multimodal Retrieval Augmented Generation (RAG) approach that simultaneously utilizes visual and textual RAG, combining robust visual retrieval capabilities with sophisticated linguistic reasoning. VisDoMRAG employs a multi-step reasoning process encompassing evidence curation and chain-of-thought reasoning for concurrent textual and visual RAG pipelines. A key novelty of VisDoMRAG is its consistency-constrained modality fusion mechanism, which aligns the reasoning processes across modalities at inference time to produce a coherent final answer. This leads to enhanced accuracy in scenarios where critical information is distributed across modalities and improved answer verifiability through implicit context attribution. Through extensive experiments involving open-source and proprietary large language models, we benchmark state-of-the-art document QA methods on VisDoMBench. Extensive results show that VisDoMRAG outperforms unimodal and long-context LLM baselines for end-to-end multimodal document QA by 12-20%.