Vision Search Assistant: Empower Vision-Language Models as Multimodal Search Engines
Zhixin Zhang, Yiyuan Zhang, Xiaohan Ding, Xiangyu Yue
2024-10-29
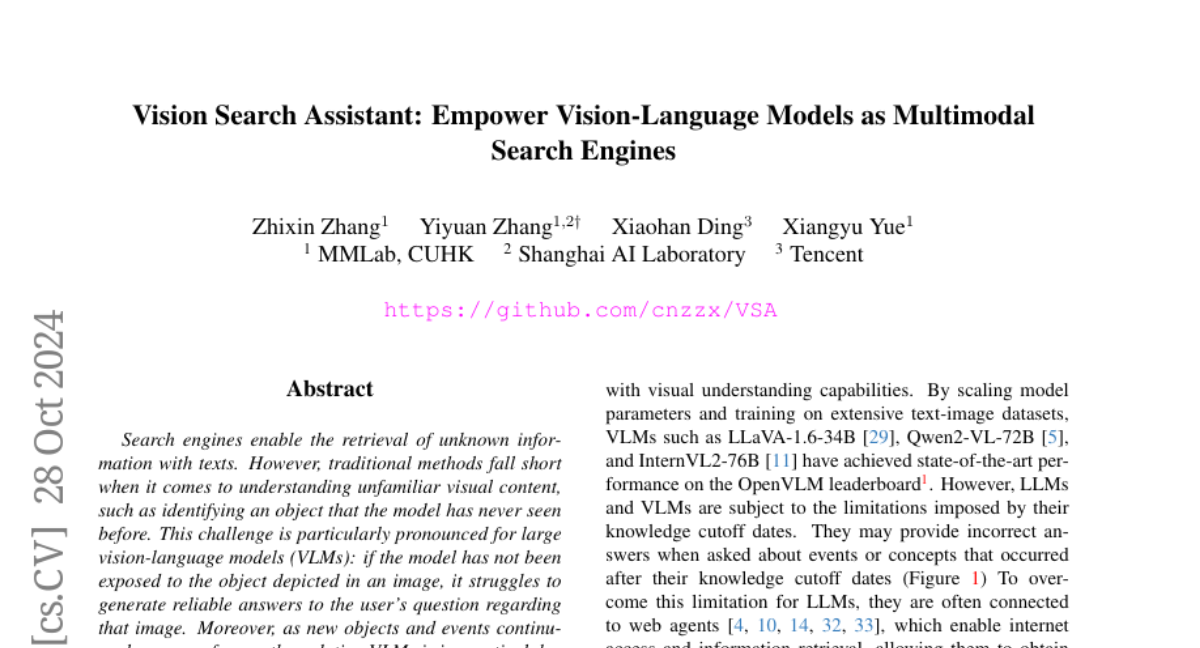
Summary
This paper presents the Vision Search Assistant, a new framework that enhances vision-language models (VLMs) to better understand and respond to images by using real-time information from the web.
What's the problem?
Traditional search engines and VLMs often struggle to identify and understand unfamiliar visual content, such as objects they have never encountered before. This is a significant issue because as new objects and events appear, it's impractical to constantly update these models due to the heavy computational resources required. Consequently, when faced with new images, these models may provide unreliable answers to user queries.
What's the solution?
To solve this problem, the authors propose the Vision Search Assistant, which allows VLMs to collaborate with web agents. This collaboration enables the model to access up-to-date information from the internet while leveraging its visual understanding capabilities. By combining visual and textual information in real-time, this framework allows VLMs to provide accurate responses even when dealing with novel images. The authors conducted extensive experiments showing that the Vision Search Assistant outperforms existing models in both open-set (unknown objects) and closed-set (known objects) question-answering tasks.
Why it matters?
This research is important because it significantly improves how AI systems can interact with and understand visual information. By enabling VLMs to access real-time data, the Vision Search Assistant can enhance applications in areas like education, customer service, and content creation, where accurate image interpretation is crucial.
Abstract
Search engines enable the retrieval of unknown information with texts. However, traditional methods fall short when it comes to understanding unfamiliar visual content, such as identifying an object that the model has never seen before. This challenge is particularly pronounced for large vision-language models (VLMs): if the model has not been exposed to the object depicted in an image, it struggles to generate reliable answers to the user's question regarding that image. Moreover, as new objects and events continuously emerge, frequently updating VLMs is impractical due to heavy computational burdens. To address this limitation, we propose Vision Search Assistant, a novel framework that facilitates collaboration between VLMs and web agents. This approach leverages VLMs' visual understanding capabilities and web agents' real-time information access to perform open-world Retrieval-Augmented Generation via the web. By integrating visual and textual representations through this collaboration, the model can provide informed responses even when the image is novel to the system. Extensive experiments conducted on both open-set and closed-set QA benchmarks demonstrate that the Vision Search Assistant significantly outperforms the other models and can be widely applied to existing VLMs.