Visual Riddles: a Commonsense and World Knowledge Challenge for Large Vision and Language Models
Nitzan Bitton-Guetta, Aviv Slobodkin, Aviya Maimon, Eliya Habba, Royi Rassin, Yonatan Bitton, Idan Szpektor, Amir Globerson, Yuval Elovici
2024-07-30
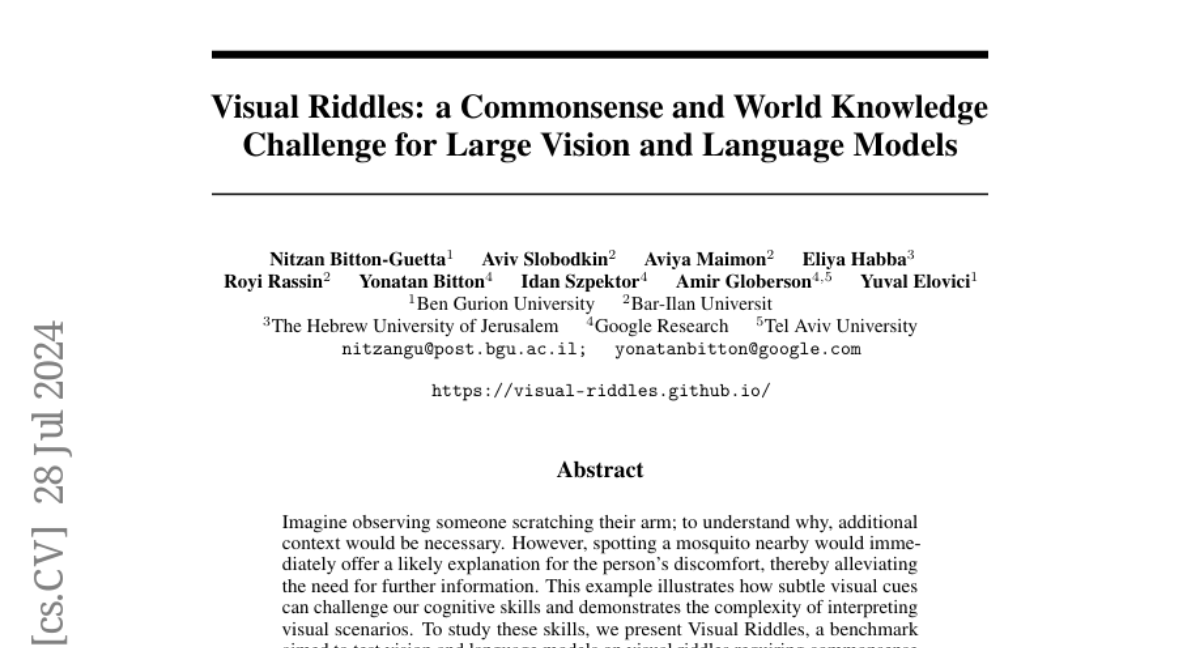
Summary
This paper introduces Visual Riddles, a benchmark designed to test how well vision and language models can understand complex visual scenarios that require commonsense and world knowledge. It includes 400 unique visual riddles that challenge AI models to interpret subtle visual cues.
What's the problem?
Understanding visual information often requires context and commonsense reasoning. Current AI models struggle with this because they can miss important details in images and lack the ability to connect visual clues with real-world knowledge. This can lead to poor performance when interpreting images, especially in ambiguous situations.
What's the solution?
To address these challenges, the authors created Visual Riddles, which consists of 400 visual riddles that include an image, a question, and a correct answer. Each riddle is designed to test the model's ability to use commonsense knowledge to solve problems based on visual cues. The benchmark allows for automatic evaluation of model performance, making it easier to assess how well different AI systems understand these riddles. Human evaluations showed that current models perform significantly worse than humans, achieving only about 40% accuracy compared to human accuracy of 82%.
Why it matters?
This research is important because it highlights the gaps in AI understanding of complex visual information and provides a new way to evaluate and improve the capabilities of vision and language models. By focusing on commonsense reasoning in visual contexts, Visual Riddles can help advance AI technology, making it more effective in real-world applications where interpreting images accurately is crucial.
Abstract
Imagine observing someone scratching their arm; to understand why, additional context would be necessary. However, spotting a mosquito nearby would immediately offer a likely explanation for the person's discomfort, thereby alleviating the need for further information. This example illustrates how subtle visual cues can challenge our cognitive skills and demonstrates the complexity of interpreting visual scenarios. To study these skills, we present Visual Riddles, a benchmark aimed to test vision and language models on visual riddles requiring commonsense and world knowledge. The benchmark comprises 400 visual riddles, each featuring a unique image created by a variety of text-to-image models, question, ground-truth answer, textual hint, and attribution. Human evaluation reveals that existing models lag significantly behind human performance, which is at 82\% accuracy, with Gemini-Pro-1.5 leading with 40\% accuracy. Our benchmark comes with automatic evaluation tasks to make assessment scalable. These findings underscore the potential of Visual Riddles as a valuable resource for enhancing vision and language models' capabilities in interpreting complex visual scenarios.