VisualAgentBench: Towards Large Multimodal Models as Visual Foundation Agents
Xiao Liu, Tianjie Zhang, Yu Gu, Iat Long Iong, Yifan Xu, Xixuan Song, Shudan Zhang, Hanyu Lai, Xinyi Liu, Hanlin Zhao, Jiadai Sun, Xinyue Yang, Yu Yang, Zehan Qi, Shuntian Yao, Xueqiao Sun, Siyi Cheng, Qinkai Zheng, Hao Yu, Hanchen Zhang, Wenyi Hong, Ming Ding
2024-08-13
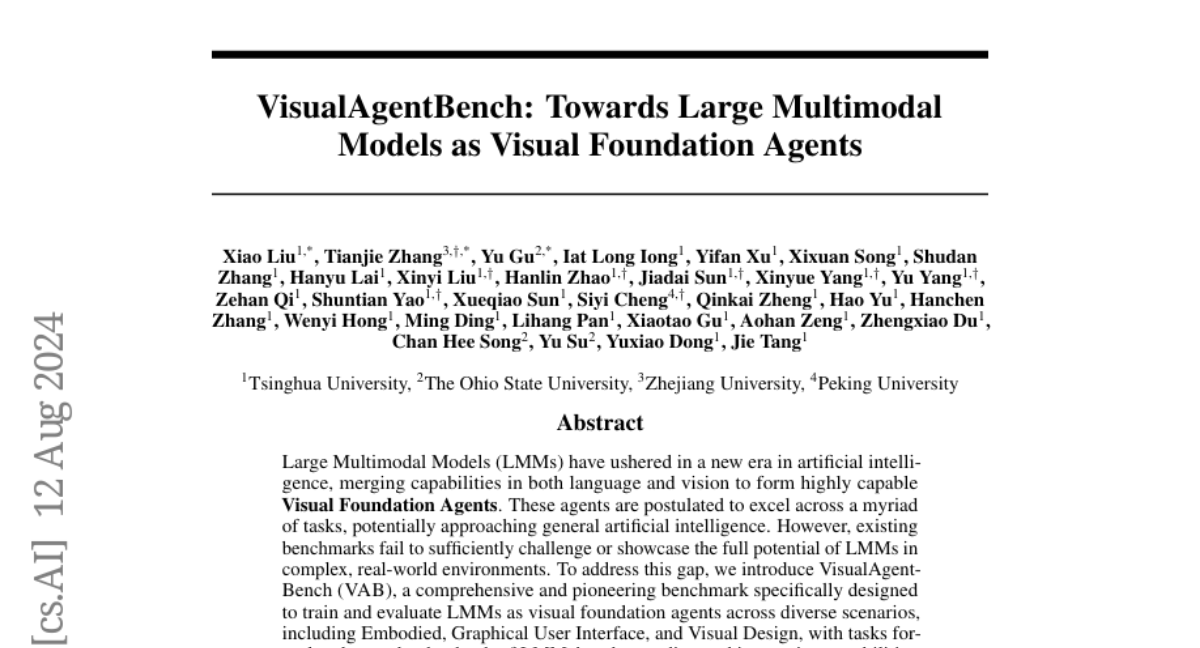
Summary
This paper introduces VisualAgentBench (VAB), a new benchmark designed to test and improve large multimodal models (LMMs) that combine language and vision skills, helping them perform better in real-world tasks.
What's the problem?
Current benchmarks do not fully challenge or demonstrate the capabilities of large multimodal models in complex environments. This means that these models might not be trained effectively to handle real-world tasks that require both understanding language and visual information.
What's the solution?
The authors created VisualAgentBench, a comprehensive set of tests specifically designed for LMMs. VAB includes various scenarios like interacting with graphical user interfaces and performing visual design tasks. It also uses advanced training techniques, including human demonstrations and program-based solvers, to help these models learn better. By rigorously testing nine different LMM APIs and eight open models, the researchers were able to show how well these models can perform across different tasks.
Why it matters?
This research is important because it sets a new standard for evaluating multimodal AI models, which are crucial for developing more advanced artificial intelligence systems. By improving how these models are trained and tested, we can move closer to achieving general artificial intelligence that can understand and interact with the world as humans do.
Abstract
Large Multimodal Models (LMMs) have ushered in a new era in artificial intelligence, merging capabilities in both language and vision to form highly capable Visual Foundation Agents. These agents are postulated to excel across a myriad of tasks, potentially approaching general artificial intelligence. However, existing benchmarks fail to sufficiently challenge or showcase the full potential of LMMs in complex, real-world environments. To address this gap, we introduce VisualAgentBench (VAB), a comprehensive and pioneering benchmark specifically designed to train and evaluate LMMs as visual foundation agents across diverse scenarios, including Embodied, Graphical User Interface, and Visual Design, with tasks formulated to probe the depth of LMMs' understanding and interaction capabilities. Through rigorous testing across nine proprietary LMM APIs and eight open models, we demonstrate the considerable yet still developing agent capabilities of these models. Additionally, VAB constructs a trajectory training set constructed through hybrid methods including Program-based Solvers, LMM Agent Bootstrapping, and Human Demonstrations, promoting substantial performance improvements in LMMs through behavior cloning. Our work not only aims to benchmark existing models but also provides a solid foundation for future development into visual foundation agents. Code, train \& test data, and part of fine-tuned open LMMs are available at https://github.com/THUDM/VisualAgentBench.