VisualCloze: A Universal Image Generation Framework via Visual In-Context Learning
Zhong-Yu Li, Ruoyi Du, Juncheng Yan, Le Zhuo, Zhen Li, Peng Gao, Zhanyu Ma, Ming-Ming Cheng
2025-04-11
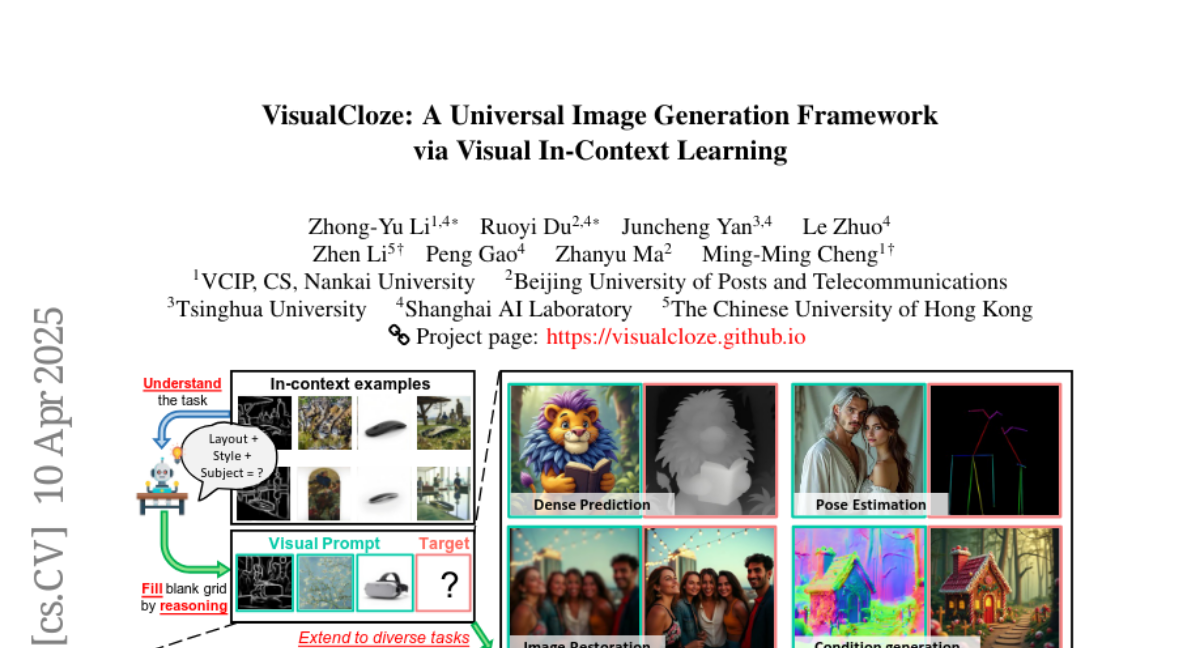
Summary
This paper talks about VisualCloze, a tool that helps AI generate all kinds of images by learning from example pictures, like teaching it to draw by showing it similar art instead of giving written instructions.
What's the problem?
Current AI image tools are built for specific tasks (like drawing cats or landscapes) and struggle to handle new requests or combine skills without retraining, plus they rely too much on text instructions that can be unclear.
What's the solution?
VisualCloze uses example images to teach the AI what to do, builds a smart dataset (Graph200K) that connects related tasks like puzzles, and works with existing image-filling models to handle new challenges without rebuilding everything from scratch.
Why it matters?
This lets creators make custom images faster for things like ads or games, helps AI learn new styles or tasks more easily, and reduces the need for expensive retraining of models.
Abstract
Recent progress in diffusion models significantly advances various image generation tasks. However, the current mainstream approach remains focused on building task-specific models, which have limited efficiency when supporting a wide range of different needs. While universal models attempt to address this limitation, they face critical challenges, including generalizable task instruction, appropriate task distributions, and unified architectural design. To tackle these challenges, we propose VisualCloze, a universal image generation framework, which supports a wide range of in-domain tasks, generalization to unseen ones, unseen unification of multiple tasks, and reverse generation. Unlike existing methods that rely on language-based task instruction, leading to task ambiguity and weak generalization, we integrate visual in-context learning, allowing models to identify tasks from visual demonstrations. Meanwhile, the inherent sparsity of visual task distributions hampers the learning of transferable knowledge across tasks. To this end, we introduce Graph200K, a graph-structured dataset that establishes various interrelated tasks, enhancing task density and transferable knowledge. Furthermore, we uncover that our unified image generation formulation shared a consistent objective with image infilling, enabling us to leverage the strong generative priors of pre-trained infilling models without modifying the architectures.