VisualLens: Personalization through Visual History
Wang Bill Zhu, Deqing Fu, Kai Sun, Yi Lu, Zhaojiang Lin, Seungwhan Moon, Kanika Narang, Mustafa Canim, Yue Liu, Anuj Kumar, Xin Luna Dong
2024-11-26
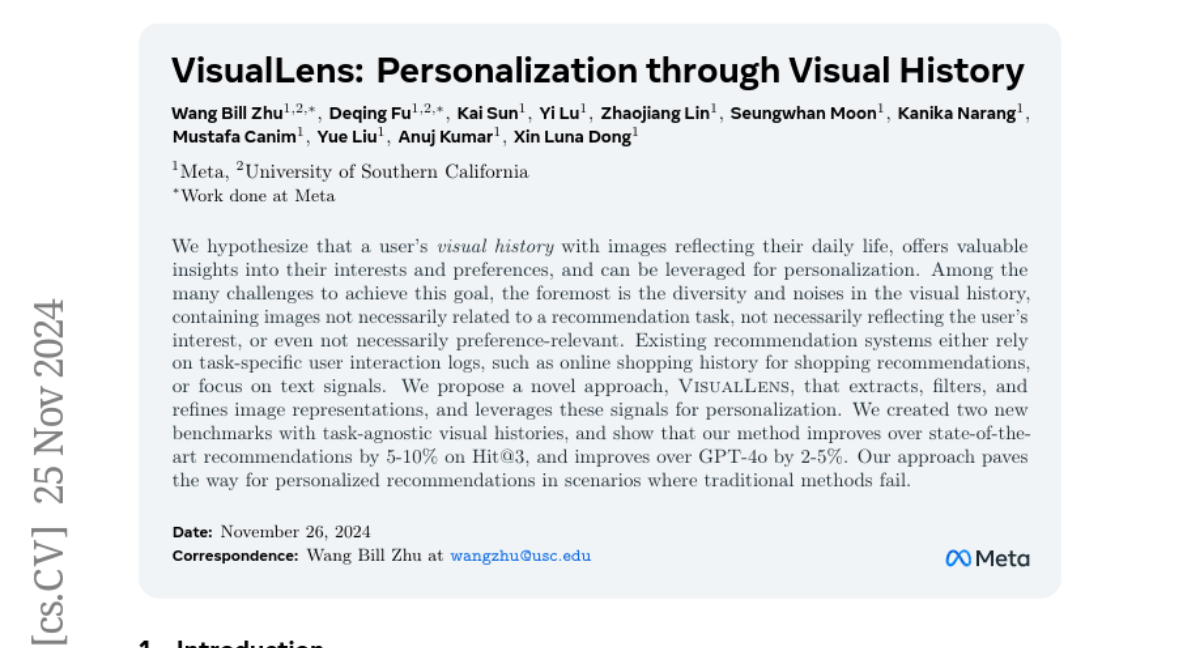
Summary
This paper introduces VisualLens, a new method for personalizing recommendations based on a user's visual history, such as photos they have taken or shared.
What's the problem?
Many recommendation systems struggle to provide personalized suggestions because they often rely on limited types of data, like shopping history or text descriptions. Users' visual histories can contain a lot of irrelevant images that do not accurately reflect their interests, making it hard to generate useful recommendations.
What's the solution?
VisualLens addresses this problem by extracting and refining information from a user's visual history. It filters out irrelevant images and focuses on the most relevant ones to understand the user's preferences better. The method uses two new benchmarks to evaluate its effectiveness and shows improvements over existing recommendation systems by 5-10% in accuracy. By leveraging both visual and textual information from the images, VisualLens creates a more accurate profile of what users like, leading to better recommendations.
Why it matters?
This research is important because it enhances how recommendation systems work by using visual data, which is often more personal and meaningful than traditional data sources. By improving personalization in recommendations, VisualLens can lead to better user experiences in various applications, such as online shopping, social media, and content discovery.
Abstract
We hypothesize that a user's visual history with images reflecting their daily life, offers valuable insights into their interests and preferences, and can be leveraged for personalization. Among the many challenges to achieve this goal, the foremost is the diversity and noises in the visual history, containing images not necessarily related to a recommendation task, not necessarily reflecting the user's interest, or even not necessarily preference-relevant. Existing recommendation systems either rely on task-specific user interaction logs, such as online shopping history for shopping recommendations, or focus on text signals. We propose a novel approach, VisualLens, that extracts, filters, and refines image representations, and leverages these signals for personalization. We created two new benchmarks with task-agnostic visual histories, and show that our method improves over state-of-the-art recommendations by 5-10% on Hit@3, and improves over GPT-4o by 2-5%. Our approach paves the way for personalized recommendations in scenarios where traditional methods fail.