VLM4Bio: A Benchmark Dataset to Evaluate Pretrained Vision-Language Models for Trait Discovery from Biological Images
M. Maruf, Arka Daw, Kazi Sajeed Mehrab, Harish Babu Manogaran, Abhilash Neog, Medha Sawhney, Mridul Khurana, James P. Balhoff, Yasin Bakis, Bahadir Altintas, Matthew J. Thompson, Elizabeth G. Campolongo, Josef C. Uyeda, Hilmar Lapp, Henry L. Bart, Paula M. Mabee, Yu Su, Wei-Lun Chao, Charles Stewart, Tanya Berger-Wolf, Wasila Dahdul, Anuj Karpatne
2024-09-02
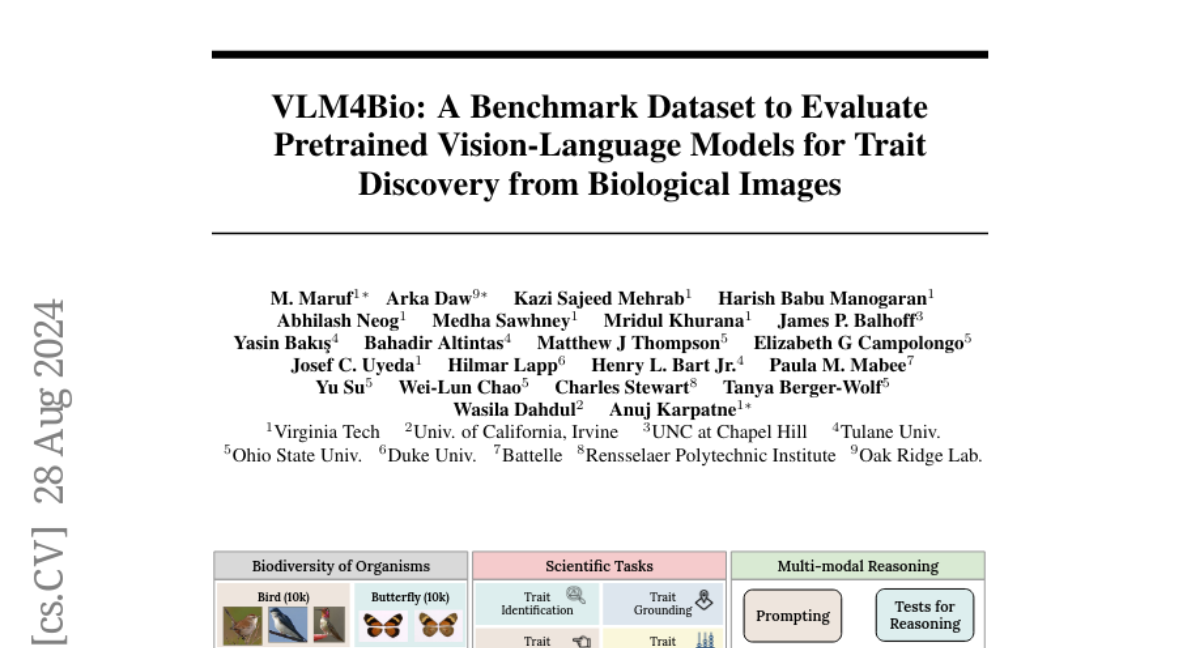
Summary
This paper talks about VLM4Bio, a new dataset created to evaluate how well pretrained vision-language models can help scientists answer questions about biological images.
What's the problem?
As scientists study biodiversity, they often rely on images to gather information about different organisms. However, existing models that combine image and text understanding (called vision-language models or VLMs) may not perform well without additional training, which can limit their usefulness in biology.
What's the solution?
The authors introduce the VLM4Bio dataset, which includes 469,000 question-answer pairs related to 30,000 images of fishes, birds, and butterflies. They evaluate 12 state-of-the-art VLMs using this dataset to see if they can accurately answer biologically relevant questions without needing extra training. The study also examines how different prompting techniques affect the models' performance.
Why it matters?
This research is important because it helps improve our understanding of how VLMs can be used in biological research. By evaluating these models with a large and relevant dataset, it paves the way for better tools that can assist scientists in making discoveries about biodiversity and ecosystem health.
Abstract
Images are increasingly becoming the currency for documenting biodiversity on the planet, providing novel opportunities for accelerating scientific discoveries in the field of organismal biology, especially with the advent of large vision-language models (VLMs). We ask if pre-trained VLMs can aid scientists in answering a range of biologically relevant questions without any additional fine-tuning. In this paper, we evaluate the effectiveness of 12 state-of-the-art (SOTA) VLMs in the field of organismal biology using a novel dataset, VLM4Bio, consisting of 469K question-answer pairs involving 30K images from three groups of organisms: fishes, birds, and butterflies, covering five biologically relevant tasks. We also explore the effects of applying prompting techniques and tests for reasoning hallucination on the performance of VLMs, shedding new light on the capabilities of current SOTA VLMs in answering biologically relevant questions using images. The code and datasets for running all the analyses reported in this paper can be found at https://github.com/sammarfy/VLM4Bio.