VMAS: Video-to-Music Generation via Semantic Alignment in Web Music Videos
Yan-Bo Lin, Yu Tian, Linjie Yang, Gedas Bertasius, Heng Wang
2024-09-12
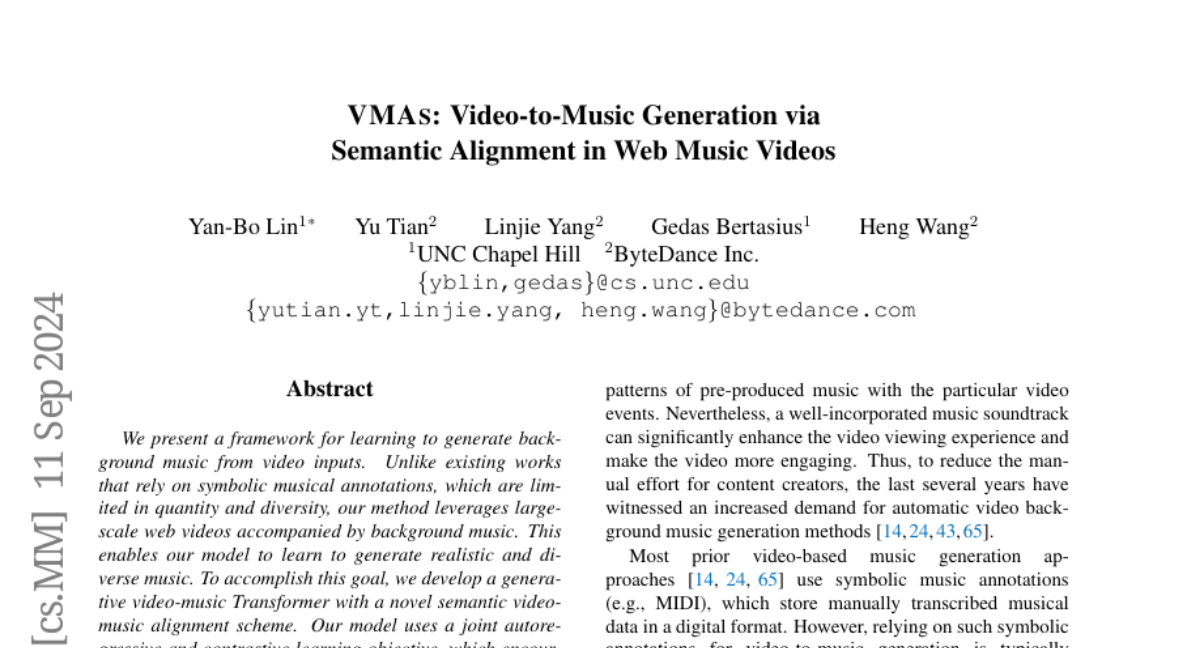
Summary
This paper talks about VMAS, a new framework that generates background music from videos by aligning the music with the content of the video.
What's the problem?
Existing methods for generating music from videos often rely on limited musical annotations, which can restrict the variety and quality of the music produced. This means that the generated music may not fit well with the video or may lack diversity.
What's the solution?
The authors developed VMAS, which uses a large dataset of web videos paired with background music to train a model that can create realistic and diverse music. They introduced a special alignment technique that connects the video content with the generated music, ensuring that the music matches what is happening in the video. Additionally, they designed a new video encoder to process videos with many frames efficiently. Their model was trained on a large dataset called DISCO-MV, which includes 2.2 million video-music pairs.
Why it matters?
This research is important because it improves how we can automatically generate background music for videos, making it more fitting and engaging. By leveraging a large amount of data and advanced techniques, VMAS can enhance the overall viewing experience in various applications, such as film production, gaming, and online content creation.
Abstract
We present a framework for learning to generate background music from video inputs. Unlike existing works that rely on symbolic musical annotations, which are limited in quantity and diversity, our method leverages large-scale web videos accompanied by background music. This enables our model to learn to generate realistic and diverse music. To accomplish this goal, we develop a generative video-music Transformer with a novel semantic video-music alignment scheme. Our model uses a joint autoregressive and contrastive learning objective, which encourages the generation of music aligned with high-level video content. We also introduce a novel video-beat alignment scheme to match the generated music beats with the low-level motions in the video. Lastly, to capture fine-grained visual cues in a video needed for realistic background music generation, we introduce a new temporal video encoder architecture, allowing us to efficiently process videos consisting of many densely sampled frames. We train our framework on our newly curated DISCO-MV dataset, consisting of 2.2M video-music samples, which is orders of magnitude larger than any prior datasets used for video music generation. Our method outperforms existing approaches on the DISCO-MV and MusicCaps datasets according to various music generation evaluation metrics, including human evaluation. Results are available at https://genjib.github.io/project_page/VMAs/index.html