VolDoGer: LLM-assisted Datasets for Domain Generalization in Vision-Language Tasks
Juhwan Choi, Junehyoung Kwon, JungMin Yun, Seunguk Yu, YoungBin Kim
2024-07-30
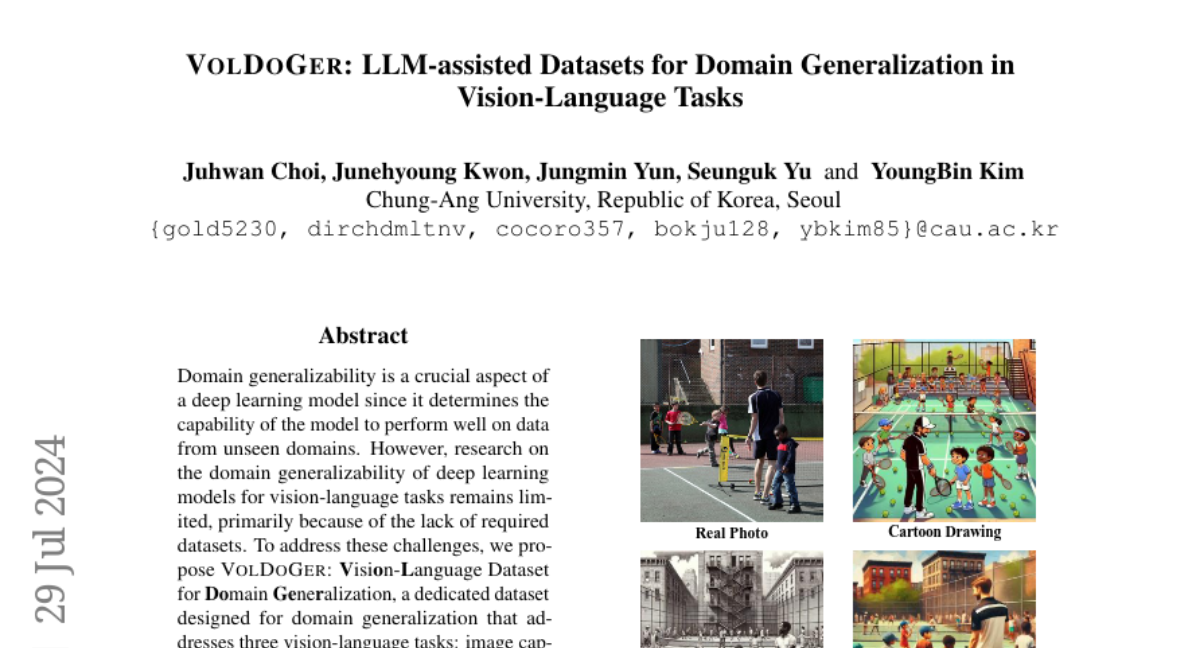
Summary
This paper introduces VolDoGer, a new dataset designed to help improve deep learning models' ability to work well with different types of data in vision-language tasks, such as image captioning and answering questions about images.
What's the problem?
Deep learning models need to perform well on data from various sources, but there aren't enough datasets that test their ability to generalize across different domains, especially for tasks that involve both images and text. This lack of diverse datasets makes it hard to train models effectively for real-world applications.
What's the solution?
To solve this problem, the authors created VolDoGer, a dataset specifically aimed at improving domain generalization for vision-language tasks. It includes data for three key tasks: image captioning, visual question answering, and visual entailment. The dataset was developed using techniques that involve large language models (LLMs) to annotate data, which reduces the need for human annotators and speeds up the process. The authors also tested various models on this dataset to evaluate their performance in handling different domains.
Why it matters?
This research is important because it provides a valuable resource for training and testing AI models in a way that reflects real-world scenarios. By improving how models can generalize across different types of data, VolDoGer can lead to better performance in applications like automated image description and interactive AI systems that understand both visual and textual information.
Abstract
Domain generalizability is a crucial aspect of a deep learning model since it determines the capability of the model to perform well on data from unseen domains. However, research on the domain generalizability of deep learning models for vision-language tasks remains limited, primarily because of the lack of required datasets. To address these challenges, we propose VolDoGer: Vision-Language Dataset for Domain Generalization, a dedicated dataset designed for domain generalization that addresses three vision-language tasks: image captioning, visual question answering, and visual entailment. We constructed VolDoGer by extending LLM-based data annotation techniques to vision-language tasks, thereby alleviating the burden of recruiting human annotators. We evaluated the domain generalizability of various models, ranging from fine-tuned models to a recent multimodal large language model, through VolDoGer.