VQ4DiT: Efficient Post-Training Vector Quantization for Diffusion Transformers
Juncan Deng, Shuaiting Li, Zeyu Wang, Hong Gu, Kedong Xu, Kejie Huang
2024-09-02
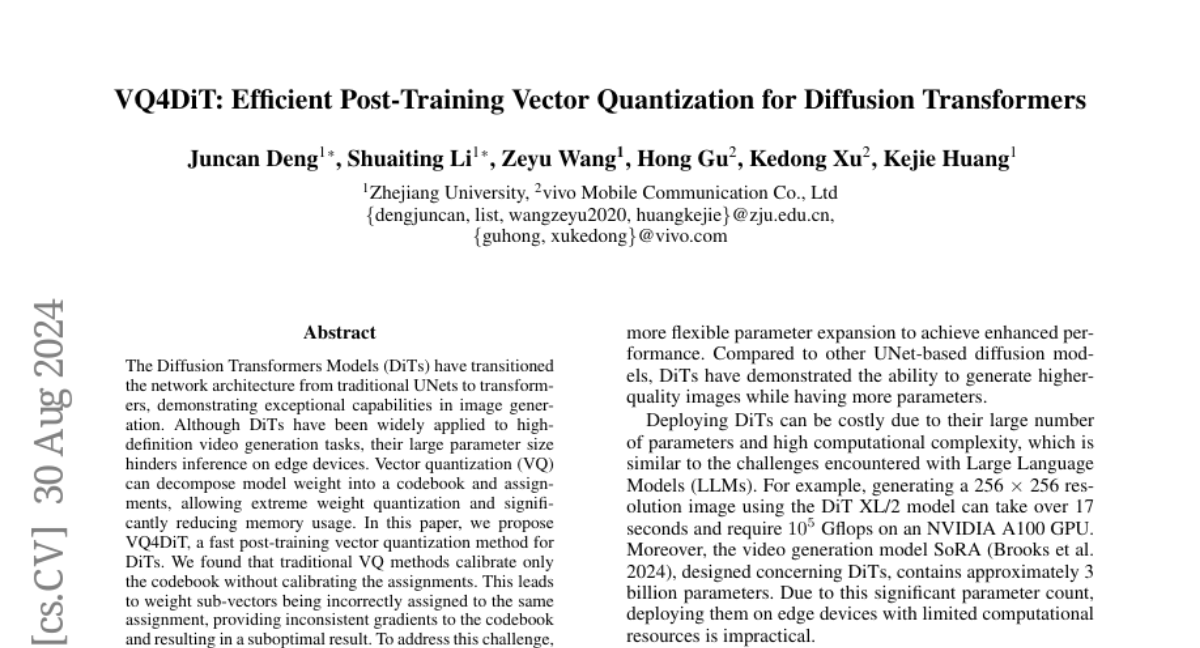
Summary
This paper talks about VQ4DiT, a new method for improving the efficiency of Diffusion Transformers, which are used for generating images.
What's the problem?
Diffusion Transformers (DiTs) are powerful tools for creating images, but they have a large number of parameters (the parts of the model that learn from data), making them difficult to run on smaller devices like smartphones or tablets. This limits their use in everyday applications.
What's the solution?
VQ4DiT introduces a technique called post-training vector quantization, which simplifies the model by breaking it down into smaller, more manageable pieces. It improves how the model assigns weights to these pieces, ensuring that they work together effectively. This method allows the model to be significantly smaller while still maintaining high-quality image generation capabilities.
Why it matters?
This research is important because it makes advanced image generation technology more accessible by allowing it to run on devices with less computing power. This could lead to more widespread use of image generation in apps and services that people use every day.
Abstract
The Diffusion Transformers Models (DiTs) have transitioned the network architecture from traditional UNets to transformers, demonstrating exceptional capabilities in image generation. Although DiTs have been widely applied to high-definition video generation tasks, their large parameter size hinders inference on edge devices. Vector quantization (VQ) can decompose model weight into a codebook and assignments, allowing extreme weight quantization and significantly reducing memory usage. In this paper, we propose VQ4DiT, a fast post-training vector quantization method for DiTs. We found that traditional VQ methods calibrate only the codebook without calibrating the assignments. This leads to weight sub-vectors being incorrectly assigned to the same assignment, providing inconsistent gradients to the codebook and resulting in a suboptimal result. To address this challenge, VQ4DiT calculates the candidate assignment set for each weight sub-vector based on Euclidean distance and reconstructs the sub-vector based on the weighted average. Then, using the zero-data and block-wise calibration method, the optimal assignment from the set is efficiently selected while calibrating the codebook. VQ4DiT quantizes a DiT XL/2 model on a single NVIDIA A100 GPU within 20 minutes to 5 hours depending on the different quantization settings. Experiments show that VQ4DiT establishes a new state-of-the-art in model size and performance trade-offs, quantizing weights to 2-bit precision while retaining acceptable image generation quality.