Watermark Anything with Localized Messages
Tom Sander, Pierre Fernandez, Alain Durmus, Teddy Furon, Matthijs Douze
2024-11-12
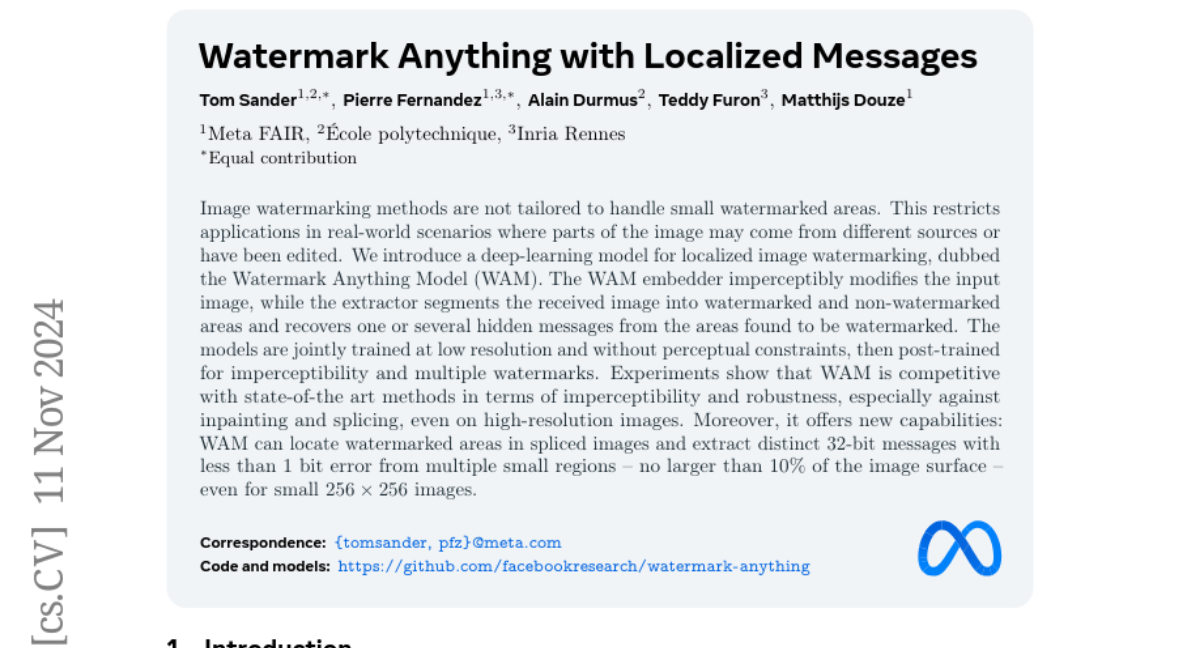
Summary
This paper introduces the Watermark Anything Model (WAM), a new deep-learning approach for adding and detecting watermarks in specific areas of images, making it easier to handle images that have been edited or come from different sources.
What's the problem?
Traditional image watermarking methods struggle with small areas that need to be watermarked. This limitation can be a problem in real-world situations where images may have been altered or combined from different sources. Existing methods often fail to accurately embed and extract watermarks in these cases, leading to challenges in ensuring the integrity and ownership of images.
What's the solution?
WAM addresses this issue by using a two-part system: an embedder that subtly adds watermarks to images and an extractor that identifies and retrieves these watermarks from the images. The model is trained to work well even when watermarked areas are small (up to 10% of the image surface) and can handle high-resolution images effectively. WAM also has the ability to locate watermarked areas in edited images and extract multiple hidden messages with high accuracy, even for small images.
Why it matters?
This research is important because it improves how watermarks can be added and detected in images, especially in complex scenarios where images are edited or combined. By enhancing watermarking techniques, WAM helps protect intellectual property and ensures that the original creators of images can maintain control over their work, which is crucial in fields like photography, digital art, and media.
Abstract
Image watermarking methods are not tailored to handle small watermarked areas. This restricts applications in real-world scenarios where parts of the image may come from different sources or have been edited. We introduce a deep-learning model for localized image watermarking, dubbed the Watermark Anything Model (WAM). The WAM embedder imperceptibly modifies the input image, while the extractor segments the received image into watermarked and non-watermarked areas and recovers one or several hidden messages from the areas found to be watermarked. The models are jointly trained at low resolution and without perceptual constraints, then post-trained for imperceptibility and multiple watermarks. Experiments show that WAM is competitive with state-of-the art methods in terms of imperceptibility and robustness, especially against inpainting and splicing, even on high-resolution images. Moreover, it offers new capabilities: WAM can locate watermarked areas in spliced images and extract distinct 32-bit messages with less than 1 bit error from multiple small regions - no larger than 10% of the image surface - even for small 256times 256 images.