We-Math: Does Your Large Multimodal Model Achieve Human-like Mathematical Reasoning?
Runqi Qiao, Qiuna Tan, Guanting Dong, Minhui Wu, Chong Sun, Xiaoshuai Song, Zhuoma GongQue, Shanglin Lei, Zhe Wei, Miaoxuan Zhang, Runfeng Qiao, Yifan Zhang, Xiao Zong, Yida Xu, Muxi Diao, Zhimin Bao, Chen Li, Honggang Zhang
2024-07-02
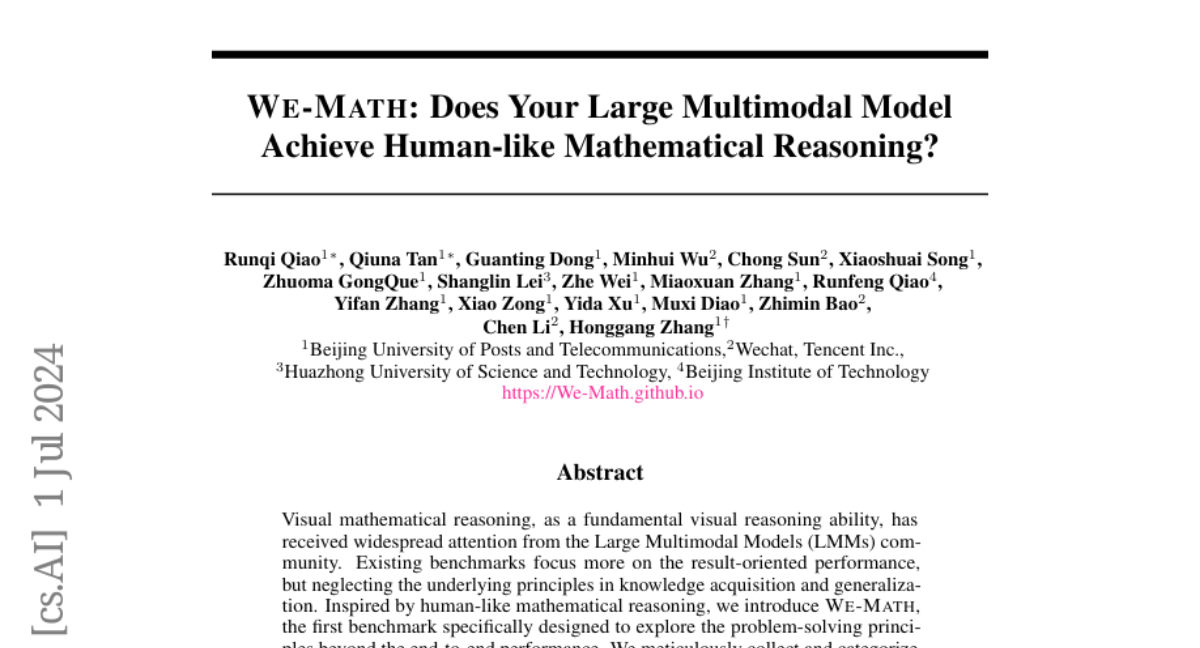
Summary
This paper talks about WE-MATH, a new benchmark designed to evaluate how well large multimodal models (LMMs) can reason through visual mathematical problems, similar to how humans do. It focuses on understanding the problem-solving processes of these models rather than just their final answers.
What's the problem?
Current benchmarks for evaluating LMMs, like MathVista and MathVerse, mainly look at whether the models can get the right answers but often ignore how they arrive at those answers. This means they don't assess whether the models truly understand the concepts involved in solving math problems. As a result, these models might perform well on some tasks but fail to grasp the underlying principles of mathematics.
What's the solution?
To address this issue, the authors created WE-MATH, which includes 6,500 visual math problems categorized into 67 different knowledge concepts. They break down complex problems into smaller sub-problems based on the knowledge needed to solve them. Additionally, they developed a four-dimensional metric to evaluate the models' performance in terms of Insufficient Knowledge (IK), Inadequate Generalization (IG), Complete Mastery (CM), and Rote Memorization (RM). This allows for a more detailed understanding of where the models struggle and how they can improve.
Why it matters?
This research is important because it sets a new standard for evaluating AI models in mathematical reasoning. By focusing on how these models solve problems rather than just whether they get the right answer, WE-MATH helps identify specific areas where AI can improve its understanding of math. This could lead to better educational tools and AI systems that can assist with learning and problem-solving in mathematics.
Abstract
Visual mathematical reasoning, as a fundamental visual reasoning ability, has received widespread attention from the Large Multimodal Models (LMMs) community. Existing benchmarks, such as MathVista and MathVerse, focus more on the result-oriented performance but neglect the underlying principles in knowledge acquisition and generalization. Inspired by human-like mathematical reasoning, we introduce WE-MATH, the first benchmark specifically designed to explore the problem-solving principles beyond end-to-end performance. We meticulously collect and categorize 6.5K visual math problems, spanning 67 hierarchical knowledge concepts and five layers of knowledge granularity. We decompose composite problems into sub-problems according to the required knowledge concepts and introduce a novel four-dimensional metric, namely Insufficient Knowledge (IK), Inadequate Generalization (IG), Complete Mastery (CM), and Rote Memorization (RM), to hierarchically assess inherent issues in LMMs' reasoning process. With WE-MATH, we conduct a thorough evaluation of existing LMMs in visual mathematical reasoning and reveal a negative correlation between solving steps and problem-specific performance. We confirm the IK issue of LMMs can be effectively improved via knowledge augmentation strategies. More notably, the primary challenge of GPT-4o has significantly transitioned from IK to IG, establishing it as the first LMM advancing towards the knowledge generalization stage. In contrast, other LMMs exhibit a marked inclination towards Rote Memorization - they correctly solve composite problems involving multiple knowledge concepts yet fail to answer sub-problems. We anticipate that WE-MATH will open new pathways for advancements in visual mathematical reasoning for LMMs. The WE-MATH data and evaluation code are available at https://github.com/We-Math/We-Math.