What Happened in LLMs Layers when Trained for Fast vs. Slow Thinking: A Gradient Perspective
Ming Li, Yanhong Li, Tianyi Zhou
2024-11-01
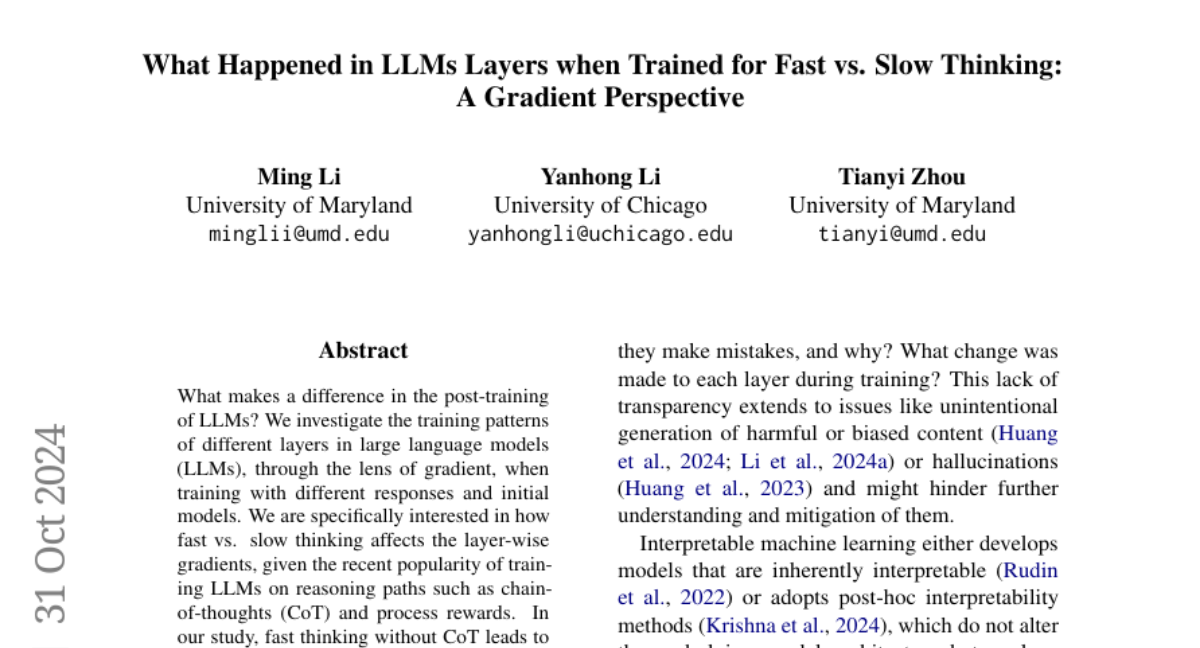
Summary
This paper explores how large language models (LLMs) behave differently when trained for fast thinking versus slow thinking, focusing on the patterns of learning in their layers.
What's the problem?
Understanding how LLMs learn is crucial for improving their performance, especially when they need to switch between quick responses and more thoughtful, detailed reasoning. However, there hasn't been much research into how these different types of thinking affect the learning process within the model's layers, particularly in terms of the gradients, which are indicators of how the model adjusts its learning.
What's the solution?
The authors investigate this by analyzing the gradients of different layers in LLMs when trained using fast thinking (giving immediate answers) versus slow thinking (using a step-by-step reasoning approach called chain-of-thought). They find that fast thinking leads to larger and more erratic gradients, indicating instability, while slow thinking results in more uniform gradients across layers, suggesting a steadier learning process. They also show that slow thinking helps the model distinguish correct answers from incorrect ones more effectively than fast thinking.
Why it matters?
This research is important because it provides insights into how LLMs can be trained more effectively. By understanding the differences between fast and slow thinking in terms of gradient behavior, developers can create better training strategies that enhance the models' reasoning abilities and overall performance. This could lead to improvements in various applications where accurate and reliable responses are critical.
Abstract
What makes a difference in the post-training of LLMs? We investigate the training patterns of different layers in large language models (LLMs), through the lens of gradient, when training with different responses and initial models. We are specifically interested in how fast vs. slow thinking affects the layer-wise gradients, given the recent popularity of training LLMs on reasoning paths such as chain-of-thoughts (CoT) and process rewards. In our study, fast thinking without CoT leads to larger gradients and larger differences of gradients across layers than slow thinking (Detailed CoT), indicating the learning stability brought by the latter. Moreover, pre-trained LLMs are less affected by the instability of fast thinking than instruction-tuned LLMs. Additionally, we study whether the gradient patterns can reflect the correctness of responses when training different LLMs using slow vs. fast thinking paths. The results show that the gradients of slow thinking can distinguish correct and irrelevant reasoning paths. As a comparison, we conduct similar gradient analyses on non-reasoning knowledge learning tasks, on which, however, trivially increasing the response length does not lead to similar behaviors of slow thinking. Our study strengthens fundamental understandings of LLM training and sheds novel insights on its efficiency and stability, which pave the way towards building a generalizable System-2 agent. Our code, data, and gradient statistics can be found in: https://github.com/MingLiiii/Layer_Gradient.