Whisper-GPT: A Hybrid Representation Audio Large Language Model
Prateek Verma
2024-12-17
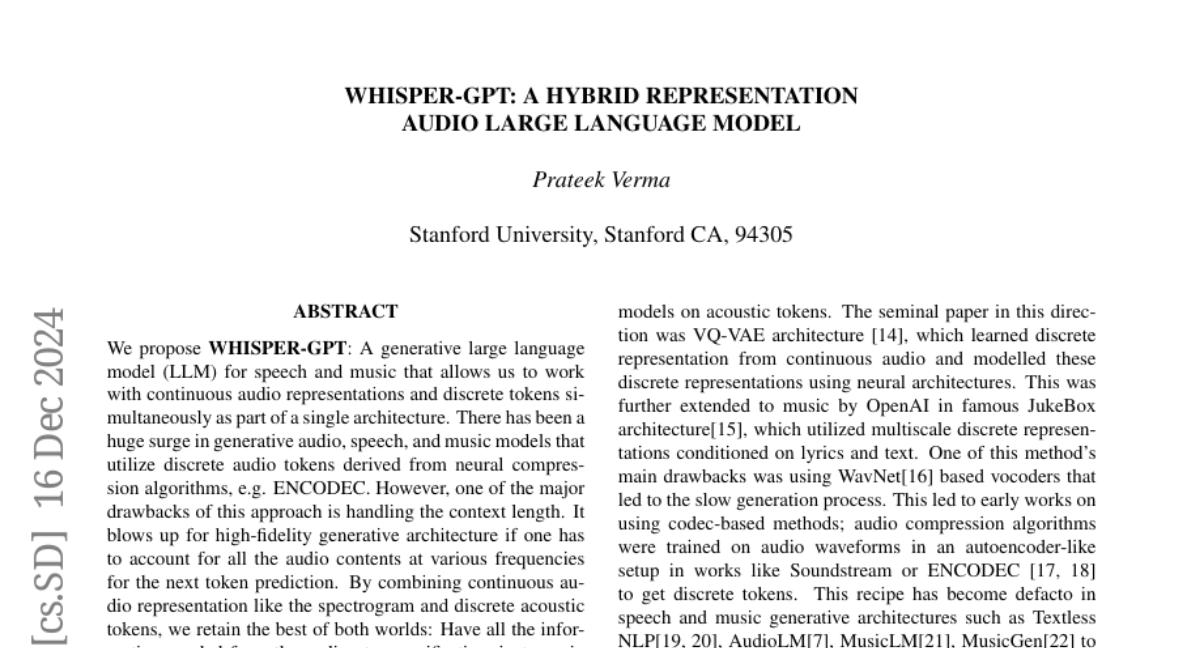
Summary
This paper introduces Whisper-GPT, a new audio model that combines continuous audio data and discrete tokens to improve how computers understand and generate speech and music.
What's the problem?
Many current audio models use discrete tokens derived from audio, which can make it hard to manage the length of the audio context. When predicting the next part of an audio sequence, these models struggle to keep track of all the details needed for high-quality sound, especially when dealing with complex audio like music or speech.
What's the solution?
Whisper-GPT solves this by integrating both continuous audio representations (like spectrograms) and discrete tokens in a single model. This allows the model to capture detailed information about the audio at specific moments while still being able to predict future sounds effectively. By doing this, it improves performance in generating and understanding audio content, resulting in better overall quality.
Why it matters?
This research is significant because it enhances how machines can generate and interpret audio, which is crucial for applications in speech recognition, music generation, and more. By improving the way computers handle audio data, Whisper-GPT can lead to advancements in technology that require high-quality sound processing, such as virtual assistants, music apps, and language learning tools.
Abstract
We propose WHISPER-GPT: A generative large language model (LLM) for speech and music that allows us to work with continuous audio representations and discrete tokens simultaneously as part of a single architecture. There has been a huge surge in generative audio, speech, and music models that utilize discrete audio tokens derived from neural compression algorithms, e.g. ENCODEC. However, one of the major drawbacks of this approach is handling the context length. It blows up for high-fidelity generative architecture if one has to account for all the audio contents at various frequencies for the next token prediction. By combining continuous audio representation like the spectrogram and discrete acoustic tokens, we retain the best of both worlds: Have all the information needed from the audio at a specific time instance in a single token, yet allow LLM to predict the future token to allow for sampling and other benefits discrete space provides. We show how our architecture improves the perplexity and negative log-likelihood scores for the next token prediction compared to a token-based LLM for speech and music.