Whiteboard-of-Thought: Thinking Step-by-Step Across Modalities
Sachit Menon, Richard Zemel, Carl Vondrick
2024-06-21
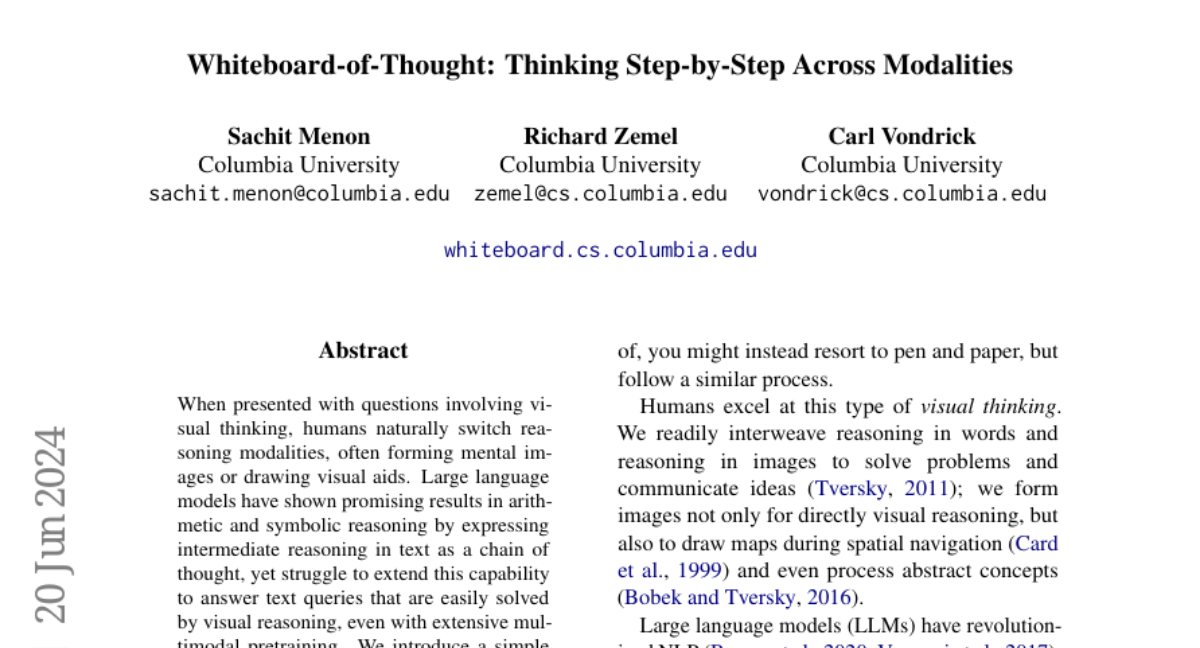
Summary
This paper introduces a new method called Whiteboard-of-Thought (WoT) prompting, which helps large language models (LLMs) improve their ability to solve visual reasoning problems by allowing them to create and use images during the reasoning process.
What's the problem?
While LLMs have been successful in tasks that require logical thinking and arithmetic, they often struggle with questions that involve visual reasoning. Humans naturally use images or drawings to help solve problems that require understanding space or visuals, but current LLMs do not effectively use visual aids, leading to poor performance on these types of tasks.
What's the solution?
The researchers developed the Whiteboard-of-Thought method, which gives LLMs a metaphorical 'whiteboard' where they can draw out their reasoning steps as images. This is done by having the model generate code using libraries like Matplotlib and Turtle to create visuals. After creating these images, the model can analyze them and use the information to answer questions more effectively. This approach has shown impressive results, achieving up to 92% accuracy on challenging tasks where traditional methods failed.
Why it matters?
This research is important because it demonstrates a new way for AI models to enhance their problem-solving abilities by incorporating visual thinking. By allowing models to create and process images, we can improve their performance on complex tasks that require understanding visual information, which has applications in fields like education, gaming, and robotics.
Abstract
When presented with questions involving visual thinking, humans naturally switch reasoning modalities, often forming mental images or drawing visual aids. Large language models have shown promising results in arithmetic and symbolic reasoning by expressing intermediate reasoning in text as a chain of thought, yet struggle to extend this capability to answer text queries that are easily solved by visual reasoning, even with extensive multimodal pretraining. We introduce a simple method, whiteboard-of-thought prompting, to unlock the visual reasoning capabilities of multimodal large language models across modalities. Whiteboard-of-thought prompting provides multimodal large language models with a metaphorical `whiteboard' to draw out reasoning steps as images, then returns these images back to the model for further processing. We find this can be accomplished with no demonstrations or specialized modules, instead leveraging models' existing ability to write code with libraries such as Matplotlib and Turtle. This simple approach shows state-of-the-art results on four difficult natural language tasks that involve visual and spatial reasoning. We identify multiple settings where GPT-4o using chain-of-thought fails dramatically, including more than one where it achieves 0% accuracy, while whiteboard-of-thought enables up to 92% accuracy in these same settings. We present a detailed exploration of where the technique succeeds as well as its sources of error.