WildGaussians: 3D Gaussian Splatting in the Wild
Jonas Kulhanek, Songyou Peng, Zuzana Kukelova, Marc Pollefeys, Torsten Sattler
2024-07-13
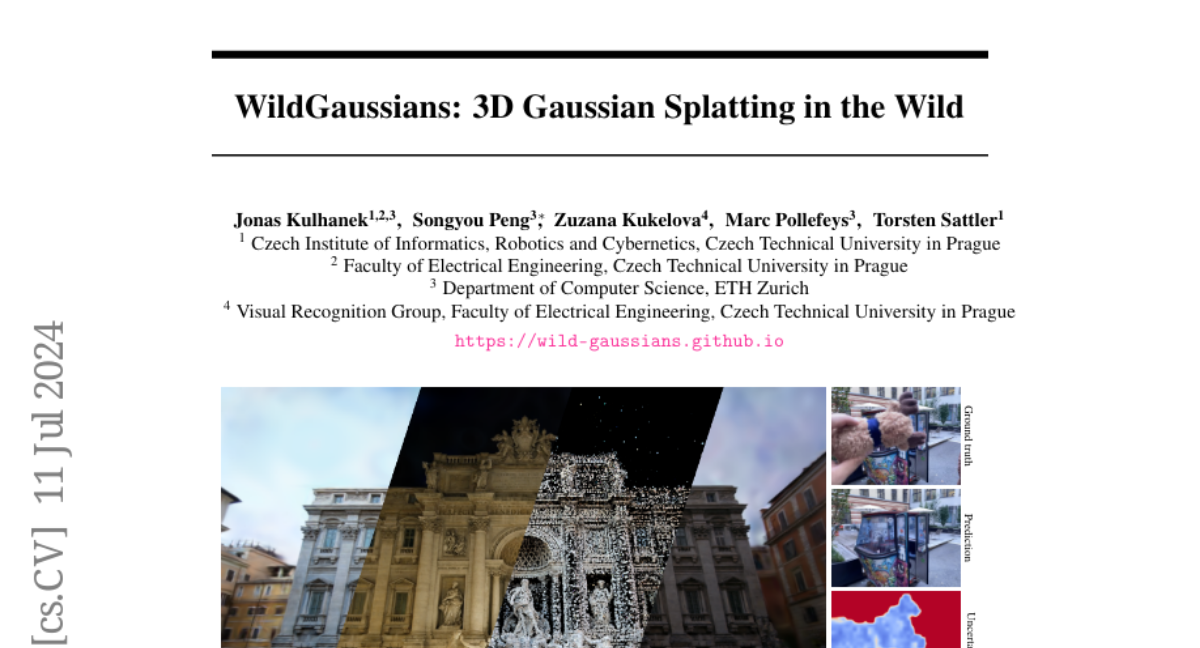
Summary
This paper introduces WildGaussians, a new method for creating 3D reconstructions of scenes from images taken in real-world conditions. It improves upon existing techniques by effectively handling challenges like changing lighting and moving objects.
What's the problem?
Current methods for 3D scene reconstruction, such as NeRFs and 3D Gaussian Splatting (3DGS), work well with controlled environments but struggle with real-world data. In the wild, images can have issues like occlusions (where objects block each other), dynamic elements (like people or cars moving), and varying light conditions, which make it hard to create accurate 3D models.
What's the solution?
WildGaussians addresses these problems by using advanced techniques to better manage occlusions and changes in appearance. It incorporates a new way to model how different lighting affects colors in images and uses robust features from a neural network called DINO to ignore parts of images that are blocked or not useful. This approach allows WildGaussians to produce high-quality 3D reconstructions quickly, matching the speed of existing methods while improving performance in challenging situations.
Why it matters?
This research is significant because it enhances the ability to create realistic 3D models from everyday photos taken in various conditions. By improving how we can reconstruct scenes from real-world data, WildGaussians has the potential to be used in fields like virtual reality, gaming, and urban planning, making it easier to visualize environments accurately.
Abstract
While the field of 3D scene reconstruction is dominated by NeRFs due to their photorealistic quality, 3D Gaussian Splatting (3DGS) has recently emerged, offering similar quality with real-time rendering speeds. However, both methods primarily excel with well-controlled 3D scenes, while in-the-wild data - characterized by occlusions, dynamic objects, and varying illumination - remains challenging. NeRFs can adapt to such conditions easily through per-image embedding vectors, but 3DGS struggles due to its explicit representation and lack of shared parameters. To address this, we introduce WildGaussians, a novel approach to handle occlusions and appearance changes with 3DGS. By leveraging robust DINO features and integrating an appearance modeling module within 3DGS, our method achieves state-of-the-art results. We demonstrate that WildGaussians matches the real-time rendering speed of 3DGS while surpassing both 3DGS and NeRF baselines in handling in-the-wild data, all within a simple architectural framework.