WildGS-SLAM: Monocular Gaussian Splatting SLAM in Dynamic Environments
Jianhao Zheng, Zihan Zhu, Valentin Bieri, Marc Pollefeys, Songyou Peng, Iro Armeni
2025-04-10
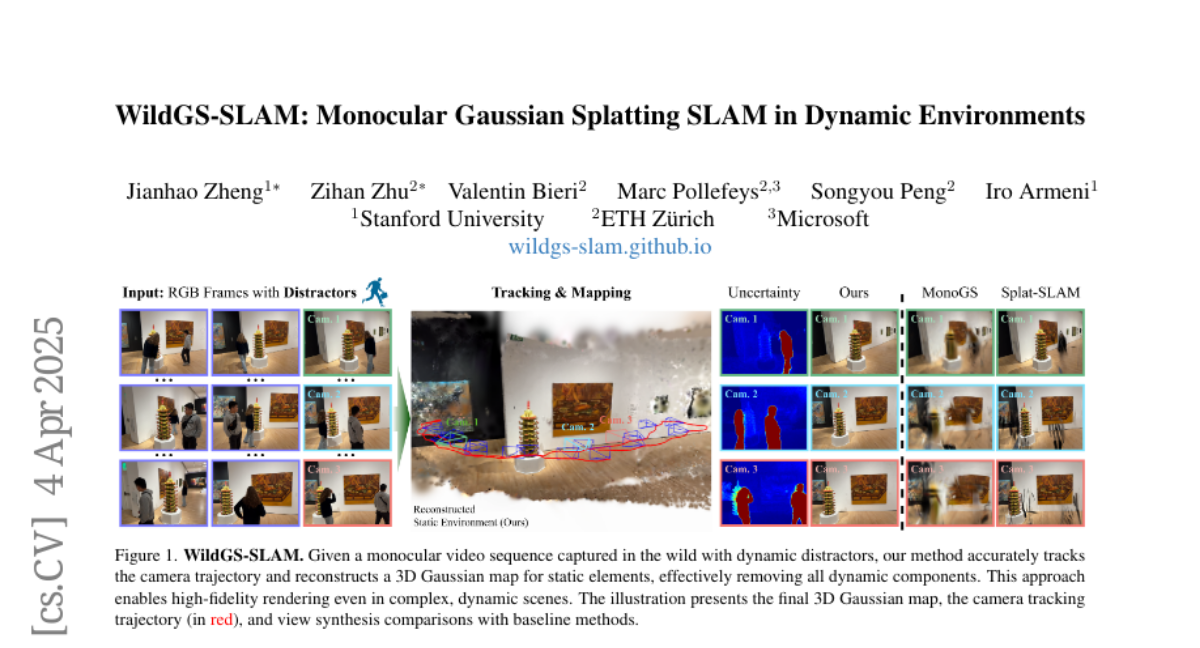
Summary
This paper talks about WildGS-SLAM, a smart camera system that can map messy, real-world scenes with moving objects by using AI to guess which parts of the scene are unreliable and ignore them.
What's the problem?
Normal camera mapping systems get confused by moving objects like people or cars, leading to blurry maps or wrong location tracking.
What's the solution?
WildGS-SLAM uses an AI-generated ‘uncertainty map’ to spot and skip moving objects, then builds a clean 3D map using only reliable parts of the scene.
Why it matters?
This helps robots, drones, or AR/VR systems work better in crowded places like cities or malls, where things move constantly, making navigation safer and more accurate.
Abstract
We present WildGS-SLAM, a robust and efficient monocular RGB SLAM system designed to handle dynamic environments by leveraging uncertainty-aware geometric mapping. Unlike traditional SLAM systems, which assume static scenes, our approach integrates depth and uncertainty information to enhance tracking, mapping, and rendering performance in the presence of moving objects. We introduce an uncertainty map, predicted by a shallow multi-layer perceptron and DINOv2 features, to guide dynamic object removal during both tracking and mapping. This uncertainty map enhances dense bundle adjustment and Gaussian map optimization, improving reconstruction accuracy. Our system is evaluated on multiple datasets and demonstrates artifact-free view synthesis. Results showcase WildGS-SLAM's superior performance in dynamic environments compared to state-of-the-art methods.