WorldCuisines: A Massive-Scale Benchmark for Multilingual and Multicultural Visual Question Answering on Global Cuisines
Genta Indra Winata, Frederikus Hudi, Patrick Amadeus Irawan, David Anugraha, Rifki Afina Putri, Yutong Wang, Adam Nohejl, Ubaidillah Ariq Prathama, Nedjma Ousidhoum, Afifa Amriani, Anar Rzayev, Anirban Das, Ashmari Pramodya, Aulia Adila, Bryan Wilie, Candy Olivia Mawalim, Ching Lam Cheng, Daud Abolade, Emmanuele Chersoni, Enrico Santus, Fariz Ikhwantri, Garry Kuwanto
2024-10-18
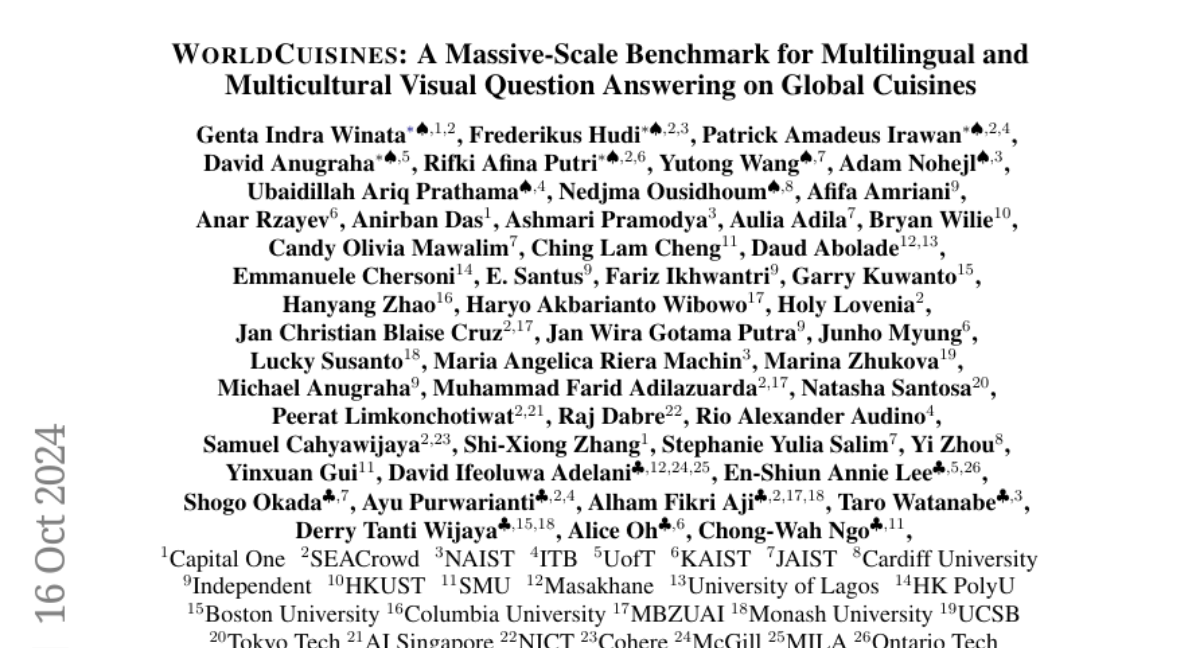
Summary
This paper introduces WorldCuisines, a large-scale benchmark designed to test how well AI models understand and answer questions about global cuisines in multiple languages and cultures.
What's the problem?
Many AI models struggle with understanding cultural knowledge, especially when it comes to cuisines from different countries and in languages other than English. Existing benchmarks often focus on a narrow range of cultures and languages, making it hard for these models to perform well in diverse real-world situations.
What's the solution?
To address this issue, the authors created WorldCuisines, which includes over 1 million images of food dishes from 196 countries, along with questions and answers in 38 different languages. This benchmark allows AI models to be tested on their ability to recognize dishes, understand their origins, and answer questions related to various cuisines. The dataset is designed to challenge models by requiring them to not only identify images but also apply cultural knowledge.
Why it matters?
This research is important because it helps improve AI's ability to understand and interact with diverse cultures. By creating a benchmark that reflects the complexity of global cuisines, WorldCuisines can lead to better AI applications in areas like recipe recommendations, culinary education, and international food services. It encourages the development of more inclusive AI systems that can engage with a wide range of cultural contexts.
Abstract
Vision Language Models (VLMs) often struggle with culture-specific knowledge, particularly in languages other than English and in underrepresented cultural contexts. To evaluate their understanding of such knowledge, we introduce WorldCuisines, a massive-scale benchmark for multilingual and multicultural, visually grounded language understanding. This benchmark includes a visual question answering (VQA) dataset with text-image pairs across 30 languages and dialects, spanning 9 language families and featuring over 1 million data points, making it the largest multicultural VQA benchmark to date. It includes tasks for identifying dish names and their origins. We provide evaluation datasets in two sizes (12k and 60k instances) alongside a training dataset (1 million instances). Our findings show that while VLMs perform better with correct location context, they struggle with adversarial contexts and predicting specific regional cuisines and languages. To support future research, we release a knowledge base with annotated food entries and images along with the VQA data.