WPO: Enhancing RLHF with Weighted Preference Optimization
Wenxuan Zhou, Ravi Agrawal, Shujian Zhang, Sathish Reddy Indurthi, Sanqiang Zhao, Kaiqiang Song, Silei Xu, Chenguang Zhu
2024-06-18
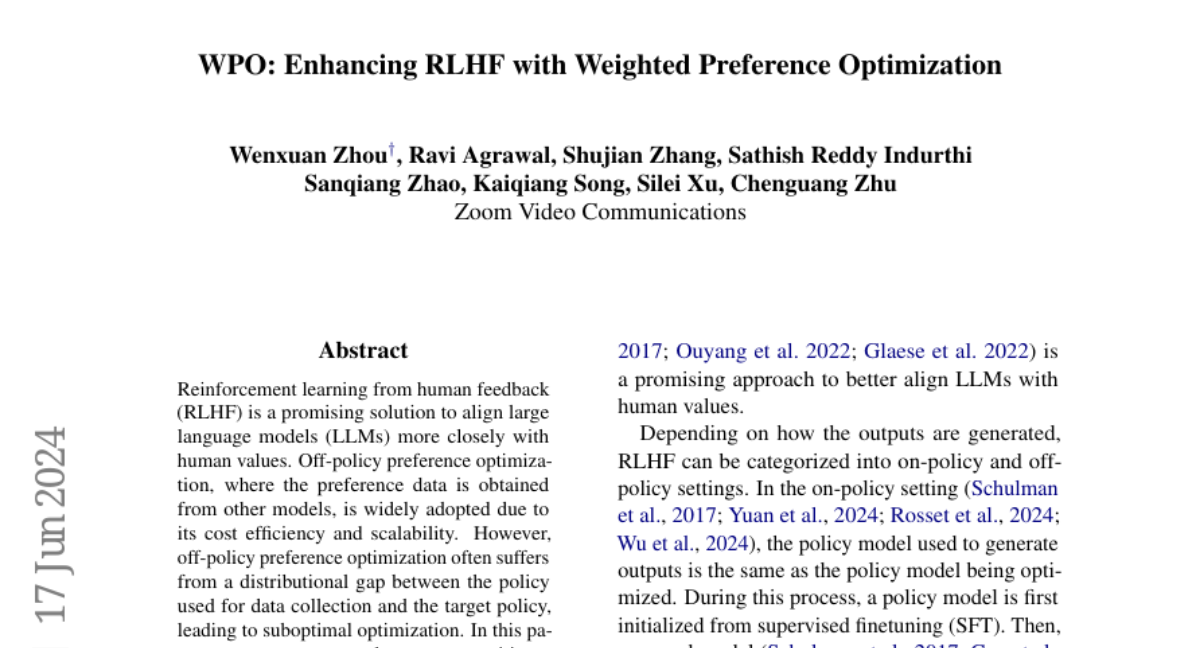
Summary
This paper introduces a new method called Weighted Preference Optimization (WPO) designed to improve how large language models (LLMs) learn from human feedback. It focuses on making the training process more effective by better using data collected from previous models.
What's the problem?
Reinforcement Learning from Human Feedback (RLHF) is a technique used to train AI models by incorporating feedback from people. However, when using data from other models (called off-policy data), there can be a mismatch between how the data was collected and how the model should learn from it. This mismatch can lead to less effective training and poorer performance in understanding human preferences.
What's the solution?
To solve this problem, the authors developed WPO, which adjusts off-policy data to make it more similar to on-policy data (data collected directly from the model being trained). They do this by reweighting preference pairs based on their likelihood under the current model. This approach helps bridge the gap between the two types of data, improving the training process without requiring extra costs. The authors tested WPO on various benchmarks and found that it outperformed previous methods, achieving better results in instruction-following tasks.
Why it matters?
This research is significant because it enhances how AI systems align with human values and preferences. By improving the way these models learn from feedback, WPO can lead to more accurate and reliable AI applications, making them more useful in real-world situations like customer service or personal assistants. This advancement could ultimately help create AI that better understands and responds to human needs.
Abstract
Reinforcement learning from human feedback (RLHF) is a promising solution to align large language models (LLMs) more closely with human values. Off-policy preference optimization, where the preference data is obtained from other models, is widely adopted due to its cost efficiency and scalability. However, off-policy preference optimization often suffers from a distributional gap between the policy used for data collection and the target policy, leading to suboptimal optimization. In this paper, we propose a novel strategy to mitigate this problem by simulating on-policy learning with off-policy preference data. Our Weighted Preference Optimization (WPO) method adapts off-policy data to resemble on-policy data more closely by reweighting preference pairs according to their probability under the current policy. This method not only addresses the distributional gap problem but also enhances the optimization process without incurring additional costs. We validate our method on instruction following benchmarks including Alpaca Eval 2 and MT-bench. WPO not only outperforms Direct Preference Optimization (DPO) by up to 5.6% on Alpaca Eval 2 but also establishes a remarkable length-controlled winning rate against GPT-4-turbo of 48.6% based on Llama-3-8B-Instruct, making it the strongest 8B model on the leaderboard. We will release the code and models at https://github.com/wzhouad/WPO.