Writing in the Margins: Better Inference Pattern for Long Context Retrieval
Melisa Russak, Umar Jamil, Christopher Bryant, Kiran Kamble, Axel Magnuson, Mateusz Russak, Waseem AlShikh
2024-08-28
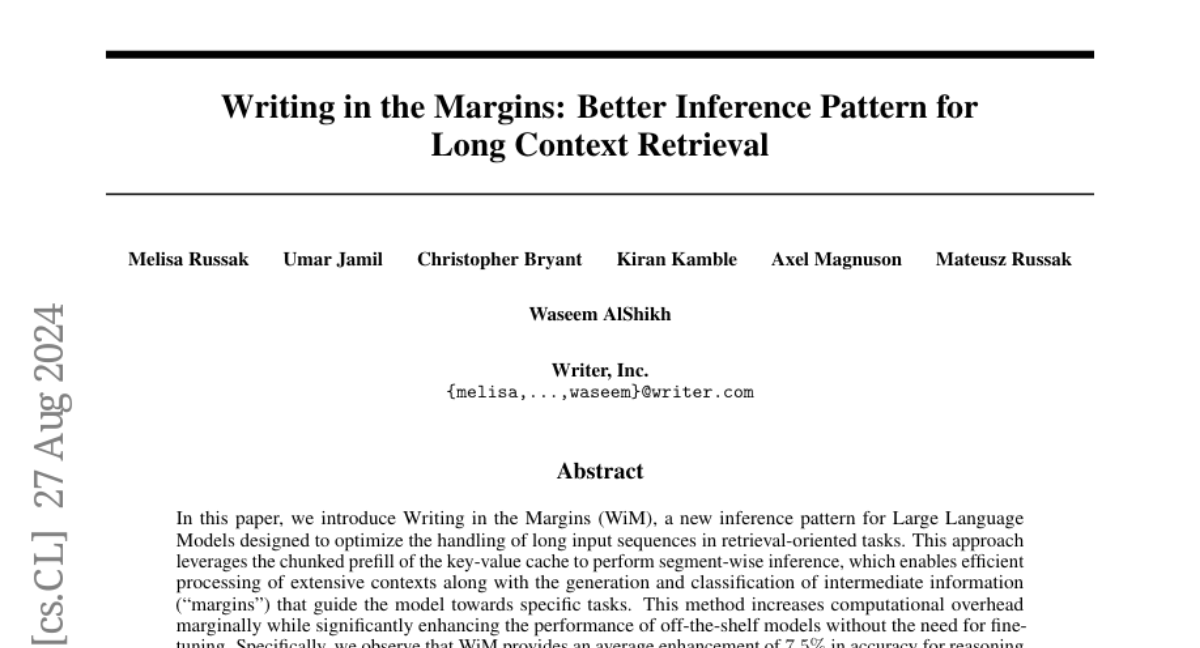
Summary
This paper introduces Writing in the Margins (WiM), a new method for improving how large language models handle long inputs when retrieving information.
What's the problem?
Large language models can struggle with long pieces of text because they often can't process everything at once. This makes it hard for them to provide accurate responses, especially when they need to remember details from earlier in the text. Existing methods either take too long or don't work well enough with complex tasks.
What's the solution?
WiM optimizes the way these models work by breaking down long inputs into smaller parts and processing them in segments. It uses a technique called chunked prefill to manage information better and generates 'margins' or intermediate information that helps guide the model's responses. This approach improves the accuracy of the model without needing extra training, resulting in better performance on various tasks.
Why it matters?
This research is important because it enhances how AI systems understand and respond to long texts, making them more effective for real-world applications like answering questions or summarizing information. By improving these models, we can create smarter tools for education, customer service, and many other fields.
Abstract
In this paper, we introduce Writing in the Margins (WiM), a new inference pattern for Large Language Models designed to optimize the handling of long input sequences in retrieval-oriented tasks. This approach leverages the chunked prefill of the key-value cache to perform segment-wise inference, which enables efficient processing of extensive contexts along with the generation and classification of intermediate information ("margins") that guide the model towards specific tasks. This method increases computational overhead marginally while significantly enhancing the performance of off-the-shelf models without the need for fine-tuning. Specifically, we observe that WiM provides an average enhancement of 7.5% in accuracy for reasoning skills (HotpotQA, MultiHop-RAG) and more than a 30.0% increase in the F1-score for aggregation tasks (CWE). Additionally, we show how the proposed pattern fits into an interactive retrieval design that provides end-users with ongoing updates about the progress of context processing, and pinpoints the integration of relevant information into the final response. We release our implementation of WiM using Hugging Face Transformers library at https://github.com/writer/writing-in-the-margins.