xGen-VideoSyn-1: High-fidelity Text-to-Video Synthesis with Compressed Representations
Can Qin, Congying Xia, Krithika Ramakrishnan, Michael Ryoo, Lifu Tu, Yihao Feng, Manli Shu, Honglu Zhou, Anas Awadalla, Jun Wang, Senthil Purushwalkam, Le Xue, Yingbo Zhou, Huan Wang, Silvio Savarese, Juan Carlos Niebles, Zeyuan Chen, Ran Xu, Caiming Xiong
2024-08-23
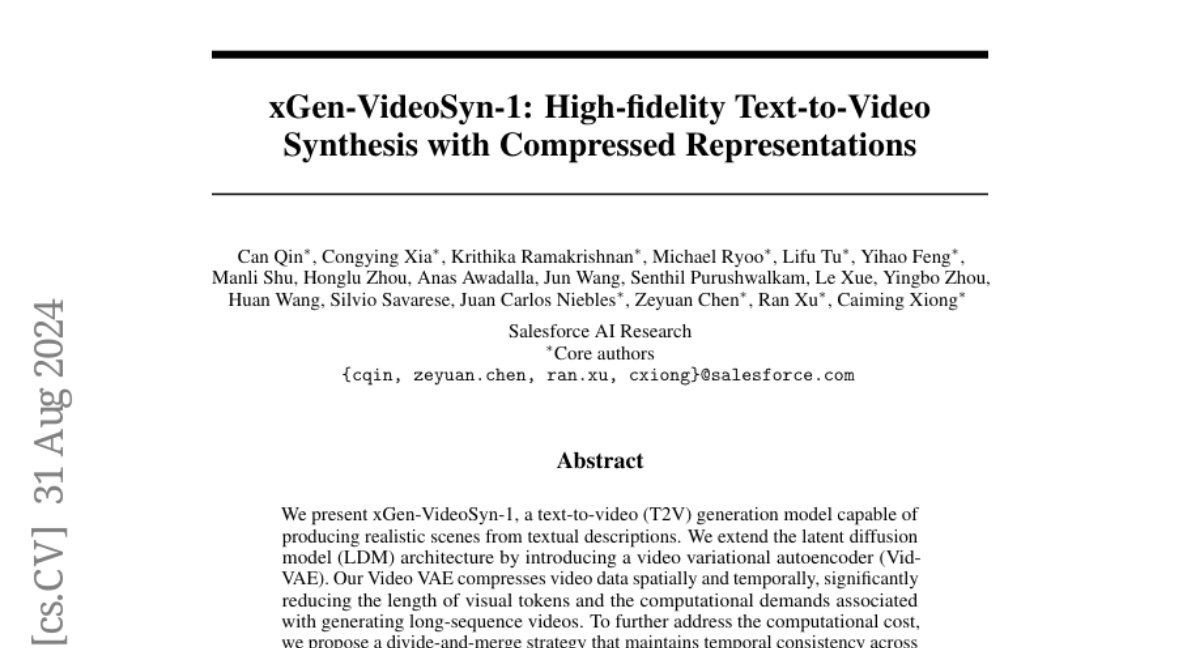
Summary
This paper introduces xGen-VideoSyn-1, a model that creates high-quality videos from text descriptions, making it easier to generate realistic video content.
What's the problem?
Generating videos from text is challenging because it requires a lot of computational power and efficient data processing. Traditional methods can struggle with long videos and mixed types of data, leading to slow performance and lower quality.
What's the solution?
The authors developed xGen-VideoSyn-1, which uses a new architecture called a latent diffusion model (LDM) and a video variational autoencoder (VidVAE) to compress video data. This helps reduce the amount of information needed to create videos while maintaining quality. They also introduced a divide-and-merge strategy to keep video segments consistent over time. The model can generate videos that are over 14 seconds long at 720p resolution and performs well on various benchmarks compared to other models.
Why it matters?
This research is significant because it advances the technology for creating videos from text, which has applications in entertainment, education, and content creation. By improving the efficiency and quality of video generation, it opens up new possibilities for how we can use AI in multimedia projects.
Abstract
We present xGen-VideoSyn-1, a text-to-video (T2V) generation model capable of producing realistic scenes from textual descriptions. Building on recent advancements, such as OpenAI's Sora, we explore the latent diffusion model (LDM) architecture and introduce a video variational autoencoder (VidVAE). VidVAE compresses video data both spatially and temporally, significantly reducing the length of visual tokens and the computational demands associated with generating long-sequence videos. To further address the computational costs, we propose a divide-and-merge strategy that maintains temporal consistency across video segments. Our Diffusion Transformer (DiT) model incorporates spatial and temporal self-attention layers, enabling robust generalization across different timeframes and aspect ratios. We have devised a data processing pipeline from the very beginning and collected over 13M high-quality video-text pairs. The pipeline includes multiple steps such as clipping, text detection, motion estimation, aesthetics scoring, and dense captioning based on our in-house video-LLM model. Training the VidVAE and DiT models required approximately 40 and 642 H100 days, respectively. Our model supports over 14-second 720p video generation in an end-to-end way and demonstrates competitive performance against state-of-the-art T2V models.