XLand-100B: A Large-Scale Multi-Task Dataset for In-Context Reinforcement Learning
Alexander Nikulin, Ilya Zisman, Alexey Zemtsov, Viacheslav Sinii, Vladislav Kurenkov, Sergey Kolesnikov
2024-06-17
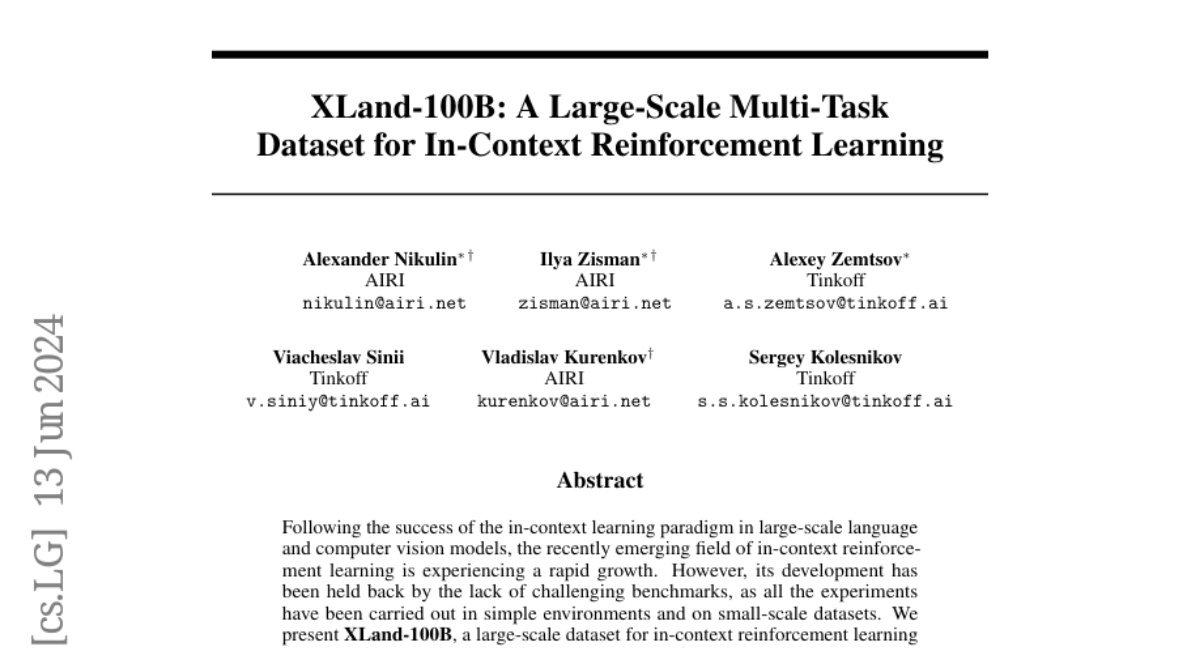
Summary
This paper introduces XLand-100B, a large-scale dataset designed for in-context reinforcement learning. It aims to provide a challenging set of tasks for AI models to learn from, helping them improve their performance in complex environments.
What's the problem?
The field of in-context reinforcement learning has been growing rapidly, but researchers have struggled because there aren't enough challenging benchmarks to test AI models effectively. Most existing experiments have been done in simple settings with small datasets, which limits the ability to evaluate how well these models can learn and adapt to new situations.
What's the solution?
To address this issue, the authors created XLand-100B, which includes detailed learning histories for nearly 30,000 different tasks. This dataset covers a massive 100 billion transitions and 2.5 billion episodes, providing a rich resource for training AI models. Collecting this dataset required an enormous amount of computational power—50,000 GPU hours—which is typically too much for smaller research teams. The authors also made tools available to help others reproduce or expand upon their work.
Why it matters?
This research is significant because it opens up new opportunities for studying and improving in-context reinforcement learning. By providing a comprehensive dataset, XLand-100B allows researchers to better understand how AI models can learn from complex tasks and environments. This could lead to advancements in AI applications across various fields, making them more effective and adaptable.
Abstract
Following the success of the in-context learning paradigm in large-scale language and computer vision models, the recently emerging field of in-context reinforcement learning is experiencing a rapid growth. However, its development has been held back by the lack of challenging benchmarks, as all the experiments have been carried out in simple environments and on small-scale datasets. We present XLand-100B, a large-scale dataset for in-context reinforcement learning based on the XLand-MiniGrid environment, as a first step to alleviate this problem. It contains complete learning histories for nearly 30,000 different tasks, covering 100B transitions and 2.5B episodes. It took 50,000 GPU hours to collect the dataset, which is beyond the reach of most academic labs. Along with the dataset, we provide the utilities to reproduce or expand it even further. With this substantial effort, we aim to democratize research in the rapidly growing field of in-context reinforcement learning and provide a solid foundation for further scaling. The code is open-source and available under Apache 2.0 licence at https://github.com/dunno-lab/xland-minigrid-datasets.